Prospective Validation of Machine Learning Algorithms for Absorption, Distribution, Metabolism, and Excretion Prediction: An Industrial Perspective.
Journal of chemical information and modeling(2023)
摘要
Absorption, distribution, metabolism, and excretion (ADME), which collectively define the concentration profile of a drug at the site of action, are of critical importance to the success of a drug candidate. Recent advances in machine learning algorithms and the availability of larger proprietary as well as public ADME data sets have generated renewed interest within the academic and pharmaceutical science communities in predicting pharmacokinetic and physicochemical endpoints in early drug discovery. In this study, we collected 120 internal prospective data sets over 20 months across six ADME in vitro endpoints: human and rat liver microsomal stability, MDR1-MDCK efflux ratio, solubility, and human and rat plasma protein binding. A variety of machine learning algorithms in combination with different molecular representations were evaluated. Our results suggest that gradient boosting decision tree and deep learning models consistently outperformed random forest over time. We also observed better performance when models were retrained on a fixed schedule, and the more frequent retraining generally resulted in increased accuracy, while hyperparameters tuning only improved the prospective predictions marginally.
更多查看译文
关键词
excretion prediction,machine learning algorithms,machine learning,metabolism,absorption
AI 理解论文
溯源树
样例
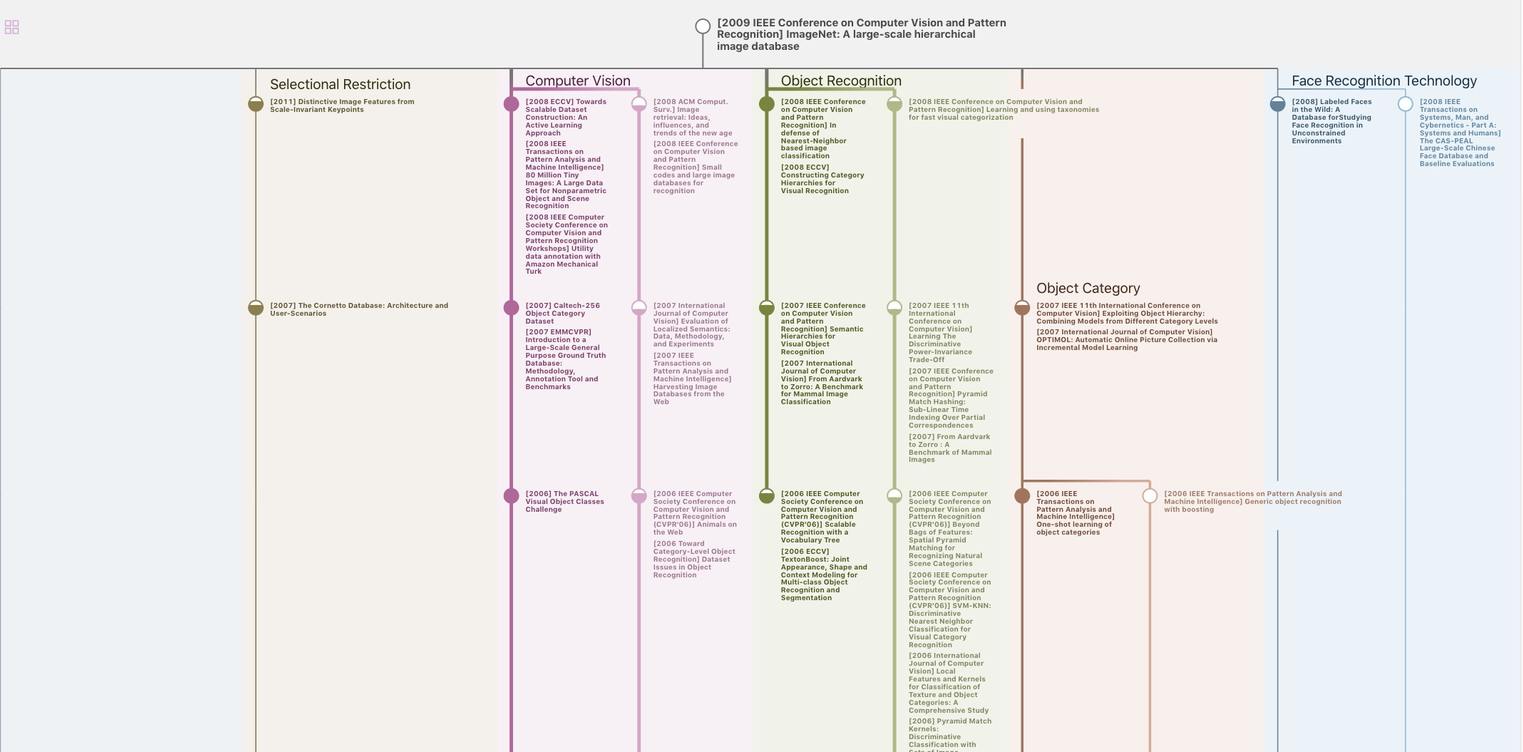
生成溯源树,研究论文发展脉络
Chat Paper
正在生成论文摘要