Source-Free Domain Adaptation (SFDA) for Privacy-Preserving Seizure Subtype Classification.
IEEE transactions on neural systems and rehabilitation engineering : a publication of the IEEE Engineering in Medicine and Biology Society(2023)
摘要
Electroencephalogram (EEG) based seizure subtype classification is very important in clinical diagnostics. Source-free domain adaptation (SFDA) uses a pre-trained source model, instead of the source data, for privacy-preserving transfer learning. SFDA is useful in seizure subtype classification, which can protect the privacy of the source patients, while reducing the amount of labeled calibration data for a new patient. This paper introduces semi-supervised transfer boosting (SS-TrBoosting), a boosting-based SFDA approach for seizure subtype classification. We further extend it to unsupervised transfer boosting (U-TrBoosting) for unsupervised SFDA, i.e., the new patient does not need any labeled EEG data. Experiments on three public seizure datasets demonstrated that SS-TrBoosting and U-TrBoosting outperformed multiple classical and state-of-the-art machine learning approaches in cross-dataset/cross-patient seizure subtype classification.
更多查看译文
关键词
Boosting, ensemble learning, EEG, seizure subtype classification, source-free domain adaptation, transfer learning
AI 理解论文
溯源树
样例
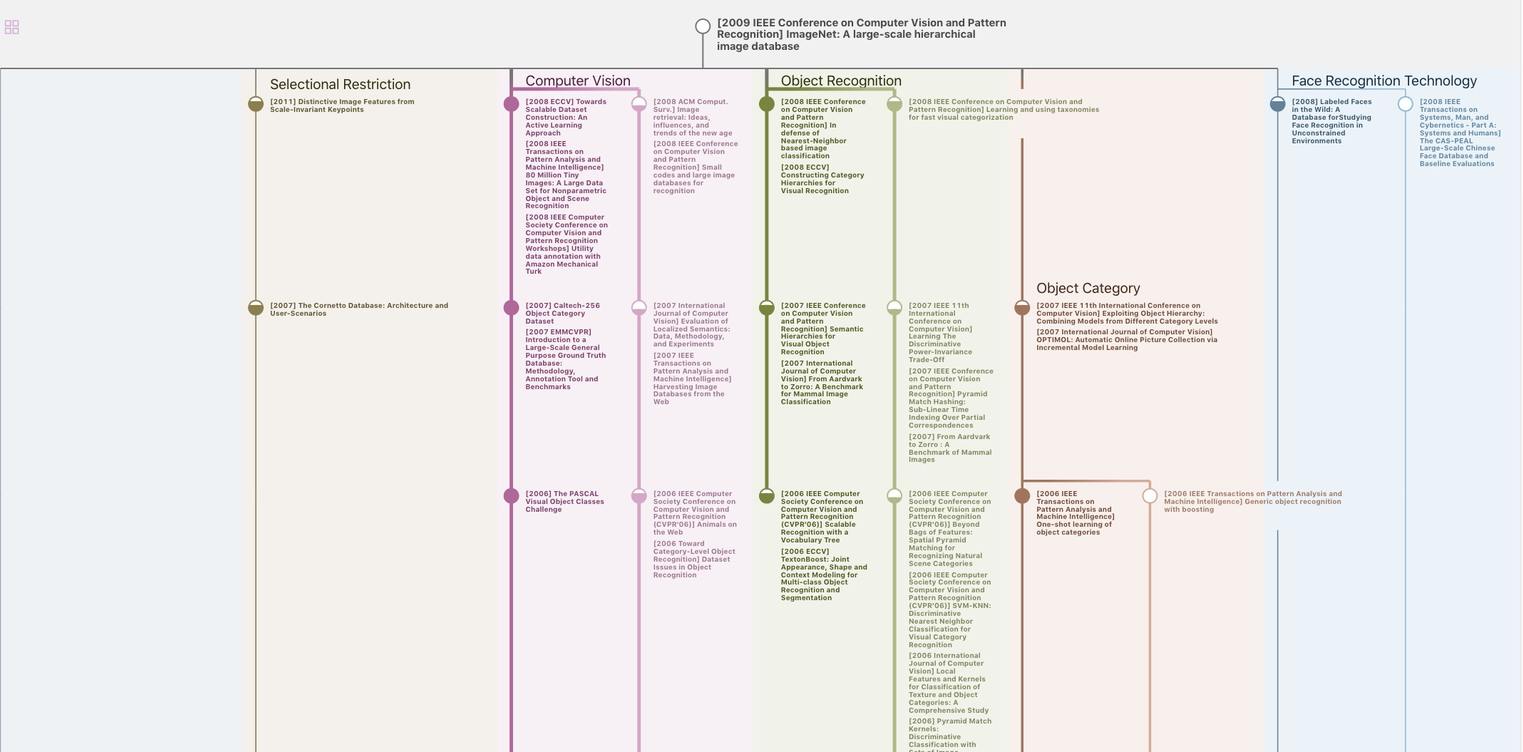
生成溯源树,研究论文发展脉络
Chat Paper
正在生成论文摘要