Prediction of microplastic abundance in surface water of the ocean and influencing factors based on ensemble learning.
Environmental pollution (Barking, Essex : 1987)(2023)
摘要
Microplastics are regarded as emergent contaminants posing a serious threat to the marine ecosystem. It is time-consuming and labor-intensive to determine the number of microplastics in different seas using traditional sampling and detection methods. Machine learning can provide a promising tool for prediction, but there is a lack of research on this. To screen high-performance models for the prediction of microplastic abundance in the marine surface water and explore the influencing factors, three ensemble learning models, random forest (RF), gradient boosted decision tree (GBDT), and extreme gradient boosting (XGBoost), were developed and compared. A total of 1169 samples were collected, and multi-classification prediction models were constructed with 16 features of the data as inputs and six classes of microplastic abundance intervals as outputs. Our results show that the XGBoost model has the best performance of prediction, with a total accuracy rate of 0.719 and an ROC AUC (Receiver Operating Characteristic curve, Area Under Curve) value of 0.914. The distance between the sampling point and the coast (DIS), seawater phosphate (PHOS), and seawater temperature (TEMP) have negative effects on the abundance of microplastics in surface seawater, while the influence of wind stress (WS), human development index (HDI), and sampling latitude (LAT) have positive effects. This work not only predicts the abundance of microplastics in different seas but also offers a framework for the use of machine learning in the study of marine microplastics.
更多查看译文
关键词
Seawater, Microplastics, Abundance, Ensemble learning
AI 理解论文
溯源树
样例
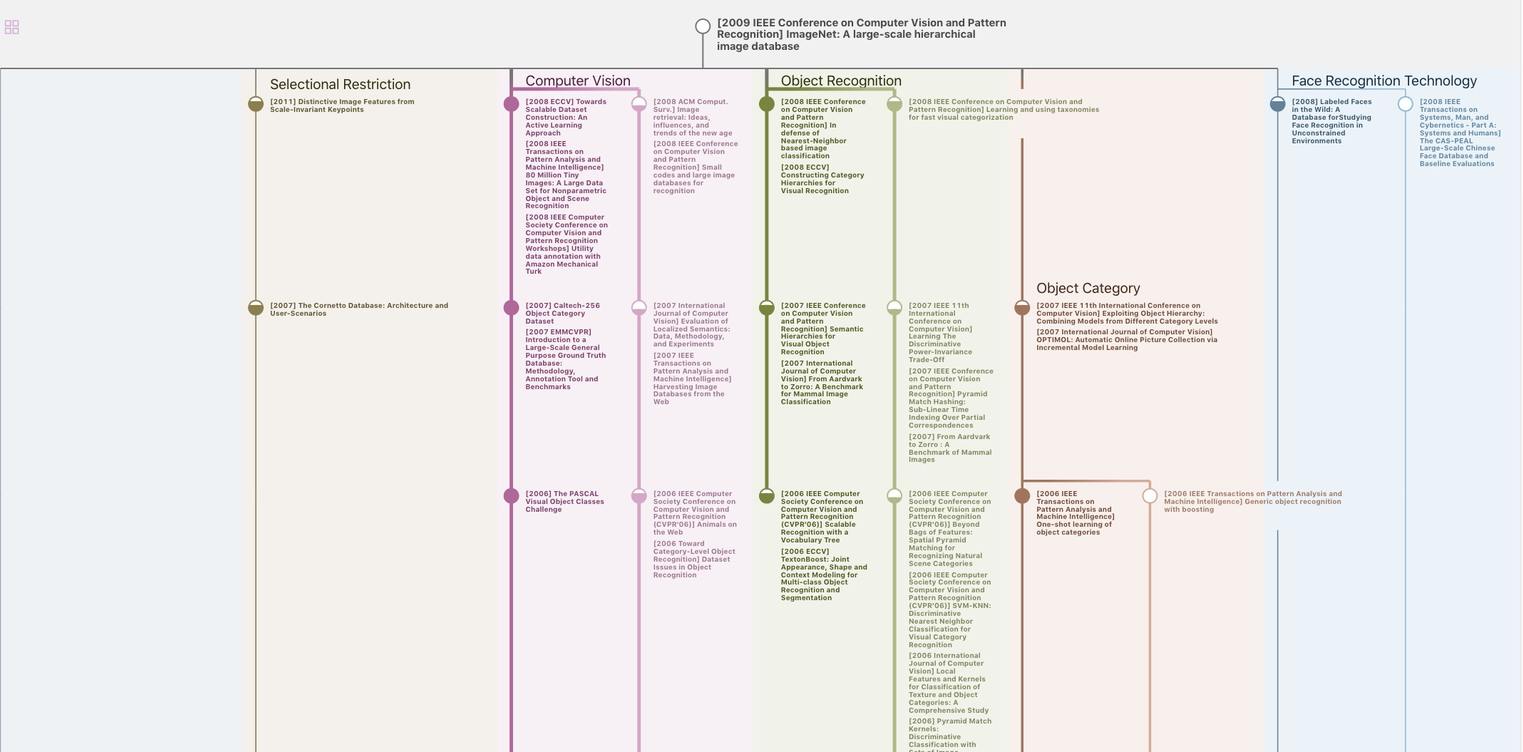
生成溯源树,研究论文发展脉络
Chat Paper
正在生成论文摘要