Automatic Lumen and Anatomical Layers Segmentation in IVOCT Images Using Meta Learning.
Journal of biophotonics(2023)
摘要
Automated analysis of the vessel structure in intravascular optical coherence tomography (IVOCT) images is critical to assess the health status of vessels and monitor coronary artery disease progression. However, deep learning-based methods usually require well-annotated large datasets, which are difficult to obtain in the field of medical image analysis. Hence, an automatic layers segmentation method based on Meta-learning was proposed, which can simultaneously extract the surfaces of the lumen, intima, media, and adventitia using a handful of annotated samples. Specifically, we leverage a bi-level gradient strategy to train a meta-learner for capturing the shared meta-knowledge among different anatomical layers and quickly adapting to unknown anatomical layers. Then, a Claw-type network and a contrast consistency loss were designed to better learn the meta-knowledge according to the characteristic of annotation of the lumen and anatomical layers. Experimental results on the two cardiovascular IVOCT datasets show that the proposed method achieved state-of-art performance. This article is protected by copyright. All rights reserved.
更多查看译文
关键词
anatomical layers segmentation,automatic lumen,images,learning
AI 理解论文
溯源树
样例
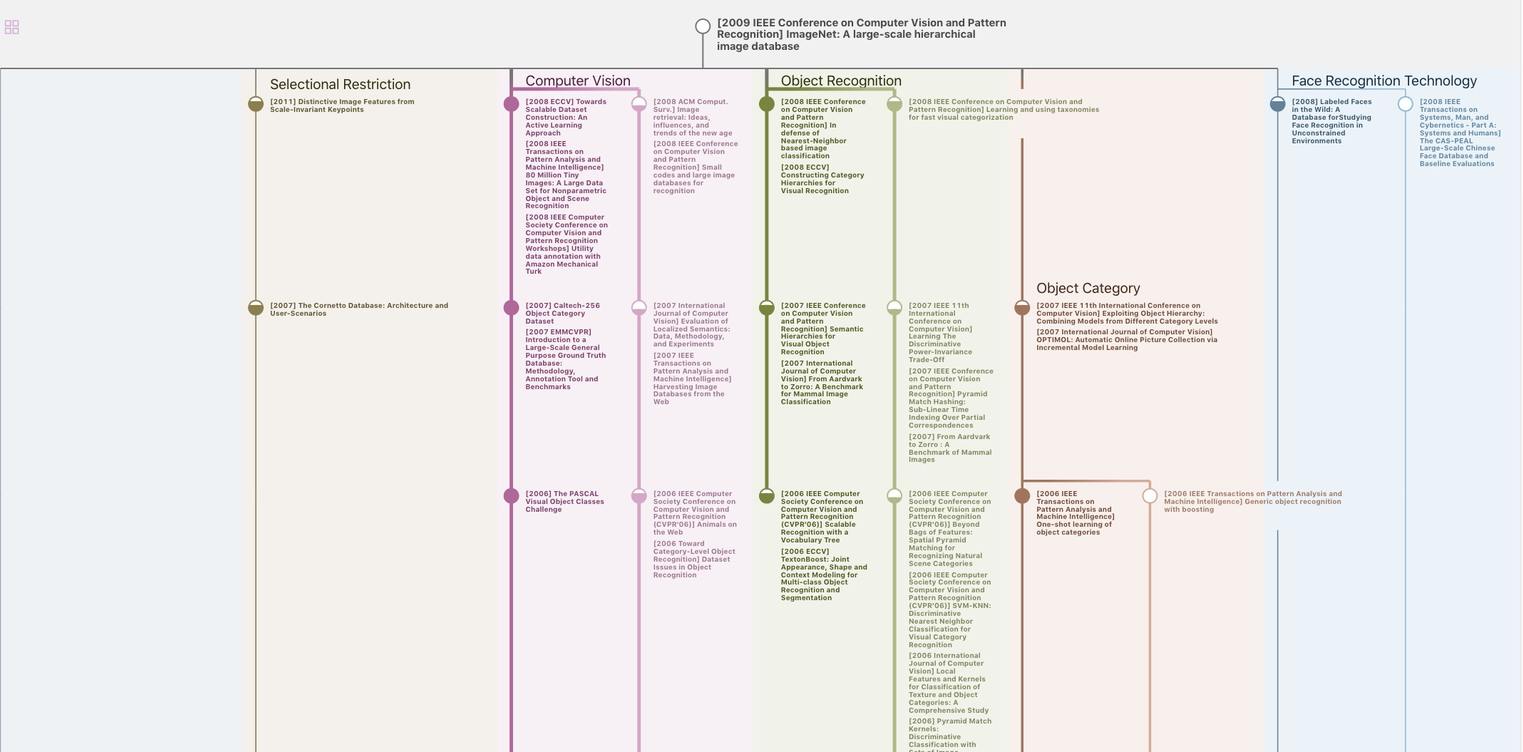
生成溯源树,研究论文发展脉络
Chat Paper
正在生成论文摘要