HuCurl: Human-induced Curriculum Discovery
conf_acl(2023)
摘要
We introduce the problem of curriculum discovery and describe a curriculum learning framework capable of discovering effective curricula in a curriculum space based on prior knowledge about sample difficulty. Using annotation entropy and loss as measures of difficulty, we show that (i): the top-performing discovered curricula for a given model and dataset are often non-monotonic as apposed to monotonic curricula in existing literature, (ii): the prevailing easy-to-hard or hard-to-easy transition curricula are often at the risk of underperforming, and (iii): the curricula discovered for smaller datasets and models perform well on larger datasets and models respectively. The proposed framework encompasses some of the existing curriculum learning approaches and can discover curricula that outperform them across several NLP tasks.
更多查看译文
关键词
discovery,human-induced
AI 理解论文
溯源树
样例
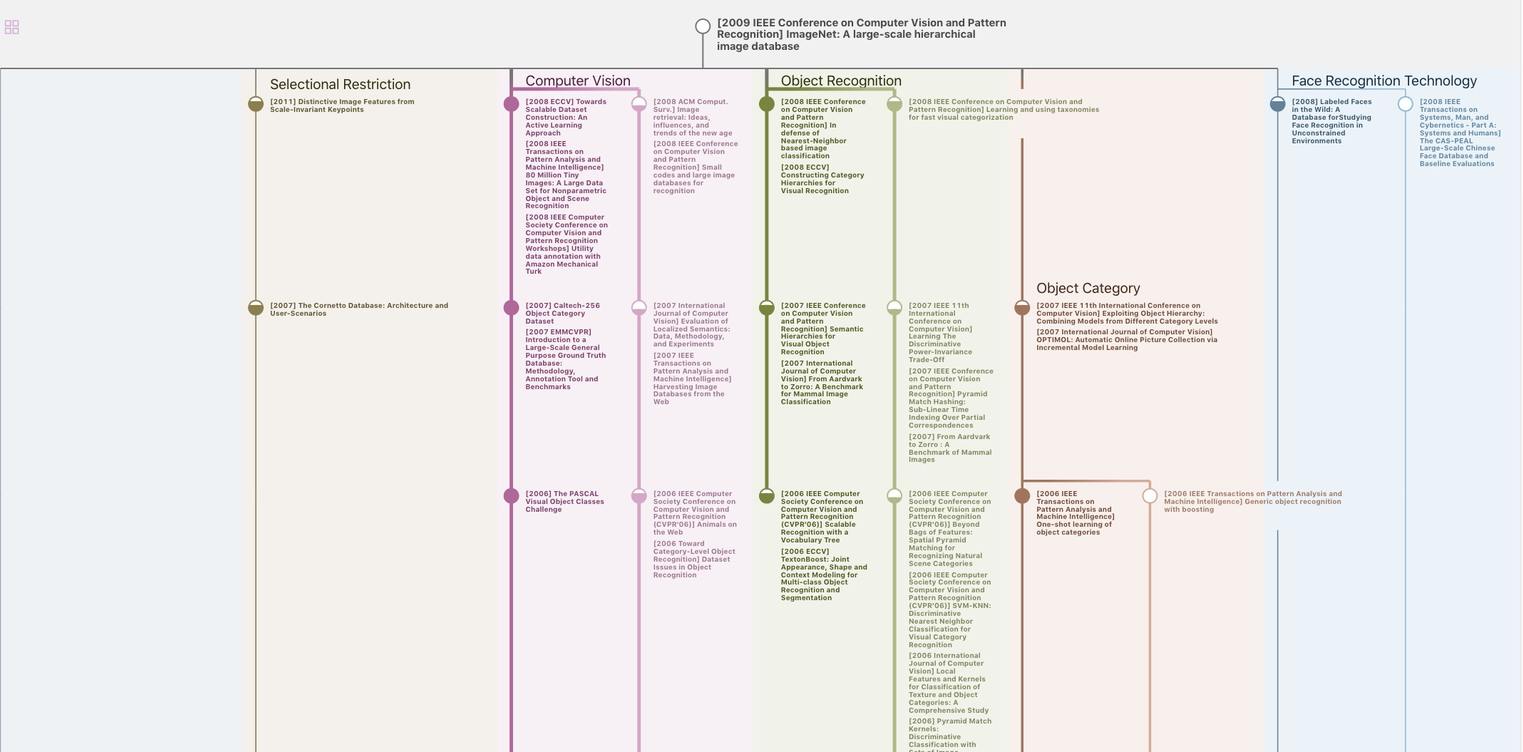
生成溯源树,研究论文发展脉络
Chat Paper
正在生成论文摘要