Iterative Multiview Subspace Learning for Unpaired Multiview Clustering.
IEEE transactions on neural networks and learning systems(2023)
摘要
In real applications, several unpredictable or uncertain factors could result in unpaired multiview data, i.e., the observed samples between views cannot be matched. Since joint clustering among views is more effective than individual clustering in each view, we investigate unpaired multiview clustering (UMC), which is a valuable but insufficiently studied problem. Due to lack of matched samples between views, we could fail to build the connection between views. Therefore, we aim to learn the latent subspace shared by views. However, existing multiview subspace learning methods usually rely on the matched samples between views. To address this issue, we propose an iterative multiview subspace learning strategy iterative unpaired multiview clustering (IUMC), aiming to learn a complete and consistent subspace representation among views for UMC. Moreover, based on IUMC, we design two effective UMC methods: 1) Iterative unpaired multiview clustering via covariance matrix alignment (IUMC-CA) that further aligns the covariance matrix of subspace representations and then performs clustering on the subspace and 2) iterative unpaired multiview clustering via one-stage clustering assignments (IUMC-CY) that performs one-stage multiview clustering (MVC) by replacing the subspace representations with clustering assignments. Extensive experiments show the excellent performance of our methods for UMC, compared with the state-of-the-art methods. Also, the clustering performance of observed samples in each view can be considerably improved by those observed samples from the other views. In addition, our methods have good applicability in incomplete MVC.
更多查看译文
关键词
Covariance matrix alignment,iterative multiview subspace learning,unpaired multiview clustering (UMC)
AI 理解论文
溯源树
样例
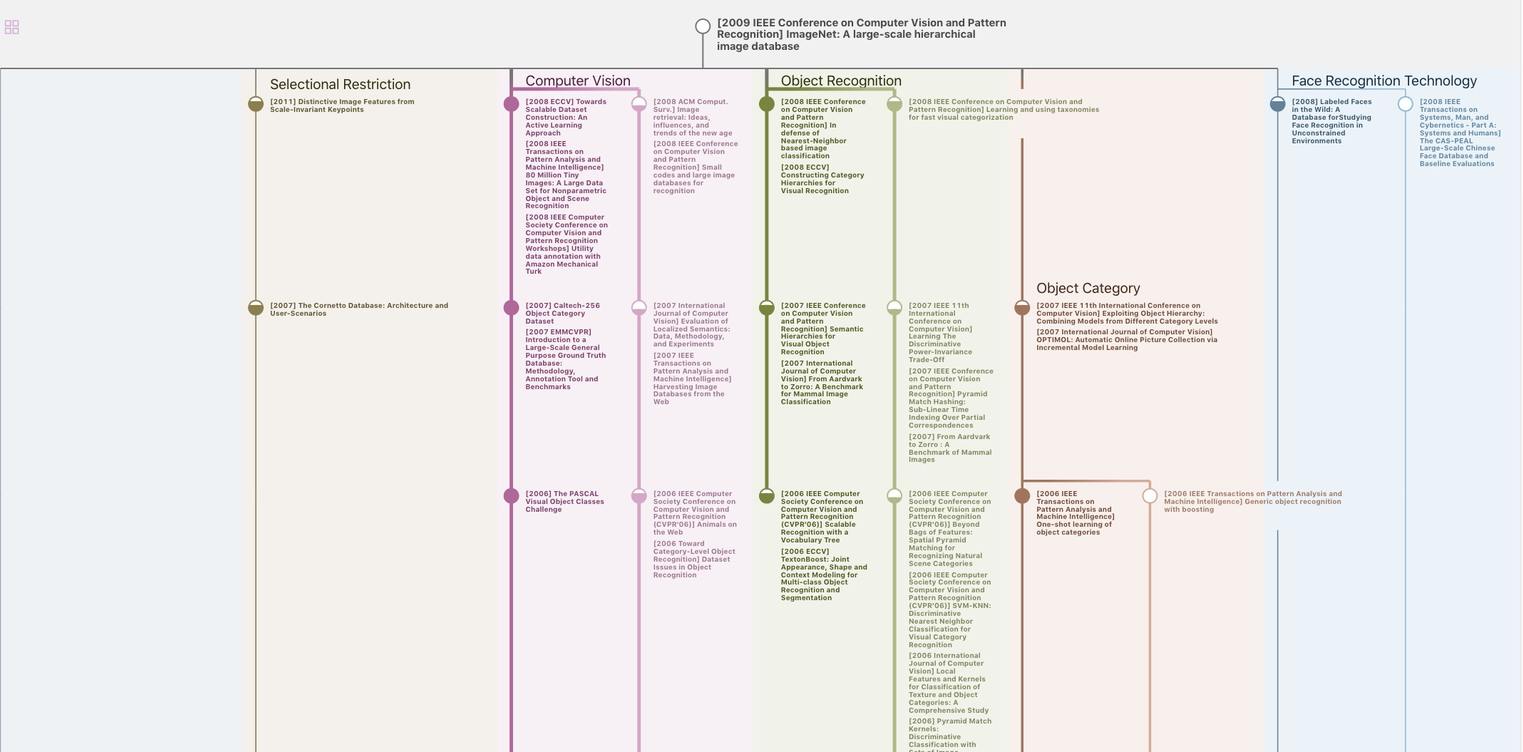
生成溯源树,研究论文发展脉络
Chat Paper
正在生成论文摘要