ProtoHAR: Prototype Guided Personalized Federated Learning for Human Activity Recognition.
IEEE journal of biomedical and health informatics(2023)
摘要
Federated Learning (FL) has recently attracted great interest in sensor-based human activity recognition (HAR) tasks. However, in real-world environment, sensor data on devices is non-independently and identically distributed (Non-IID), e.g., activity data recorded by most devices is sparse, and sensor data distribution for each client may be inconsistent. As a result, the traditional FL methods in the heterogeneous environment may incur a drifted global model that causes slow convergence and a heavy communication burden. Although some FL methods are gradually being applied to HAR, they are designed for overly ideal scenarios and do not address such Non-IID problem in the real-world setting. It is still a question whether they can be applied to cross-device FL. To tackle this challenge, we propose ProtoHAR, a prototype-guided FL framework for HAR, which aims to decouple the representation and classifier in the heterogeneous FL setting efficiently. It leverages the global prototype to correct the activity feature representation to make the prototype knowledge flow among clients without leaking privacy while solving a better classifier to avoid excessive drift of the local model in personalized training. Extensive experiments are conducted on four publicly available datasets: USC-HAD, UNIMIB-SHAR, PAMAP2, and HARBOX, which are collected in both controlled environments and real-world scenarios. The results show that compared with the state-of-the-art FL algorithms, ProtoHAR achieves the best performance and faster convergence speed in HAR datasets.
更多查看译文
关键词
Human activity recognition,federated learning,representation learning,sensor,deep learning
AI 理解论文
溯源树
样例
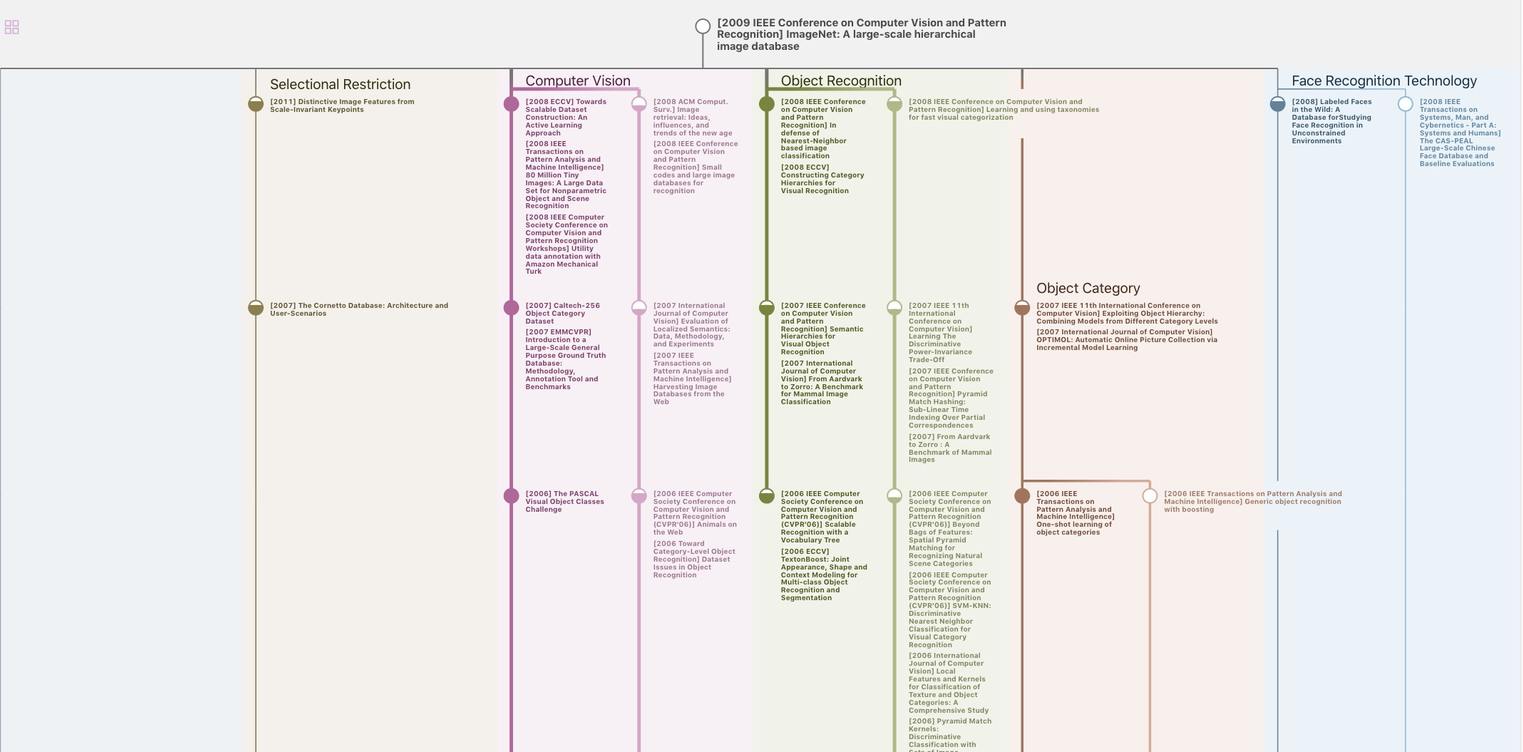
生成溯源树,研究论文发展脉络
Chat Paper
正在生成论文摘要