Deep learning based decision tree ensembles for incomplete medical datasets
TECHNOLOGY AND HEALTH CARE(2024)
摘要
BACKGROUND: In practice, the collected datasets for data analysis are usually incomplete as some data contain missing attribute values. Many related works focus on constructing specific models to produce estimations to replace the missing values, to make the original incomplete datasets become complete. Another type of solution is to directly handle the incomplete datasets without missing value imputation, with decision trees being the major technique for this purpose. OBJECTIVE: To introduce a novel approach, namely Deep Learning-based Decision Tree Ensembles (DLDTE), which borrows the bounding box and sliding window strategies used in deep learning techniques to divide an incomplete dataset into a number of subsets and learning from each subset by a decision tree, resulting in decision tree ensembles. METHOD: Two medical domain problem datasets contain several hundred feature dimensions with the missing rates of 10% to 50% are used for performance comparison. RESULTS: The proposed DLDTE provides the highest rate of classification accuracy when compared with the baseline decision tree method, as well as two missing value imputation methods (mean and k-nearest neighbor), and the case deletion method. CONCLUSION: The results demonstrate the effectiveness of DLDTE for handling incomplete medical datasets with different missing rates.
更多查看译文
关键词
Data science,deep learning,decision trees,classifier ensembles,missing value imputation
AI 理解论文
溯源树
样例
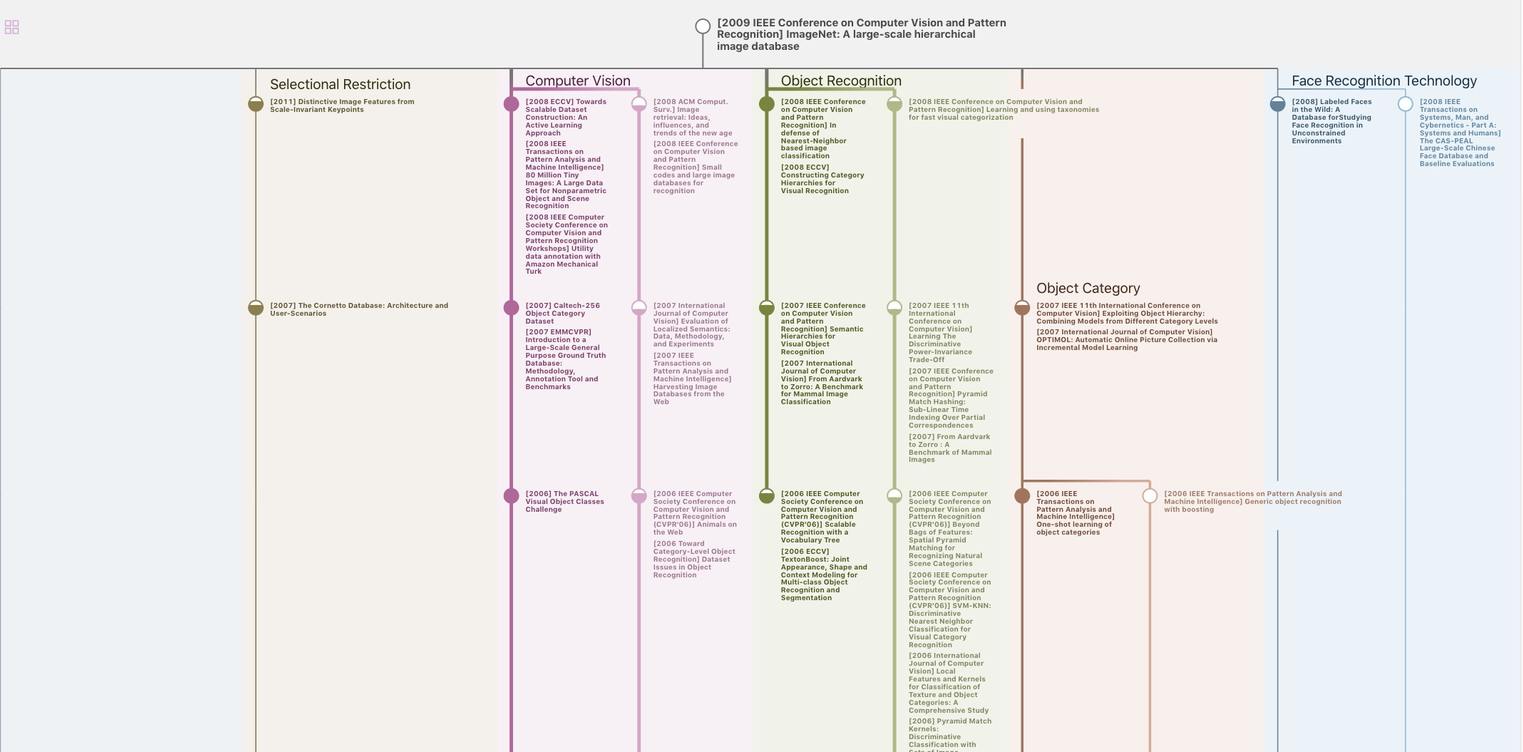
生成溯源树,研究论文发展脉络
Chat Paper
正在生成论文摘要