Fusion-Extracted Features by Deep Networks for Improved COVID-19 Classification with Chest X-ray Radiography.
Healthcare (Basel, Switzerland)(2023)
摘要
Convolutional neural networks (CNNs) have shown promise in accurately diagnosing coronavirus disease 2019 (COVID-19) and bacterial pneumonia using chest X-ray images. However, determining the optimal feature extraction approach is challenging. This study investigates the use of fusion-extracted features by deep networks to improve the accuracy of COVID-19 and bacterial pneumonia classification with chest X-ray radiography. A Fusion CNN method was developed using five different deep learning models after transferred learning to extract image features (Fusion CNN). The combined features were used to build a support vector machine (SVM) classifier with a RBF kernel. The performance of the model was evaluated using accuracy, Kappa values, recall rate, and precision scores. The Fusion CNN model achieved an accuracy and Kappa value of 0.994 and 0.991, with precision scores for normal, COVID-19, and bacterial groups of 0.991, 0.998, and 0.994, respectively. The results indicate that the Fusion CNN models with the SVM classifier provided reliable and accurate classification performance, with Kappa values no less than 0.990. Using a Fusion CNN approach could be a possible solution to enhance accuracy further. Therefore, the study demonstrates the potential of deep learning and fusion-extracted features for accurate COVID-19 and bacterial pneumonia classification with chest X-ray radiography.
更多查看译文
关键词
chest,deep networks,features,fusion-extracted,x-ray
AI 理解论文
溯源树
样例
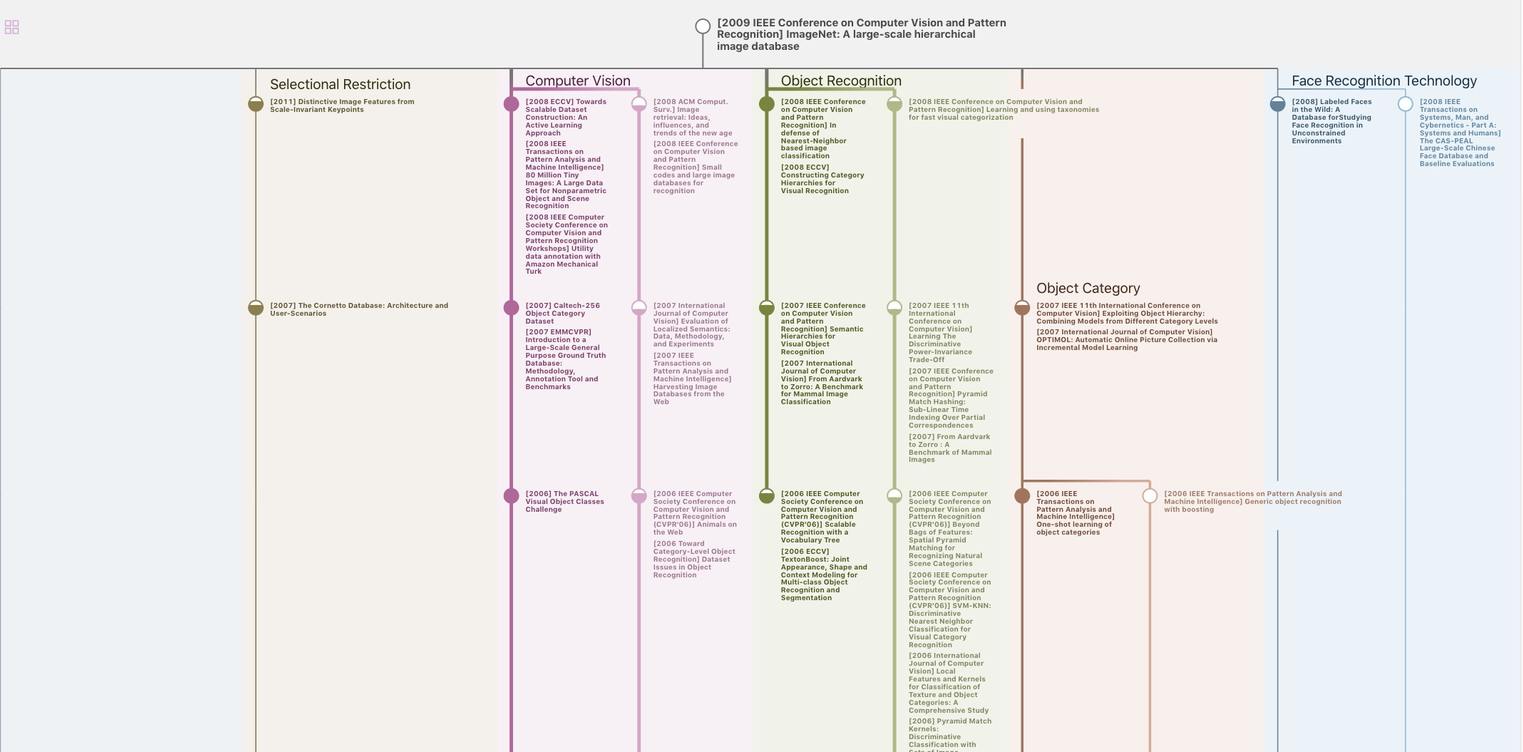
生成溯源树,研究论文发展脉络
Chat Paper
正在生成论文摘要