Massively parallel CRISPR off-target detection enables rapid off-target prediction model building.
Med (New York, N.Y.)(2023)
摘要
BACKGROUND:CRISPR (clustered regularly interspaced short palindromic repeats) genome editing holds tremendous potential in clinical translation. However, the off-target effect has always been a major concern.
METHODS:Here, we have developed a novel sensitive and specific off-target detection method, AID-seq (adaptor-mediated off-target identification by sequencing), that can comprehensively and faithfully detect the low-frequency off targets generated by different CRISPR nucleases (including Cas9 and Cas12a).
FINDINGS:Based on AID-seq, we developed a pooled strategy to simultaneously identify the on/off targets of multiple gRNAs, as well as using mixed human and human papillomavirus (HPV) genomes to screen the most efficient and safe targets from 416 HPV gRNA candidates for antiviral therapy. Moreover, we used the pooled strategy with 2,069 single-guide RNAs (sgRNAs) at a pool size of about 500 to profile the properties of our newly discovered CRISPR, FrCas9. Importantly, we successfully built an off-target detection model using these off-target data via the CRISPR-Net deep learning method (area under the receiver operating characteristic curve [AUROC] = 0.97, area under the precision recall curve [AUPRC] = 0.29).
CONCLUSIONS:To our knowledge, AID-seq is the most sensitive and specific in vitro off-target detection method to date. And the pooled AID-seq strategy can be used as a rapid and high-throughput platform to select the best sgRNAs and characterize the properties of new CRISPRs.
FUNDING:This work was supported by The National Natural Science Foundation of China (grant nos. 32171465 and 82102392), the General Program of Natural Science Foundation of Guangdong Province of China (grant no. 2021A1515012438), Guangdong Basic and Applied Basic Research Foundation (grant no. 2020A1515110170), and the National Ten Thousand Plan-Young Top Talents of China (grant no. 80000-41180002).
更多查看译文
AI 理解论文
溯源树
样例
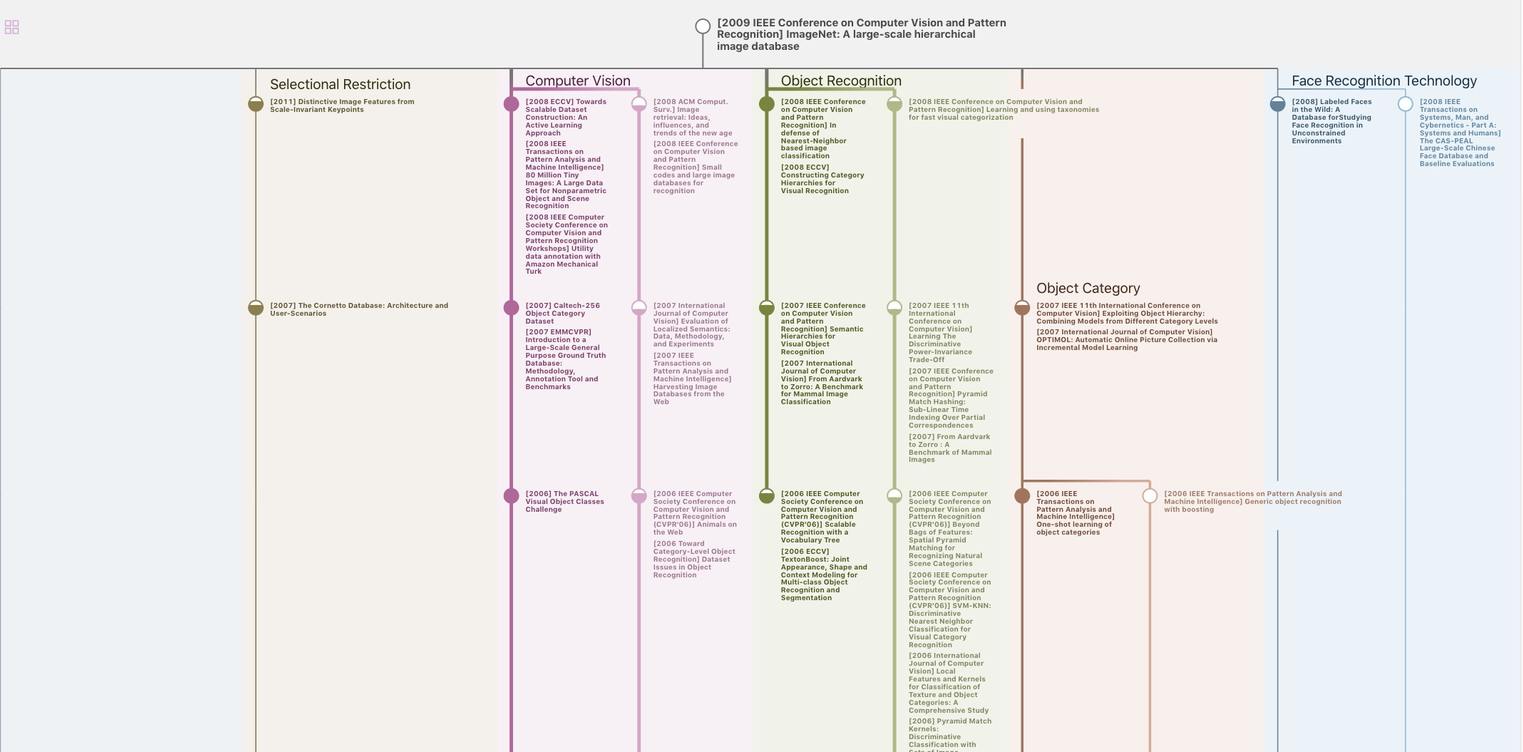
生成溯源树,研究论文发展脉络
Chat Paper
正在生成论文摘要