Emission impacts of left-turn lane on light-heavy-duty mixed traffic in signalized intersections: Optimization and empirical analysis.
Heliyon(2023)
摘要
Reducing emissions from the transport sector is one of the crucial countermeasures for climate action. This study focuses on the optimization and emission analysis regarding the impacts of left-turn lanes on the emissions of mixed traffic flow (CO, HC, and NO) with both heavy-duty vehicles (HDV) and light-duty vehicles (LDV) at urban intersections, combining high-resolution field emission data and simulation tools. Based on high-precision field emission data collected by Portable OBEAS-3000, this study first develops instantaneous emission models for HDV and LDV under various operating conditions. Then, a tailored model is formulated to determine the optimal left-lane length for mixed traffic. Afterward, we empirically validate the model and analyze the effect of the left-turn lane (before and after optimization) on the emissions at the intersections using the established emission models and VISSIM simulations. The proposed method can reduce CO, HC, and NO emissions crossing intersections by around 30% compared to the original scenario. The proposed method significantly reduces average traffic delays after optimization by 16.67% (North), 21.09% (South), 14.61% (West), and 2.68% (East) in different entrance directions. The maximum queue lengths decrease by 79.42%, 39.09%, and 37.02% in different directions. Even though HDVs account for only a minor traffic volume, they contribute the most to CO, HC, and NO emissions at the intersection. The optimality of the proposed method is validated through an enumeration process. Overall, the method provides useful guidance and design methods for traffic designers to alleviate traffic congestion and emissions at urban intersections by strengthening left-turn lanes and improving traffic efficiency.
更多查看译文
关键词
mixed traffic,intersections,left-turn,light-heavy-duty
AI 理解论文
溯源树
样例
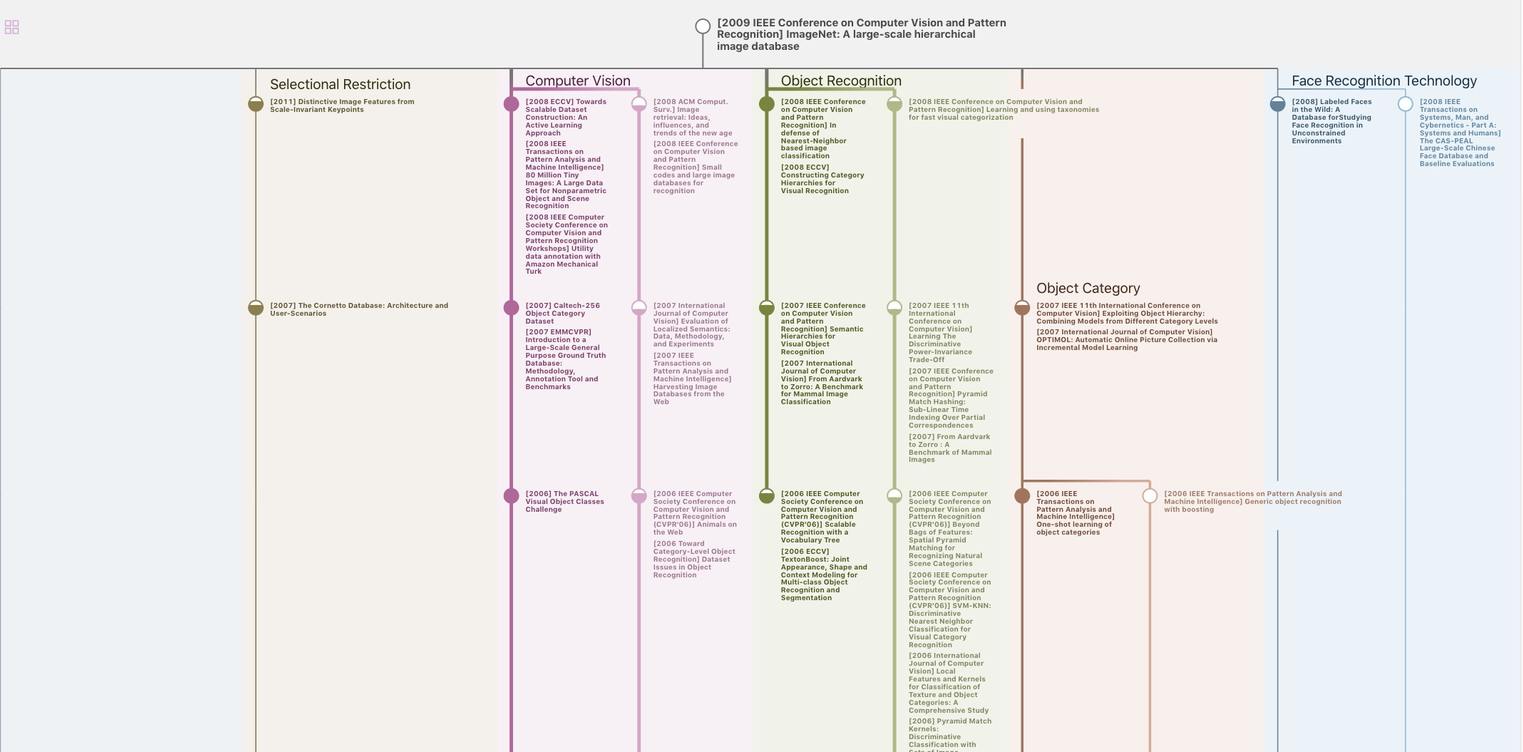
生成溯源树,研究论文发展脉络
Chat Paper
正在生成论文摘要