Comparison of Artificial Intelligence-Based Applications for Mandible Segmentation: From Established Platforms to In-House-Developed Software.
Bioengineering (Basel, Switzerland)(2023)
摘要
Medical image segmentation, whether semi-automatically or manually, is labor-intensive, subjective, and needs specialized personnel. The fully automated segmentation process recently gained importance due to its better design and understanding of CNNs. Considering this, we decided to develop our in-house segmentation software and compare it to the systems of established companies, an inexperienced user, and an expert as ground truth. The companies included in the study have a cloud-based option that performs accurately in clinical routine (dice similarity coefficient of 0.912 to 0.949) with an average segmentation time ranging from 3'54″ to 85'54″. Our in-house model achieved an accuracy of 94.24% compared to the best-performing software and had the shortest mean segmentation time of 2'03″. During the study, developing in-house segmentation software gave us a glimpse into the strenuous work that companies face when offering clinically relevant solutions. All the problems encountered were discussed with the companies and solved, so both parties benefited from this experience. In doing so, we demonstrated that fully automated segmentation needs further research and collaboration between academics and the private sector to achieve full acceptance in clinical routines.
更多查看译文
关键词
artificial intelligence, mandible, segmentation, 3D virtual reconstruction, CBCT, CT, Convolutional Neural Networks, comparison, in-house, software, patch size, Cranio-Maxillofacial surgery, DICOM
AI 理解论文
溯源树
样例
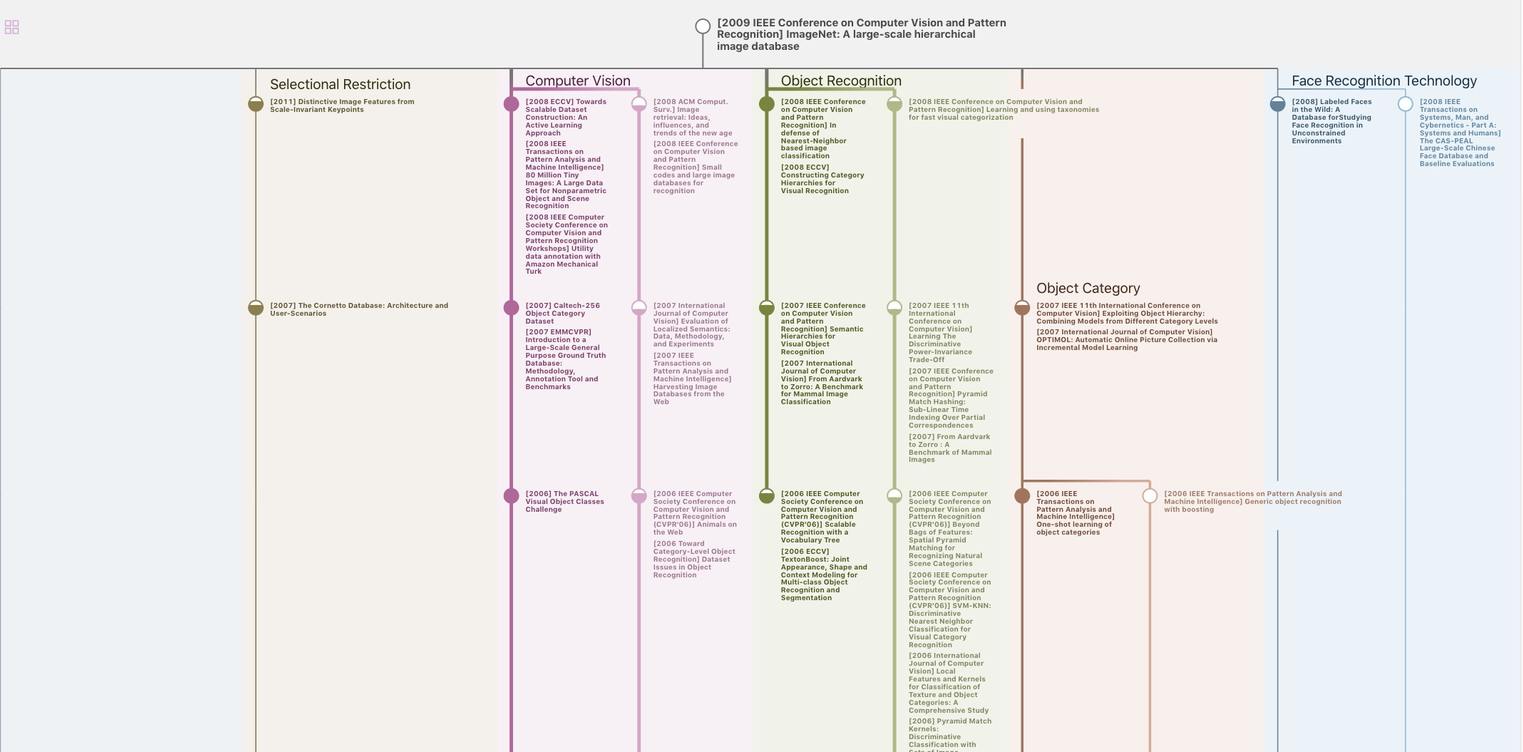
生成溯源树,研究论文发展脉络
Chat Paper
正在生成论文摘要