Persistent Homology-Based Machine Learning Method for Filtering and Classifying Mammographic Microcalcification Images in Early Cancer Detection.
Cancers(2023)
摘要
Microcalcifications in mammogram images are primary indicators for detecting the early stages of breast cancer. However, dense tissues and noise in the images make it challenging to classify the microcalcifications. Currently, preprocessing procedures such as noise removal techniques are applied directly on the images, which may produce a blurry effect and loss of image details. Further, most of the features used in classification models focus on local information of the images and are often burdened with details, resulting in data complexity. This research proposed a filtering and feature extraction technique using persistent homology (PH), a powerful mathematical tool used to study the structure of complex datasets and patterns. The filtering process is not performed directly on the image matrix but through the diagrams arising from PH. These diagrams will enable us to distinguish prominent characteristics of the image from noise. The filtered diagrams are then vectorised using PH features. Supervised machine learning models are trained on the MIAS and DDSM datasets to evaluate the extracted features' efficacy in discriminating between benign and malignant classes and to obtain the optimal filtering level. This study reveals that appropriate PH filtering levels and features can improve classification accuracy in early cancer detection.
更多查看译文
关键词
mammographic microcalcification images,machine learning method,filtering,detection,homology-based
AI 理解论文
溯源树
样例
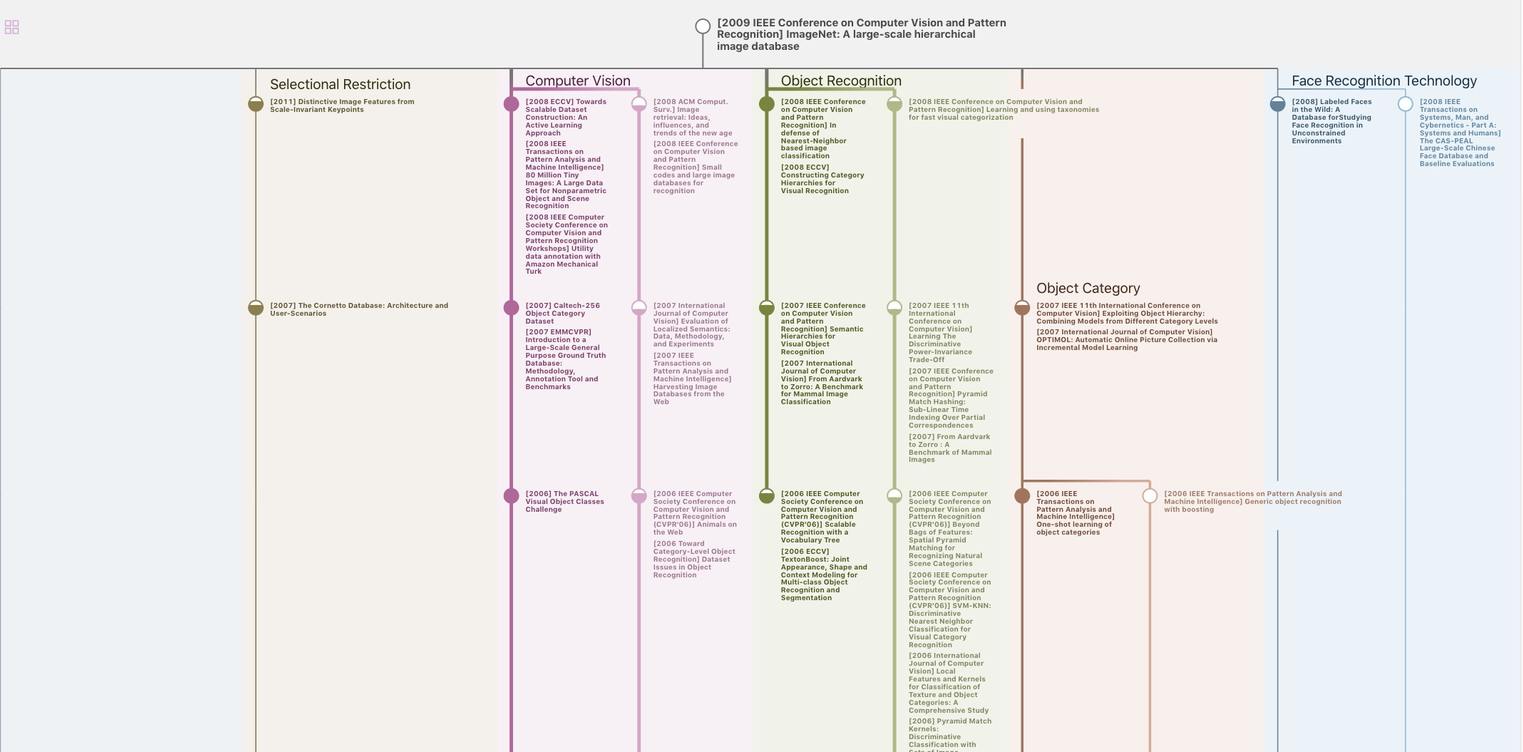
生成溯源树,研究论文发展脉络
Chat Paper
正在生成论文摘要