Variational Data-Free Knowledge Distillation for Continual Learning.
IEEE transactions on pattern analysis and machine intelligence(2023)
摘要
Deep neural networks suffer from catastrophic forgetting when trained on sequential tasks in continual learning. Various methods rely on storing data of previous tasks to mitigate catastrophic forgetting, which is prohibited in real-world applications considering privacy and security issues. In this paper, we consider a realistic setting of continual learning, where training data of previous tasks are unavailable and memory resources are limited. We contribute a novel knowledge distillation-based method in an information-theoretic framework by maximizing mutual information between outputs of previously learned and current networks. Due to the intractability of computation of mutual information, we instead maximize its variational lower bound, where the covariance of variational distribution is modeled by a graph convolutional network. The inaccessibility of data of previous tasks is tackled by Taylor expansion, yielding a novel regularizer in network training loss for continual learning. The regularizer relies on compressed gradients of network parameters. It avoids storing previous task data and previously learned networks. Additionally, we employ self-supervised learning technique for learning effective features, which improves the performance of continual learning. We conduct extensive experiments including image classification and semantic segmentation, and the results show that our method achieves state-of-the-art performance on continual learning benchmarks.
更多查看译文
关键词
distillation,learning,knowledge,data-free
AI 理解论文
溯源树
样例
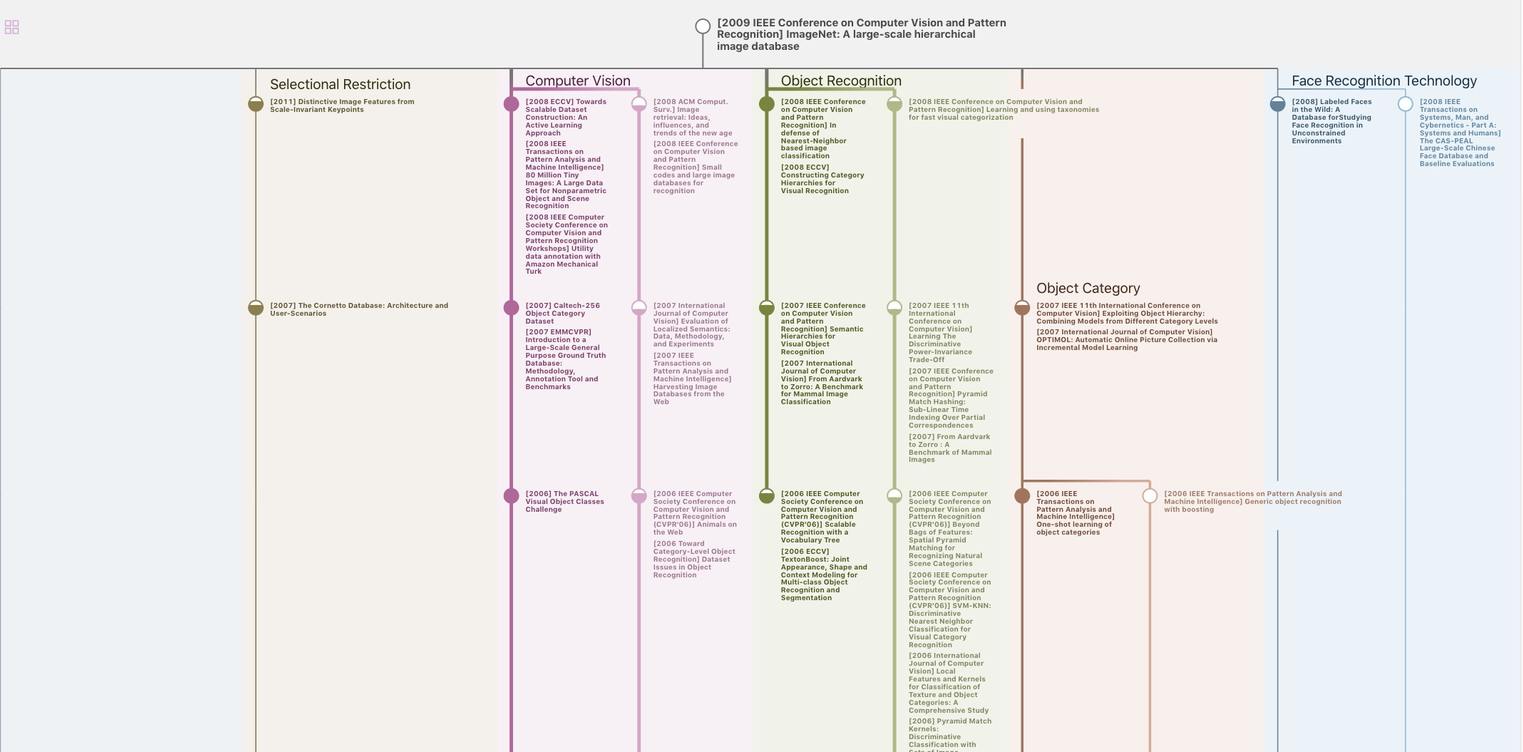
生成溯源树,研究论文发展脉络
Chat Paper
正在生成论文摘要