LARNeXt: End-to-End Lie Algebra Residual Network for Face Recognition.
IEEE transactions on pattern analysis and machine intelligence(2023)
摘要
Face recognition has always been courted in computer vision and is especially amenable to situations with significant variations between frontal and profile faces. Traditional techniques make great strides either by synthesizing frontal faces from sizable datasets or by empirical pose invariant learning. In this paper, we propose a completely integrated embedded end-to-end Lie algebra residual architecture (LARNeXt) to achieve pose robust face recognition. First, we explore how the face rotation in the 3D space affects the deep feature generation process of convolutional neural networks (CNNs), and prove that face rotation in the image space is equivalent to an additive residual component in the feature space of CNNs, which is determined solely by the rotation. Second, on the basis of this theoretical finding, we further design three critical subnets to leverage a soft regression subnet with novel multi-fusion attention feature aggregation for efficient pose estimation, a residual subnet for decoding rotation information from input face images, and a gating subnet to learn rotation magnitude for controlling the strength of the residual component that contributes to the feature learning process. Finally, we conduct a large number of ablation experiments, and our quantitative and visualization results both corroborate the credibility of our theory and corresponding network designs. Our comprehensive experimental evaluations on frontal-profile face datasets, general unconstrained face recognition datasets, and industrial-grade tasks demonstrate that our method consistently outperforms the state-of-the-art ones. Our code and model are made publicly available at https://github.com/paradocx/LARNet.
更多查看译文
关键词
face recognition,end-to-end
AI 理解论文
溯源树
样例
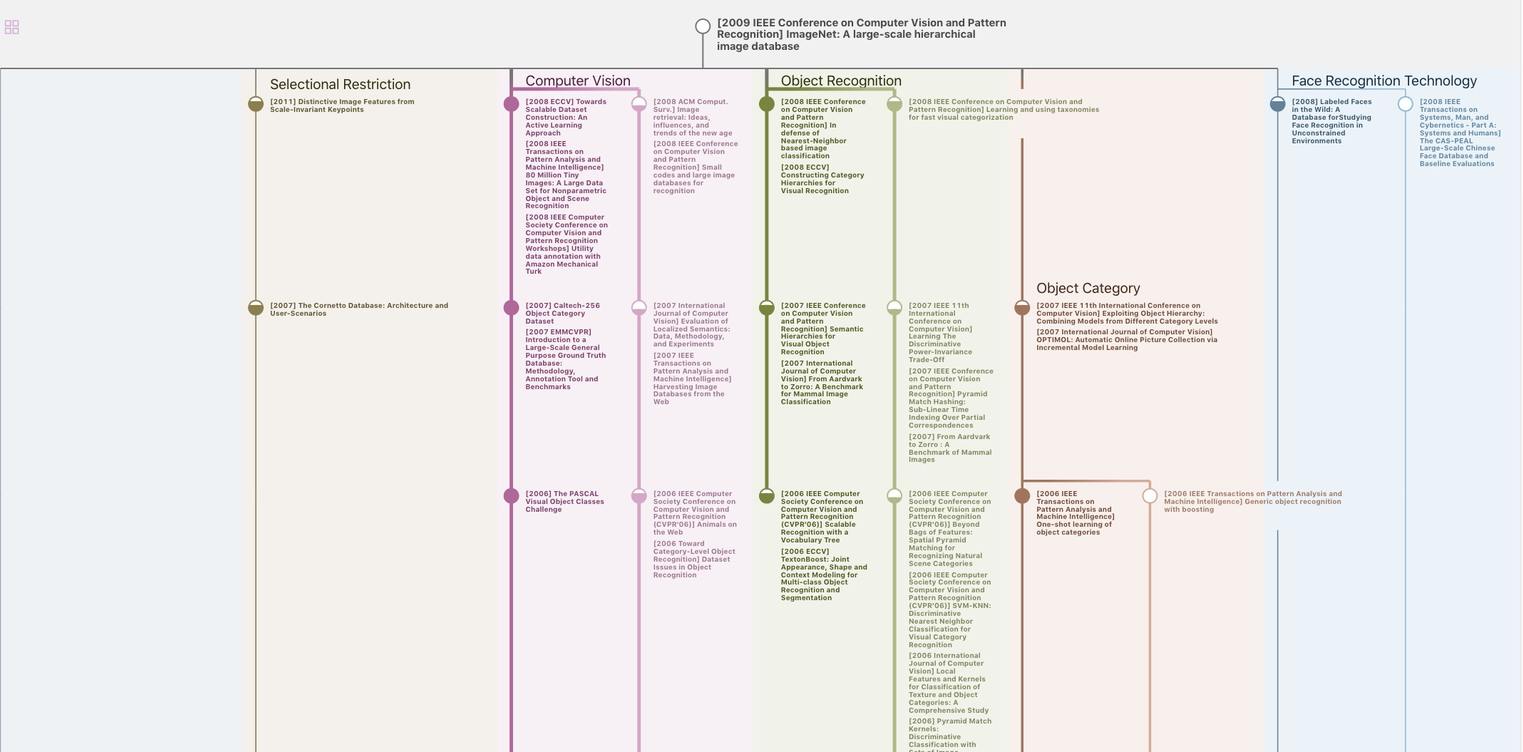
生成溯源树,研究论文发展脉络
Chat Paper
正在生成论文摘要