Self-Supervised Medical Image Denoising Based on WISTA-Net for Human Healthcare in Metaverse.
IEEE journal of biomedical and health informatics(2023)
摘要
Medical image processing plays an important role in the interaction of real world and metaverse for healthcare. Self-supervised denoising based on sparse coding methods, without any prerequisite on large-scale training samples, has been attracting extensive attention for medical image processing. Whereas, existing self-supervised methods suffer from poor performance and low efficiency. In this paper, to achieve state-of-the-art denoising performance on the one hand, we present a self-supervised sparse coding method, named the weighted iterative shrinkage thresholding algorithm (WISTA). It does not rely on noisy-clean ground-truth image pairs to learn from only a single noisy image. On the other hand, to further improve denoising efficiency, we unfold the WISTA to construct a deep neural network (DNN) structured WISTA, named WISTA-Net. Specifically, in WISTA, motivated by the merit of the l-norm, WISTA-Net has better denoising performance than the classical orthogonal matching pursuit (OMP) algorithm and the ISTA. Moreover, leveraging the high-efficiency of DNN structure in parameter updating, WISTA-Net outperforms the compared methods in denoising efficiency. In detail, for a 256 by 256 noisy image, the running time of WISTA-Net is 4.72 s on the CPU, which is much faster than WISTA, OMP, and ISTA by 32.88 s, 13.06 s, and 6.17 s, respectively.
更多查看译文
关键词
Healthcare,metaverse,medical image denoising,self-supervised,sparse coding,ISTA
AI 理解论文
溯源树
样例
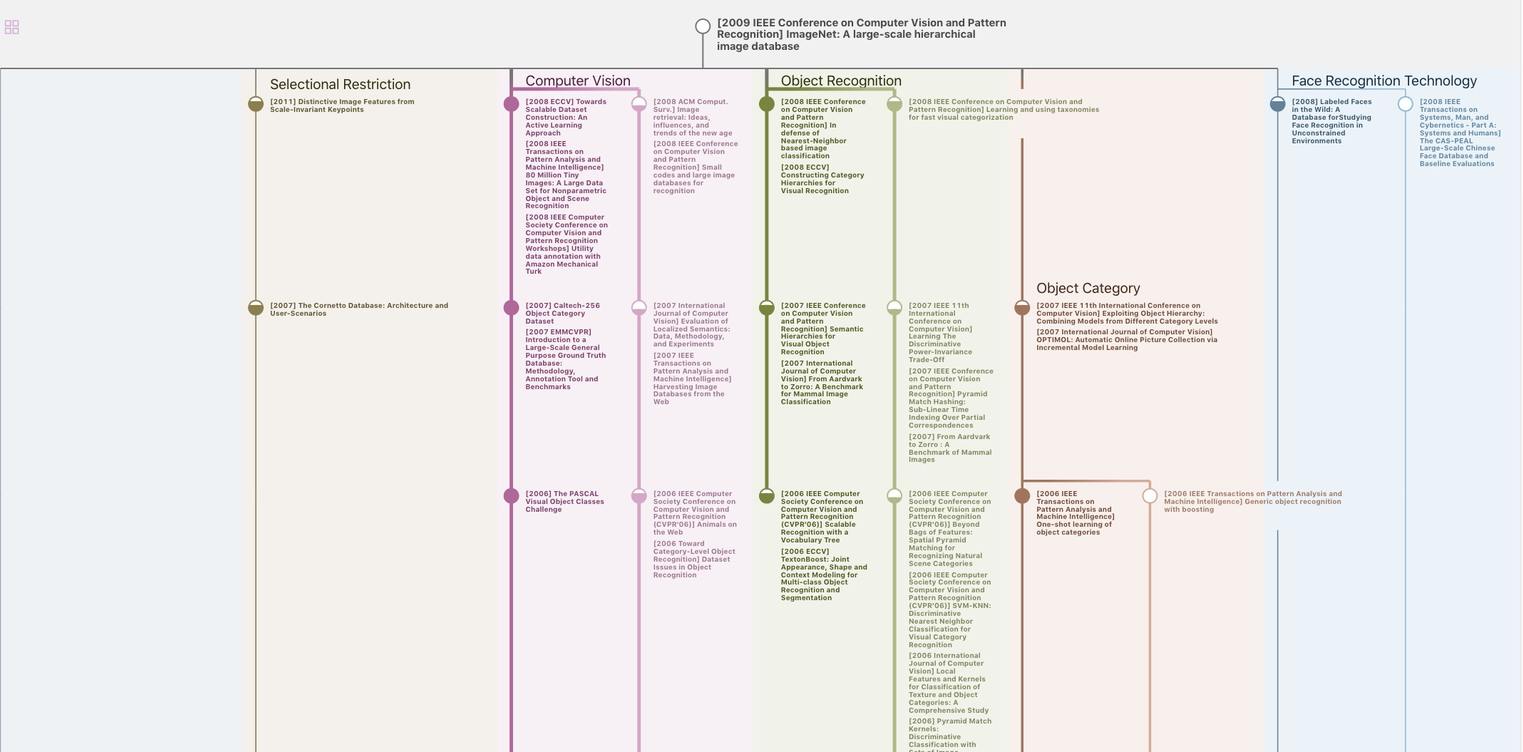
生成溯源树,研究论文发展脉络
Chat Paper
正在生成论文摘要