Machine learning based on gadoxetic acid-enhanced MRI for differentiating atypical intrahepatic mass-forming cholangiocarcinoma from poorly differentiated hepatocellular carcinoma
Abdominal radiology (New York)(2023)
摘要
Purpose The study was to develop a Gd-EOB-DTPA-enhanced MRI radiomics model for differentiating atypical intrahepatic mass-forming cholangiocarcinoma (aIMCC) from poorly differentiated hepatocellular carcinoma (pHCC). Materials and methods A total of 134 patients (51 aIMCC and 83 pHCC) who underwent Gadoxetic acid-enhanced MRI between March 2016 and March 2022 were enrolled in this study and then randomly assigned to the training and validation cohorts by 7:3 (93 patients and 41 patients, respectively). The radiomics features were extracted from the hepatobiliary phase of Gadoxetic acid-enhanced MRI. In the training cohort, the SelectKBest and the least absolute shrinkage and selection operator (LASSO) were used to select the radiomics features. The clinical, radiomics, and clinical-radiomics model were established using four machine learning algorithms. The performance of the model was evaluated by the receiver operating characteristic (ROC) curve. Comparison of the radiomics and clinical-radiomics model was done by the Delong test. The clinical usefulness of the model was evaluated using decision curve analysis (DCA). Results In 1132 extracted radiomic features, 15 were selected to develop radiomics signature. For identifying aIMCC and pHCC, the radiomics model constructed by random forest algorithm showed the high performance (AUC = 0.90) in the training cohort. The performance of the clinical-radiomics model (AUC = 0.89) was not significantly different ( P = 0.88) from that of the radiomics model constructed by random forest algorithm (AUC = 0.86) in the validation cohort. DCA demonstrated that the clinical-radiomics model constructed by random forest algorithm had a high net clinical benefit. Conclusion The clinical-radiomics model is an effective tool to distinguish aIMCC from pHCC and may provide additional value for the development of treatment plans. Graphical abstract
更多查看译文
关键词
Hepatocellular carcinoma,Cholangiocarcinoma,Gadoxetic acid,Magnetic resonance imaging,Machine learning
AI 理解论文
溯源树
样例
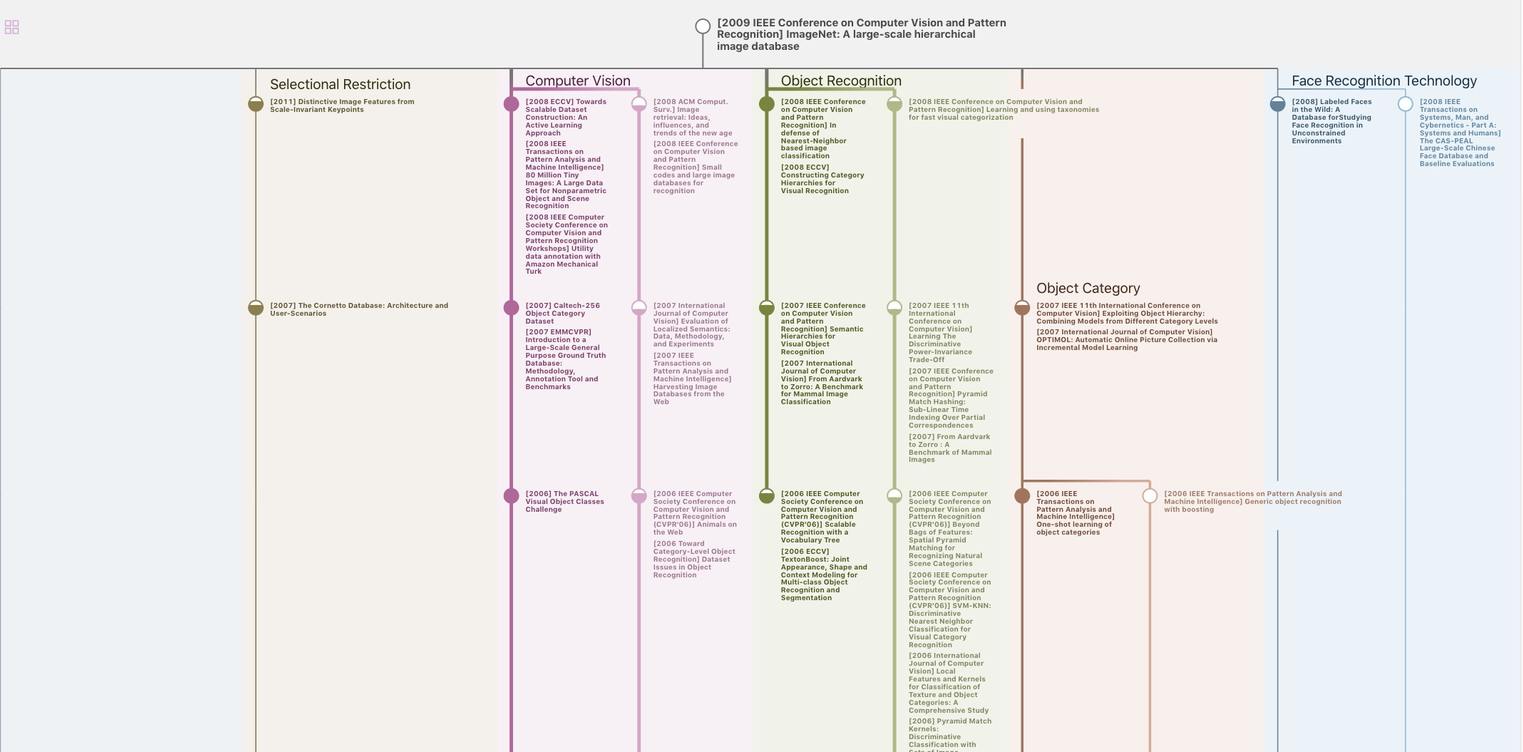
生成溯源树,研究论文发展脉络
Chat Paper
正在生成论文摘要