Voice analytics in the wild: Validity and predictive accuracy of common audio-recording devices
Behavior research methods(2023)
摘要
The use of voice recordings in both research and industry practice has increased dramatically in recent years—from diagnosing a COVID-19 infection based on patients’ self-recorded voice samples to predicting customer emotions during a service center call. Crowdsourced audio data collection in participants’ natural environment using their own recording device has opened up new avenues for researchers and practitioners to conduct research at scale across a broad range of disciplines. The current research examines whether fundamental properties of the human voice are reliably and validly captured through common consumer-grade audio-recording devices in current medical, behavioral science, business, and computer science research. Specifically, this work provides evidence from a tightly controlled laboratory experiment analyzing 1800 voice samples and subsequent simulations that recording devices with high proximity to a speaker (such as a headset or a lavalier microphone) lead to inflated measures of amplitude compared to a benchmark studio-quality microphone while recording devices with lower proximity to a speaker (such as a laptop or a smartphone in front of the speaker) systematically reduce measures of amplitude and can lead to biased measures of the speaker’s true fundamental frequency. We further demonstrate through simulation studies that these differences can lead to biased and ultimately invalid conclusions in, for example, an emotion detection task. Finally, we outline a set of recording guidelines to ensure reliable and valid voice recordings and offer initial evidence for a machine-learning approach to bias correction in the case of distorted speech signals.
更多查看译文
关键词
Audio-recording devices,Crowdsourcing,Audio data,Voice analytics,Amplitude,Fundamental frequency
AI 理解论文
溯源树
样例
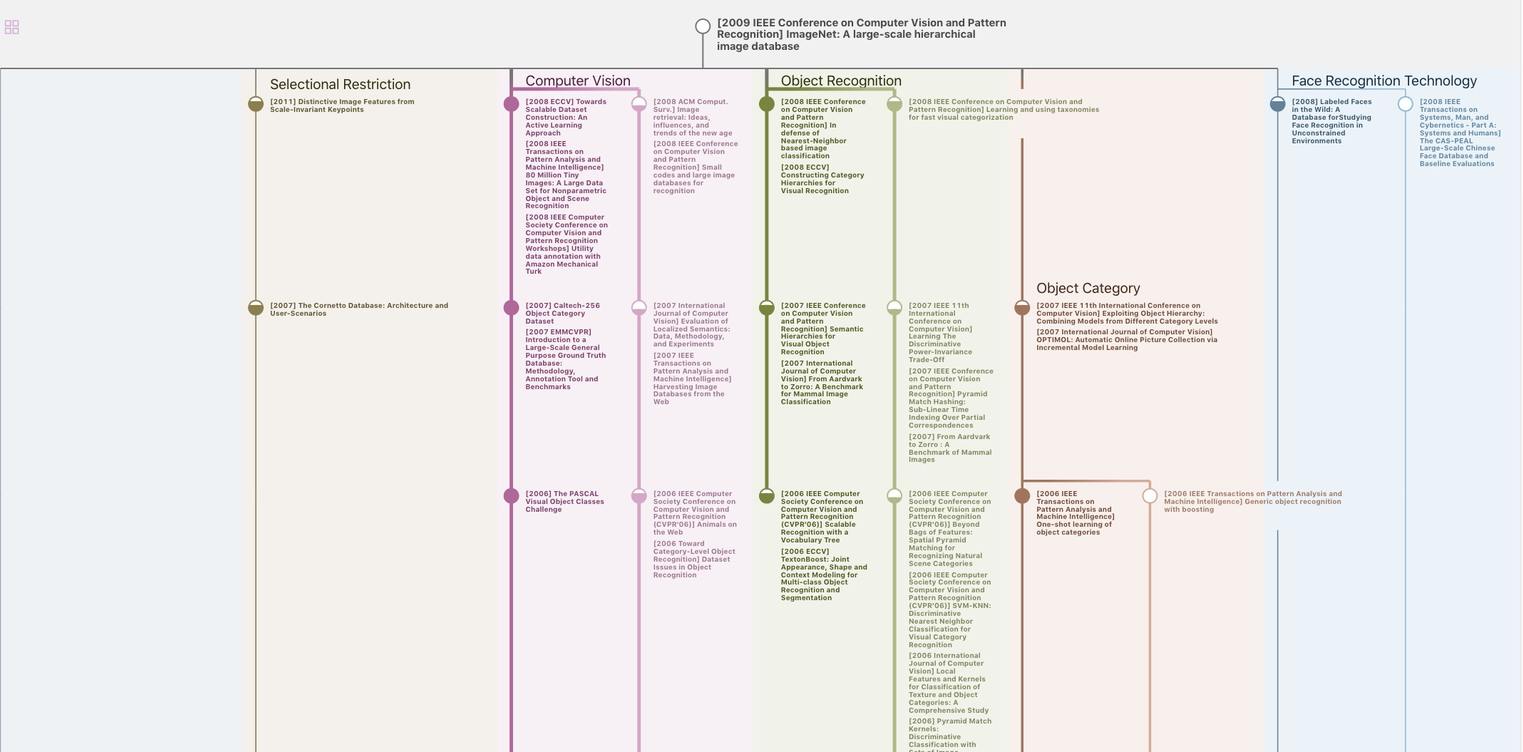
生成溯源树,研究论文发展脉络
Chat Paper
正在生成论文摘要