Two Examples are Better than One: Context Regularization for Gradient-based Prompt Tuning
conf_acl(2023)
摘要
Prompting has gained tremendous attention as an efficient method for the adaptation of large-scale language models.However, prompts often act against human intuition and report unstable performances, which has motivated methods that automatically find effective prompts.One popular approach is gradient-based search, which iteratively updates a (randomly) initialized prompt towards the optimal one with the guide of gradients.We propose a novel regularization method, CoRe, for gradient-based prompt tuning techniques, which guides a prompt to produce a task context properly.CoRe realizes two regularization effects — context attuning and context filtering — that improve prediction performance in a zero-shot in-context learning setting where a model makes inferences only with the prompt tuned by CoRe, without any demonstration examples for in-context learning.Context attuning guides the context generated by the input and the tuned prompt toward embedding the appropriate context for the task.In our theoretical analysis, regularizing the context extends to improving zero-shot in-context learning performance.Context filtering steers the prompt to select only the task-related context so that context attuning solely focuses on creating and sending the right task context.We evaluate CoRe on natural language understanding datasets and two large language models, GPT2-XL and GPT-J.Our training scheme shows performance improvements up to 11.9% on GPT2-XL, and up to 6.3% on GPT-J in zero-shot settings.
更多查看译文
AI 理解论文
溯源树
样例
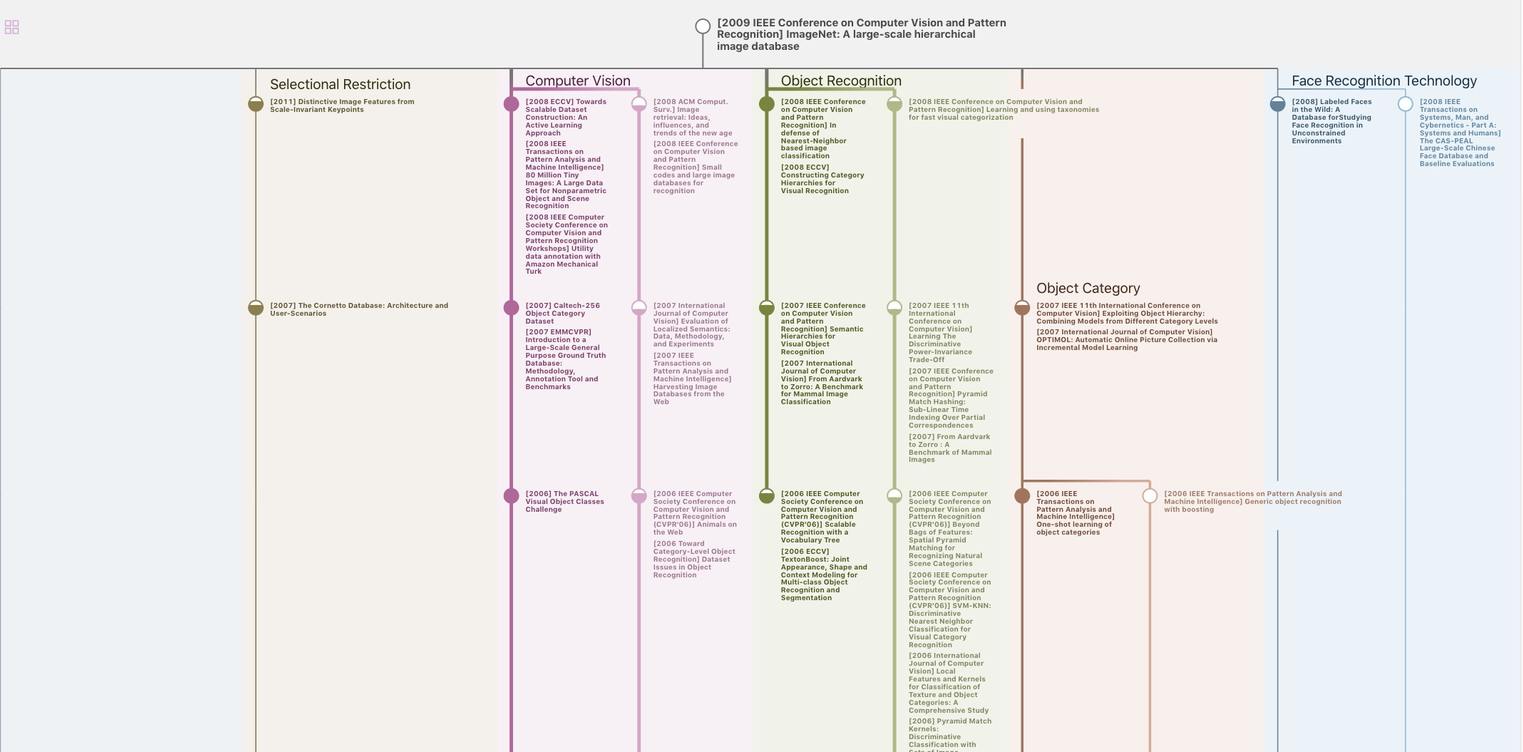
生成溯源树,研究论文发展脉络
Chat Paper
正在生成论文摘要