A population-level computational histologic signature for invasive breast cancer prognosis.
Research square(2023)
摘要
Breast cancer is a heterogeneous disease with variable survival outcomes. Pathologists grade the microscopic appearance of breast tissue using the Nottingham criteria, which is qualitative and does not account for non-cancerous elements within the tumor microenvironment (TME). We present the Histomic Prognostic Signature (HiPS), a comprehensive, interpretable scoring of the survival risk incurred by breast TME morphology. HiPS uses deep learning to accurately map cellular and tissue structures in order to measure epithelial, stromal, immune, and spatial interaction features. It was developed using a population-level cohort from the Cancer Prevention Study (CPS)-II and validated using data from three independent cohorts, including the PLCO trial, CPS-3, and The Cancer Genome Atlas. HiPS consistently outperformed pathologists' performance in predicting survival outcomes, independent of TNM stage and pertinent variables. This was largely driven by stromal and immune features. In conclusion, HiPS is a robustly validated biomarker to support pathologists and improve prognosis.
更多查看译文
关键词
computational histologic signature,invasive breast cancer prognosis,breast cancer,population-level
AI 理解论文
溯源树
样例
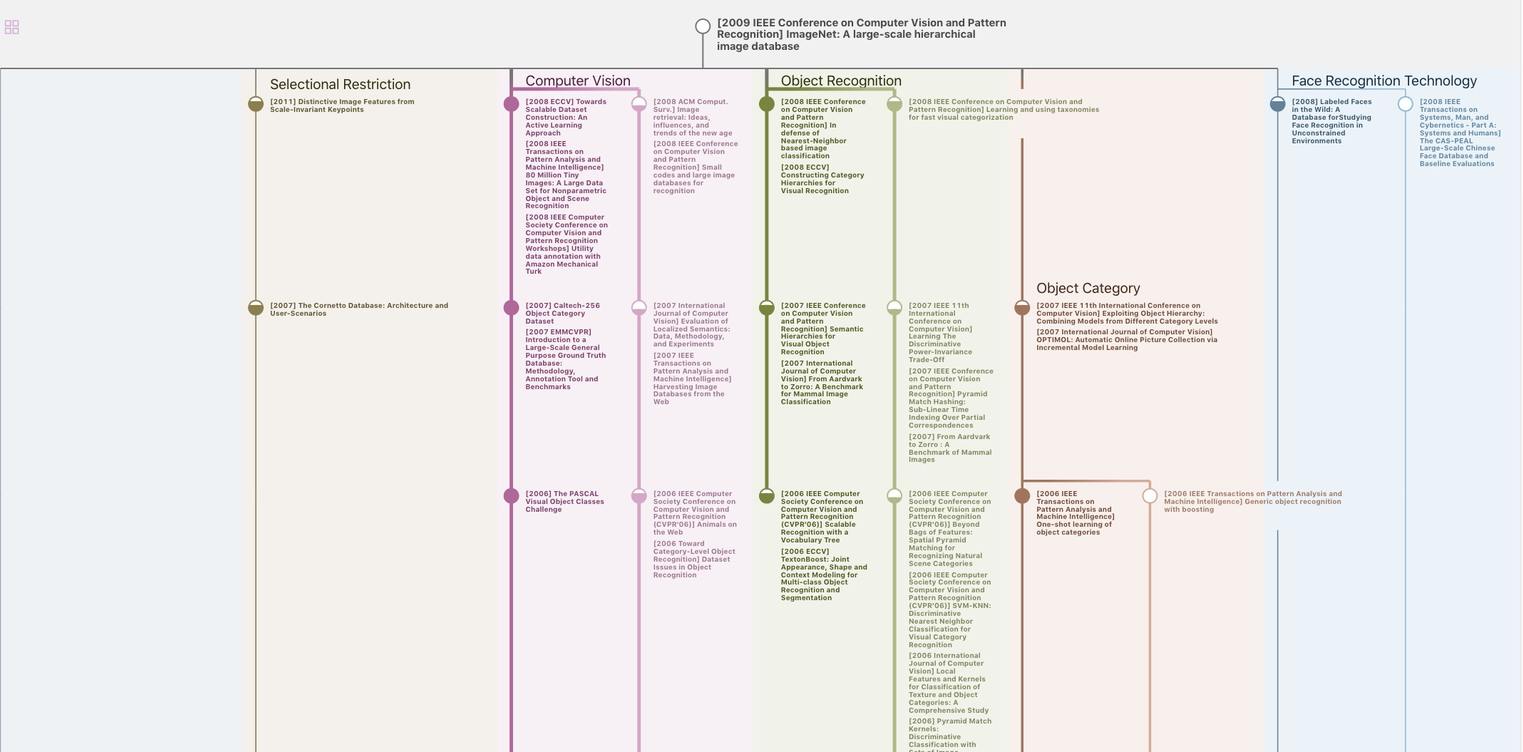
生成溯源树,研究论文发展脉络
Chat Paper
正在生成论文摘要