Optical time-stretch imaging flow cytometry in the compressed domain.
Journal of biophotonics(2023)
摘要
Imaging flow cytometry based on optical time-stretch (OTS) imaging combined with a microfluidic chip attracts much attention in the large-scale single-cell analysis due to its high-throughput, high-precision and label-free operation. Compressive sensing has been integrated into OTS imaging to relieve the pressure on the sampling and transmission of massive data. However, image decompression brings an extra overhead of computing power to the system, but does not generate additional information. In this work, we propose and demonstrate OTS imaging flow cytometry in the compressed domain. Specifically, we constructed a machine-learning network to analyze the cells without decompressing the images. The results show that our system enables high-quality imaging and high-accurate cell classification with an accuracy of over 99% at a compression ratio of 10%. This work provides a viable solution to the big data problem in OTS imaging flow cytometry, boosting its application in practice. This article is protected by copyright. All rights reserved.
更多查看译文
关键词
flow cytometry,imaging,optical,compressed domain
AI 理解论文
溯源树
样例
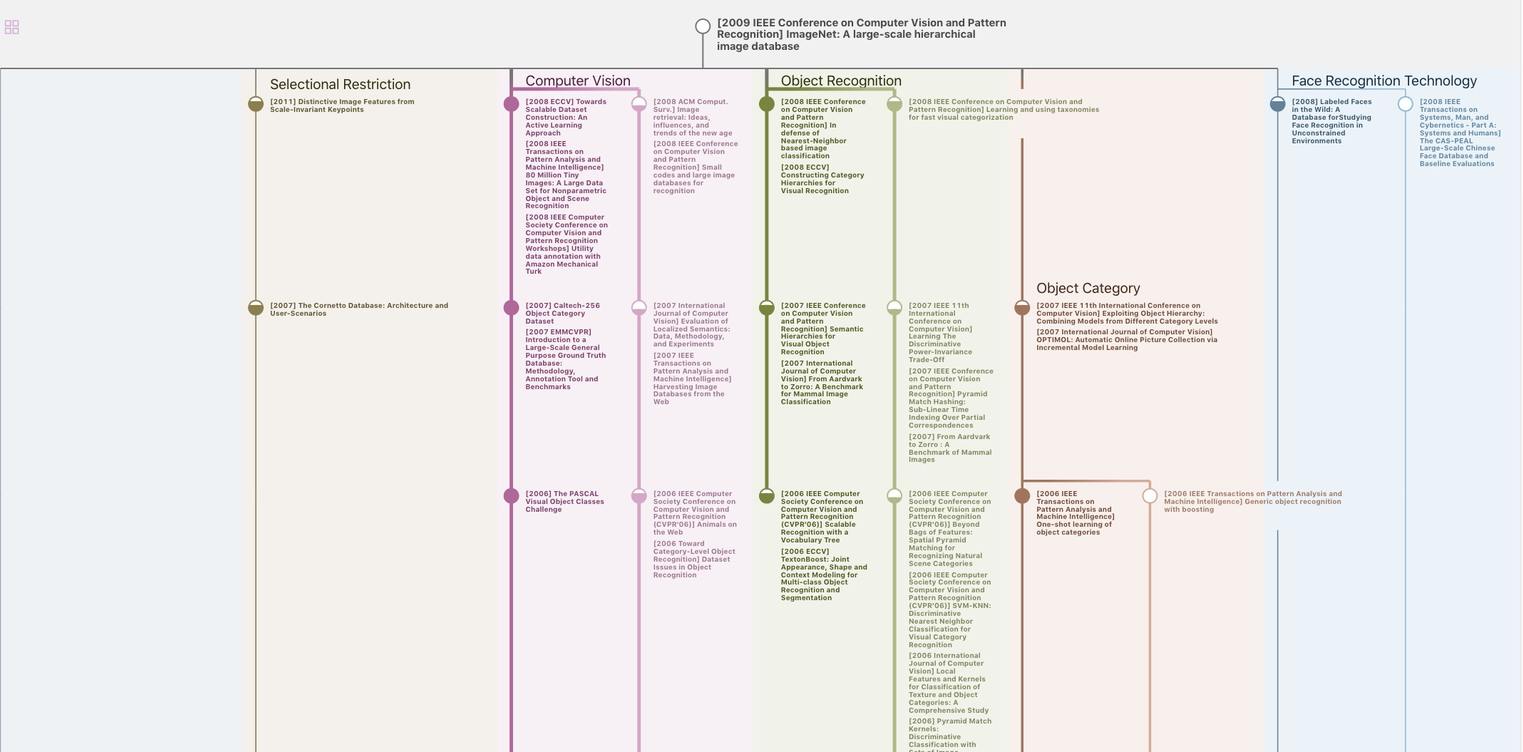
生成溯源树,研究论文发展脉络
Chat Paper
正在生成论文摘要