MCAS-GP: Deep Learning-Empowered Middle Cerebral Artery Segmentation and Gate Proposition.
IEEE/ACM transactions on computational biology and bioinformatics(2023)
摘要
With the fast development of AI technologies, deep learning is widely applied for biomedical data analytics and digital healthcare. However, there remain gaps between AI-aided diagnosis and real-world healthcare demands. For example, hemodynamic parameters of the middle cerebral artery (MCA) have significant clinical value for diagnosing adverse perinatal results. Nevertheless, the current measurement procedure is tedious for sonographers. To reduce the workload of sonographers, we propose MCAS-GP, a deep learning-empowered framework that tackles the Middle Cerebral Artery Segmentation and Gate Proposition. MCAS-GP can automatically segment the region of the MCA and detect the corresponding position of the gate in the procedure of fetal MCA Doppler assessment. In MCAS-GP, a novel learnable atrous spatial pyramid pooling (LASPP) module is designed to adaptively learn multi-scale features. We also propose a novel evaluation metric, Affiliation Index, for measuring the effectiveness of the position of the output gate. To evaluate our proposed MCAS-GP, we build a large-scale MCA dataset, collaborating with the International Peace Maternity and Child Health Hospital of China welfare institute (IPMCH). Extensive experiments on the MCA dataset and two other public surgical datasets demonstrate that MCAS-GP can achieve considerable performance improvement in both accuracy and inference time.
更多查看译文
关键词
Deep learning,keypoint detection,medical image segmentation,middle cerebral artery,ultrasound image
AI 理解论文
溯源树
样例
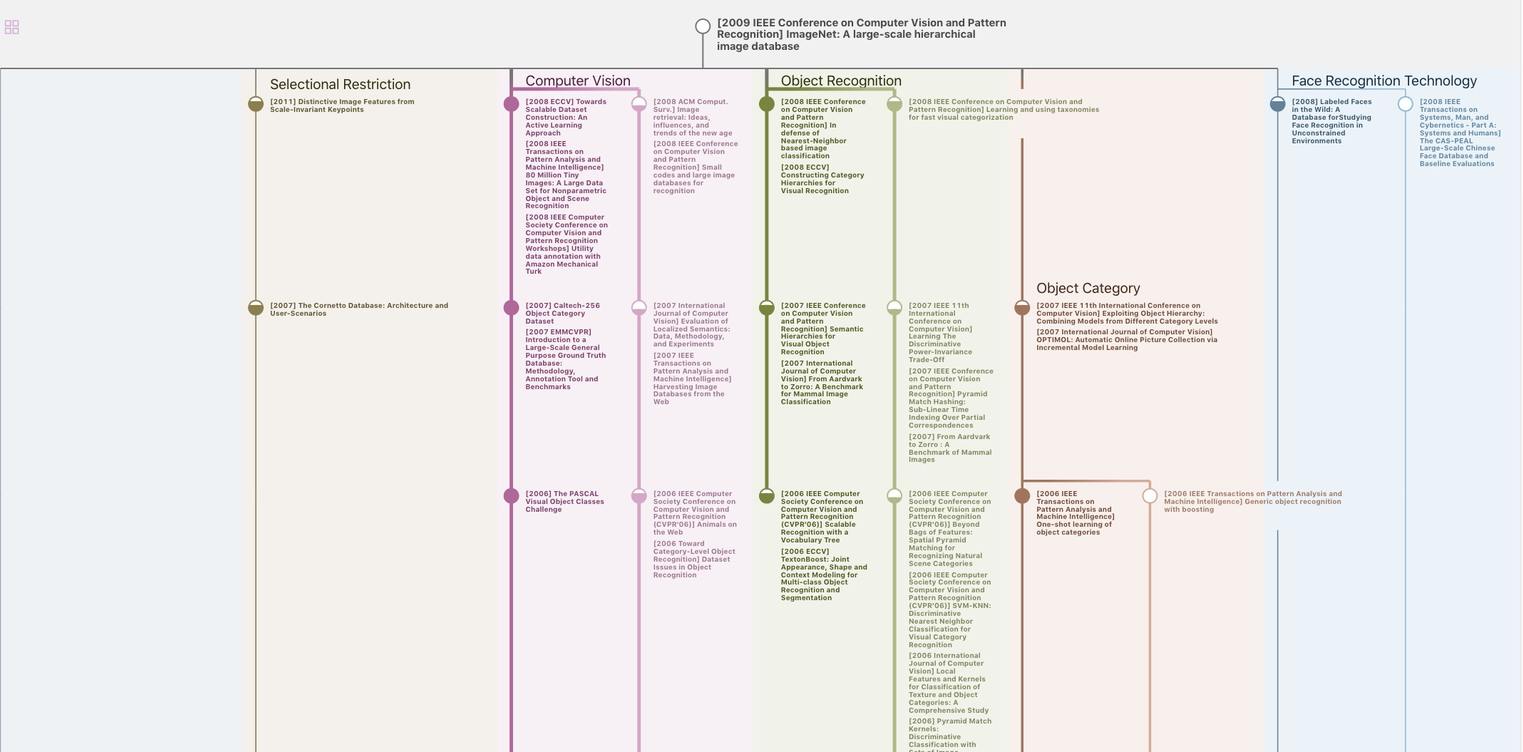
生成溯源树,研究论文发展脉络
Chat Paper
正在生成论文摘要