Large-Scale Modeling of Sparse Protein Kinase Activity Data
Journal of chemical information and modeling(2023)
摘要
Protein kinases are a protein family that plays an importantrolein several complex diseases such as cancer and cardiovascular andimmunological diseases. Protein kinases have conserved ATP bindingsites, which when targeted can lead to similar activities of inhibitorsagainst different kinases. This can be exploited to create multitargetdrugs. On the other hand, selectivity (lack of similar activities)is desirable in order to avoid toxicity issues. There is a vast amountof protein kinase activity data in the public domain, which can beused in many different ways. Multitask machine learning models areexpected to excel for these kinds of data sets because they can learnfrom implicit correlations between tasks (in this case activitiesagainst a variety of kinases). However, multitask modeling of sparsedata poses two major challenges: (i) creating a balanced train-testsplit without data leakage and (ii) handling missing data. In thiswork, we construct a protein kinase benchmark set composed of twobalanced splits without data leakage, using random and dissimilarity-drivencluster-based mechanisms, respectively. This data set can be usedfor benchmarking and developing protein kinase activity predictionmodels. Overall, the performance on the dissimilarity-driven cluster-basedsplit is lower than on random split-based sets for all models, indicatingpoor generalizability of models. Nevertheless, we show that multitaskdeep learning models, on this very sparse data set, outperform single-taskdeep learning and tree-based models. Finally, we demonstrate thatdata imputation does not improve the performance of (multitask) modelson this benchmark set.
更多查看译文
关键词
kinase,protein,modeling,large-scale
AI 理解论文
溯源树
样例
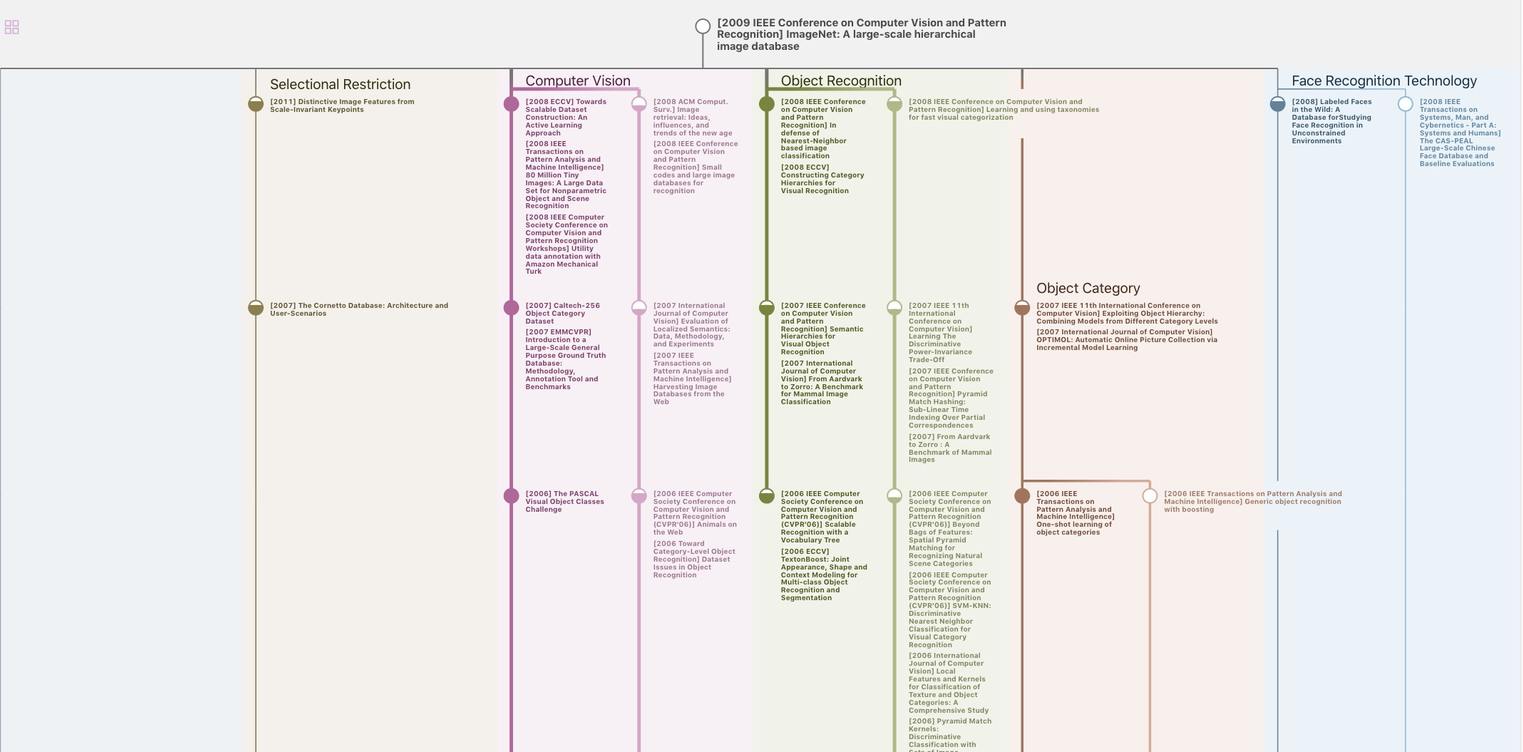
生成溯源树,研究论文发展脉络
Chat Paper
正在生成论文摘要