Learning With Incremental Instances and Features.
IEEE transactions on neural networks and learning systems(2023)
摘要
In many real-world applications, data may dynamically expand over time in both volume and feature dimensions. Besides, they are often collected in batches (also called blocks). We refer this kind of data whose volume and features increase in blocks as blocky trapezoidal data streams. Current works either assume that the feature space of data streams is fixed or stipulate that the algorithm receives only one instance at a time, and none of them can effectively handle the blocky trapezoidal data streams. In this article, we propose a novel algorithm to learn a classification model from blocky trapezoidal data streams, called learning with incremental instances and features (IIF). We attempt to design highly dynamic model update strategies that can learn from increasing training data with an expanding feature space. Specifically, we first divide the data streams obtained on each round and construct the corresponding classifiers for these different divided parts. Then, to realize the effective interaction of information between each classifier, we utilize a single global loss function to capture their relationship. Finally, we use the idea of ensemble to achieve the final classification model. Furthermore, to make this method more applicable, we directly transform it into the kernel method. Both theoretical analysis and empirical analysis validate the effectiveness of our algorithm.
更多查看译文
关键词
Blocky trapezoidal data streams,classification,evolvable features,learning with streaming data
AI 理解论文
溯源树
样例
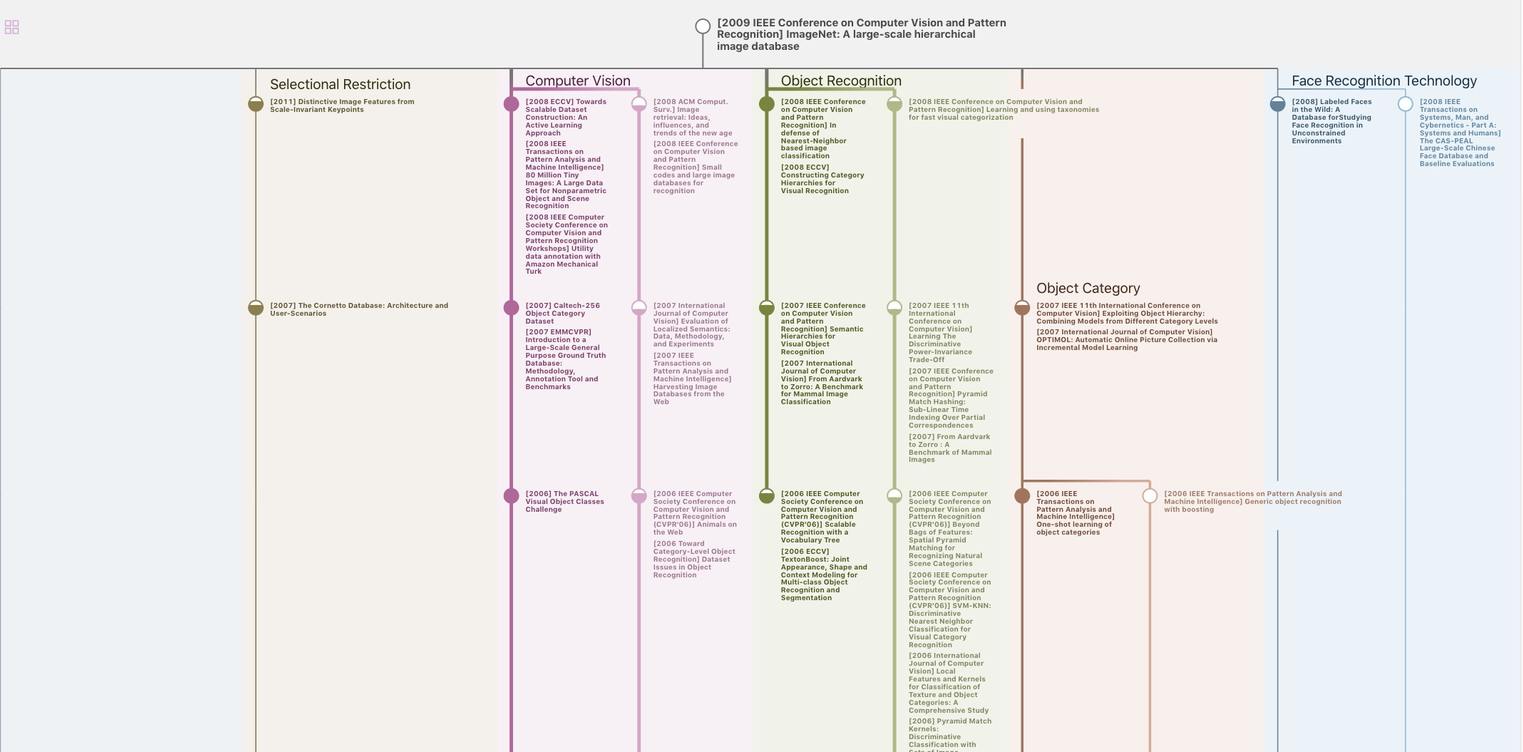
生成溯源树,研究论文发展脉络
Chat Paper
正在生成论文摘要