Reorienting Latent Variable Modeling for Supervised Learning.
Multivariate behavioral research(2023)
摘要
Despite its potentials benefits, using prediction targets generated based on latent variable (LV) modeling is not a common practice in supervised learning, a dominating framework for developing prediction models. In supervised learning, it is typically assumed that the outcome to be predicted is clear and readily available, and therefore validating outcomes before predicting them is a foreign concept and an unnecessary step. The usual goal of LV modeling is inference, and therefore using it in supervised learning and in the prediction context requires a major conceptual shift. This study lays out methodological adjustments and conceptual shifts necessary for integrating LV modeling into supervised learning. It is shown that such integration is possible by combining the traditions of LV modeling, psychometrics, and supervised learning. In this interdisciplinary learning framework, generating practical outcomes using LV modeling and systematically validating them based on clinical validators are the two main strategies. In the example using the data from the Longitudinal Assessment of Manic Symptoms (LAMS) Study, a large pool of candidate outcomes is generated by flexible LV modeling. It is demonstrated that this exploratory situation can be used as an opportunity to tailor desirable prediction targets taking advantage of contemporary science and clinical insights.
更多查看译文
关键词
latent variable modeling,supervised learning
AI 理解论文
溯源树
样例
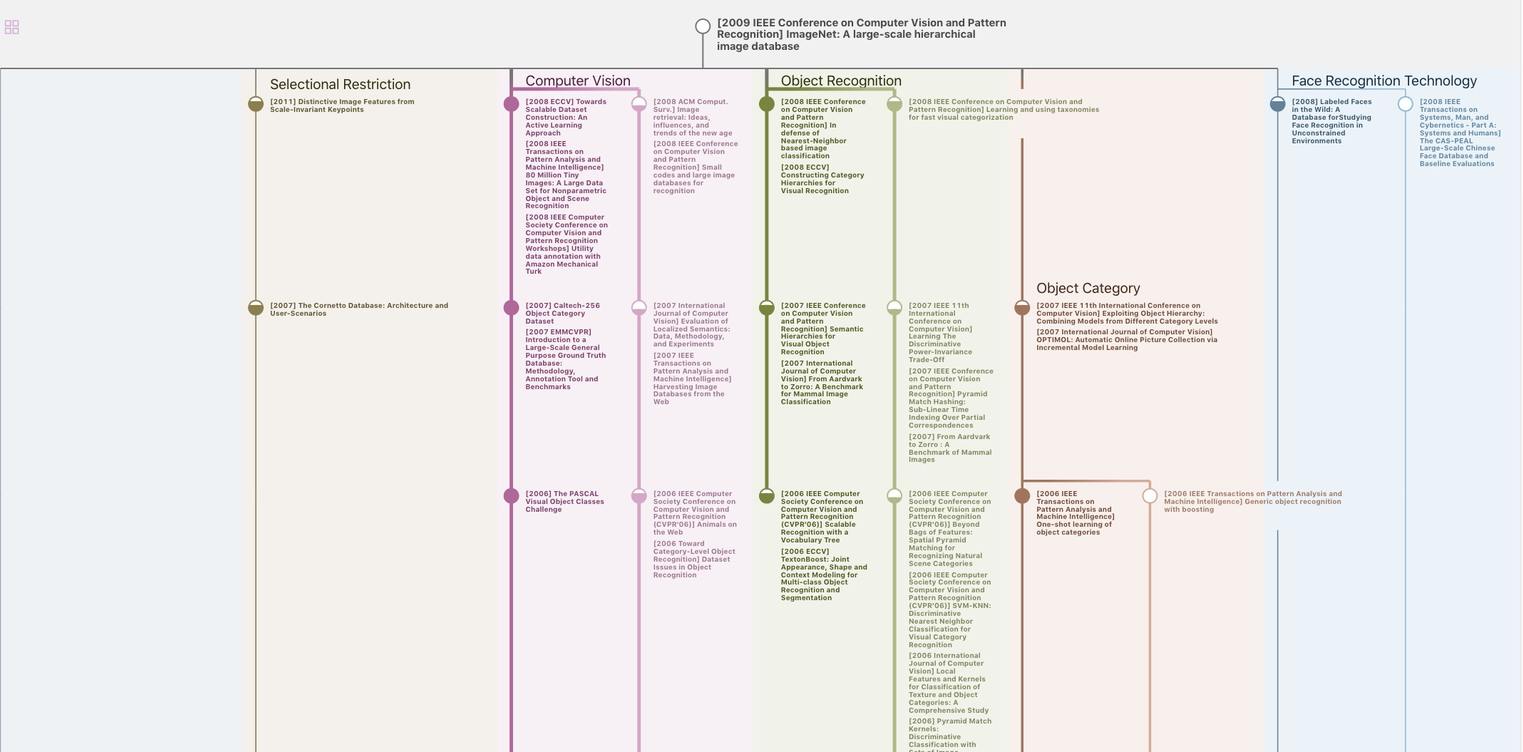
生成溯源树,研究论文发展脉络
Chat Paper
正在生成论文摘要