Decentralized adaptively weighted stacked autoencoder-based incipient fault detection for nonlinear industrial processes.
ISA transactions(2023)
摘要
Modern industrial processes often exhibit large-scale and nonlinear characteristics. Incipient fault detection for industrial processes is a big challenge because of the faint fault signature. To improve the performance of incipient fault detection for large-scale nonlinear industrial processes, a decentralized adaptively weighted stacked autoencoder (DAWSAE) -based fault detection method is proposed. First, the industrial process is divided into several sub-blocks and local adaptively weighted stacked autoencoder (AWSAE) is established for each sub-block to mine local information and obtain local adaptively weighted feature vectors and residual vectors. Second, the global AWSAE is established for the whole process to mine global information and obtain global adaptively weighted feature vectors and residual vectors. Finally, local statistics and global statistics are constructed based on local and global adaptively weighted feature vectors and residual vectors to detect the sub-blocks and the whole process, respectively. The advantages of proposed method are verified by a numerical example and Tennessee Eastman process (TEP).
更多查看译文
AI 理解论文
溯源树
样例
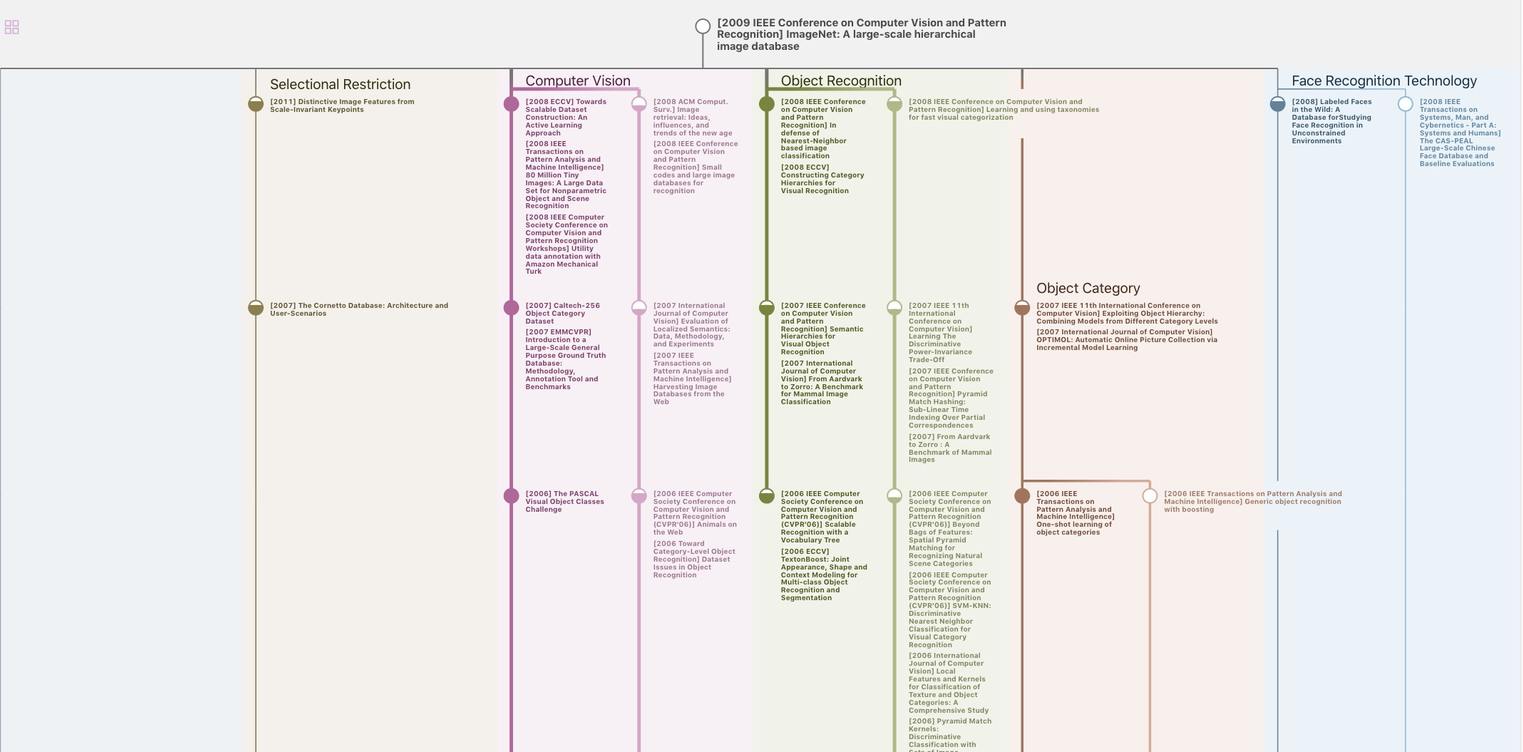
生成溯源树,研究论文发展脉络
Chat Paper
正在生成论文摘要