Machine learning-based signature of necrosis-associated lncRNAs for prognostic and immunotherapy response prediction in cutaneous melanoma and tumor immune landscape characterization.
Frontiers in endocrinology(2023)
摘要
Background:Cutaneous melanoma (CM) is one of the malignant tumors with a relative high lethality. Necroptosis is a novel programmed cell death that participates in anti-tumor immunity and tumor prognosis. Necroptosis has been found to play an important role in tumors like CM. However, the necroptosis-associated lncRNAs' potential prognostic value in CM has not been identified.
Methods:The RNA sequencing data collected from The Cancer Genome Atlas (TCGA) and Genotype-Tissue Expression Project (GTEx) was utilized to identify differentially expressed genes in CM. By using the univariate Cox regression analysis and machine learning LASSO algorithm, a prognostic risk model had been built depending on 5 necroptosis-associated lncRNAs and was verified by internal validation. The performance of this prognostic model was assessed by the receiver operating characteristic curves. A nomogram was constructed and verified by calibration. Furthermore, we also performed sub-group K-M analysis to explore the 5 lncRNAs' expression in different clinical stages. Function enrichment had been analyzed by GSEA and ssGSEA. In addition, qRT-PCR was performed to verify the five lncRNAs' expression level in CM cell line (A2058 and A375) and normal keratinocyte cell line (HaCaT).
Results:We constructed a prognostic model based on five necroptosis-associated lncRNAs (AC245041.1, LINC00665, AC018553.1, LINC01871, and AC107464.3) and divided patients into high-risk group and low-risk group depending on risk scores. A predictive nomogram had been built to be a prognostic indicator to clinical factors. Functional enrichment analysis showed that immune functions had more relationship and immune checkpoints were more activated in low-risk group than that in high-risk group. Thus, the low-risk group would have a more sensitive response to immunotherapy.
Conclusion:This risk score signature could be used to divide CM patients into low- and high-risk groups, and facilitate treatment strategy decision making that immunotherapy is more suitable for those in low-risk group, providing a new sight for CM prognostic evaluation.
更多查看译文
关键词
cutaneous melanoma,immunotherapy response prediction,lncrnas,learning-based,necrosis-associated
AI 理解论文
溯源树
样例
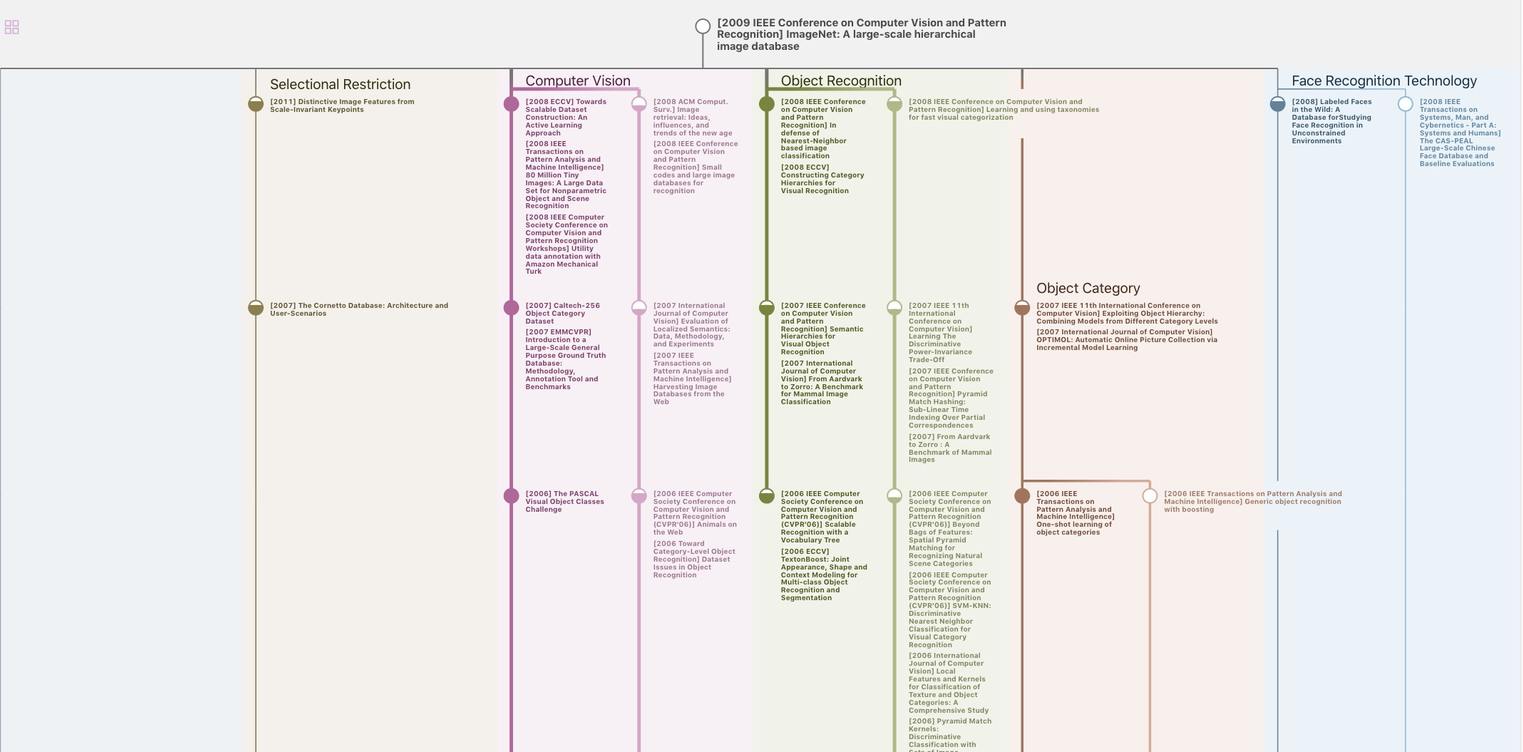
生成溯源树,研究论文发展脉络
Chat Paper
正在生成论文摘要