Transvaginal ultrasonography predictive model for the detection of pelvic congestion syndrome
Quantitative imaging in medicine and surgery(2023)
摘要
Background: The diagnosis of pelvic congestion syndrome (PCS) remains a challenge given the lack of universally accepted criteria. Although venography (VG) is the current gold standard for the diagnosis of PCS, non-invasive techniques like transvaginal ultrasonography (TVU) appear to be a valid alternative. The aim of this study was to design a predictive model for the venographic diagnostic of PCS using the parameters identified by TVU in patients with clinical suspicion of PCS, in order to individually assess the need to perform an invasive diagnostic and therapeutic technique such as VG. Methods: An observational and cross-sectional prospective study was conducted including 61 consecutively recruited patients with clinical suspicion of PCS, who were referred by the Pelvic Floor, Gynecology and Vascular Surgery Units, who were distributed in two groups: 18 belonging to the normal group and 43 to the PCS's group. We implemented and compared 19 binary logistic regression models, including the parameters that showed statistical significance in the prior univariate analysis. We evaluated individual predictive values with a receiver operating characteristic (ROC) curve and the area under the curve (AUC). Results: The selected model, based on the presence of pelvic veins or venous plexus of 8 mm or larger, observed by transvaginal ultrasound, had an AUC of 0.79 (95% CI: 0.63-0.96; P<0.001), with a sensitivity of 0.90 and specificity of 0.69, while the VG had a sensitivity of 86.05%, a specificity of 66.67%, and a positive predictive value of 86.05%. Conclusions: This assessment presents a feasible alternative that could potentially be added to our usual gynecological practice.
更多查看译文
关键词
Pelvic congestion syndrome (PCS),chronic pelvic pain (CPP),transvaginal ultrasonography (TVU),venography (VG),predictive model
AI 理解论文
溯源树
样例
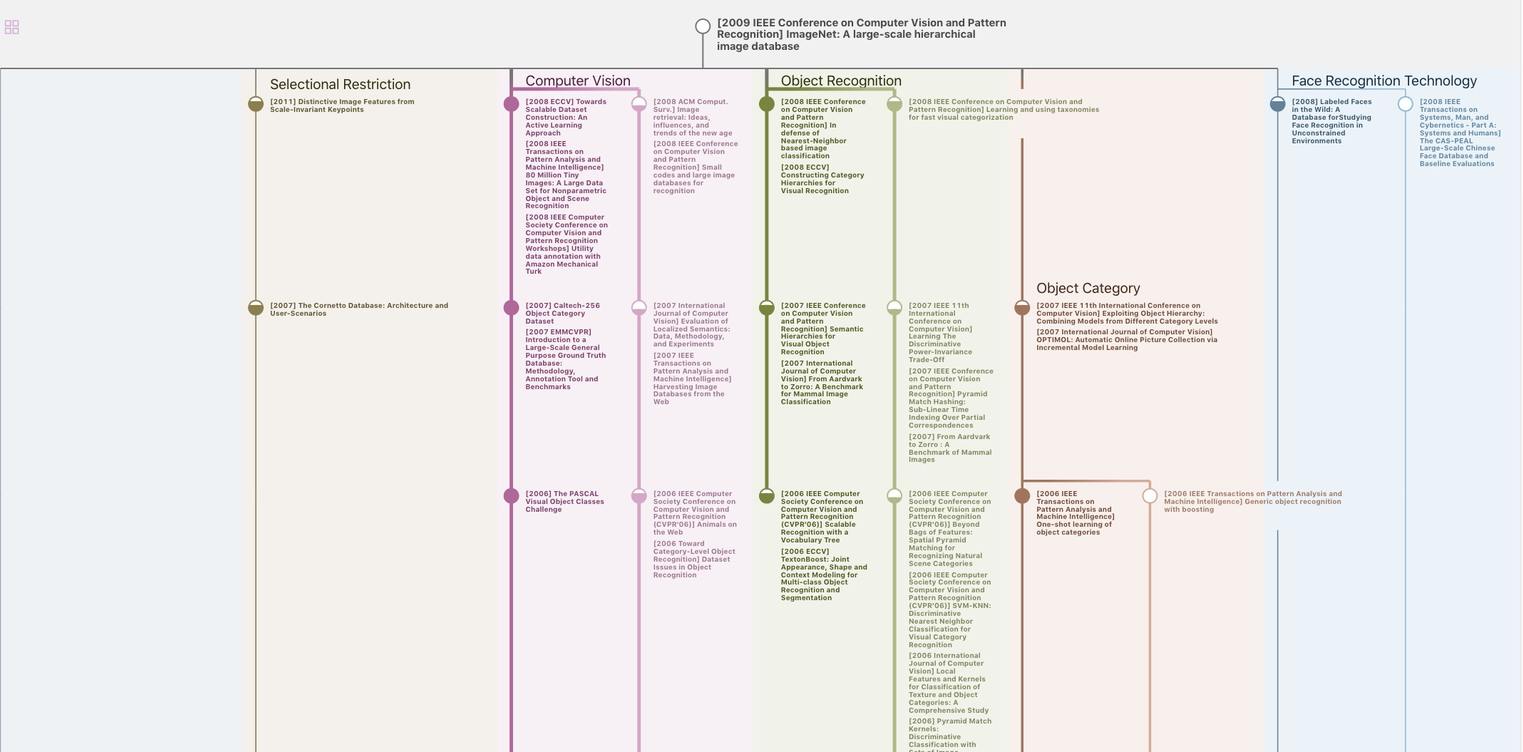
生成溯源树,研究论文发展脉络
Chat Paper
正在生成论文摘要