Development and validation of explainable machine learning models for risk of mortality in transcatheter aortic valve implantation: TAVI risk machine scores
EUROPEAN HEART JOURNAL - DIGITAL HEALTH(2023)
摘要
AimsIdentification of high-risk patients and individualized decision support based on objective criteria for rapid discharge after transcatheter aortic valve implantation (TAVI) are key requirements in the context of contemporary TAVI treatment. This study aimed to predict 30-day mortality following TAVI based on machine learning (ML) using data from the German Aortic Valve Registry.Methods and resultsMortality risk was determined using a random forest ML model that was condensed in the newly developed TAVI Risk Machine (TRIM) scores, designed to represent clinically meaningful risk modelling before (TRIMpre) and in particular after (TRIMpost) TAVI. Algorithm was trained and cross-validated on data of 22 283 patients (729 died within 30 days post-TAVI) and generalisation was examined on data of 5864 patients (146 died). TRIMpost demonstrated significantly better performance than traditional scores [C-statistics value, 0.79; 95% confidence interval (CI)] [0.74; 0.83] compared to Society of Thoracic Surgeons (STS) with C-statistics value 0.69; 95%-CI [0.65; 0.74]). An abridged (aTRIMpost) score comprising 25 features (calculated using a web interface) exhibited significantly higher performance than traditional scores (C-statistics value, 0.74; 95%-CI [0.70; 0.78]). Validation on external data of 6693 patients (205 died within 30 days post-TAVI) of the Swiss TAVI Registry confirmed significantly better performance for the TRIMpost (C-statistics value 0.75, 95%-CI [0.72; 0.79]) compared to STS (C-statistics value 0.67, CI [0.63; 0.70]).ConclusionTRIM scores demonstrate good performance for risk estimation before and after TAVI. Together with clinical judgement, they may support standardised and objective decision-making before and after TAVI. Graphical Abstract
更多查看译文
关键词
TAVI,machine learning,risk score,random forest,decision support
AI 理解论文
溯源树
样例
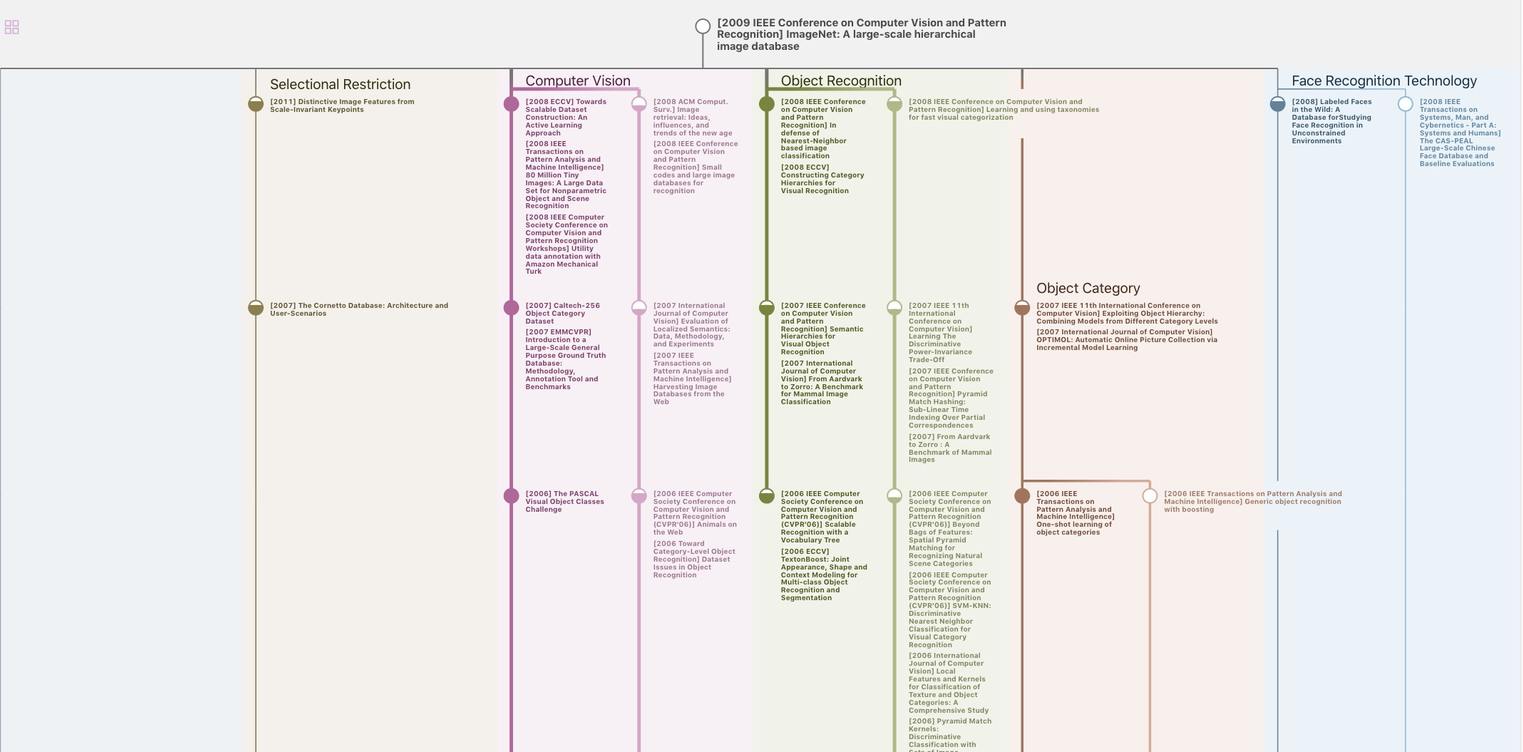
生成溯源树,研究论文发展脉络
Chat Paper
正在生成论文摘要