Rapid Diffusion: Building Domain-Specific Text-to-Image Synthesizers with Fast Inference Speed
conf_acl(2023)
摘要
Text-to-Image Synthesis (TIS) aims to generate images based on textual inputs. Recently, several large pre-trained diffusion models have been released to create high-quality images with pre-trained text encoders and diffusion-based image synthesizers. However, popular diffusion-based models from the open-source community cannot support industrial domain-specific applications due to the lack of entity knowledge and low inference speed.In this paper, we propose Rapid Diffusion, a novel framework for training and deploying super-resolution, text-to-image latent diffusion models with rich entity knowledge injected and optimized networks.Furthermore, we employ BladeDISC, an end-to-end Artificial Intelligence (AI) compiler, and FlashAttention techniques to optimize computational graphs of the generated models for online deployment. Experiments verify the effectiveness of our approach in terms of image quality and inference speed. In addition, we present industrial use cases and integrate Rapid Diffusion to an AI platform to show its practical values.
更多查看译文
AI 理解论文
溯源树
样例
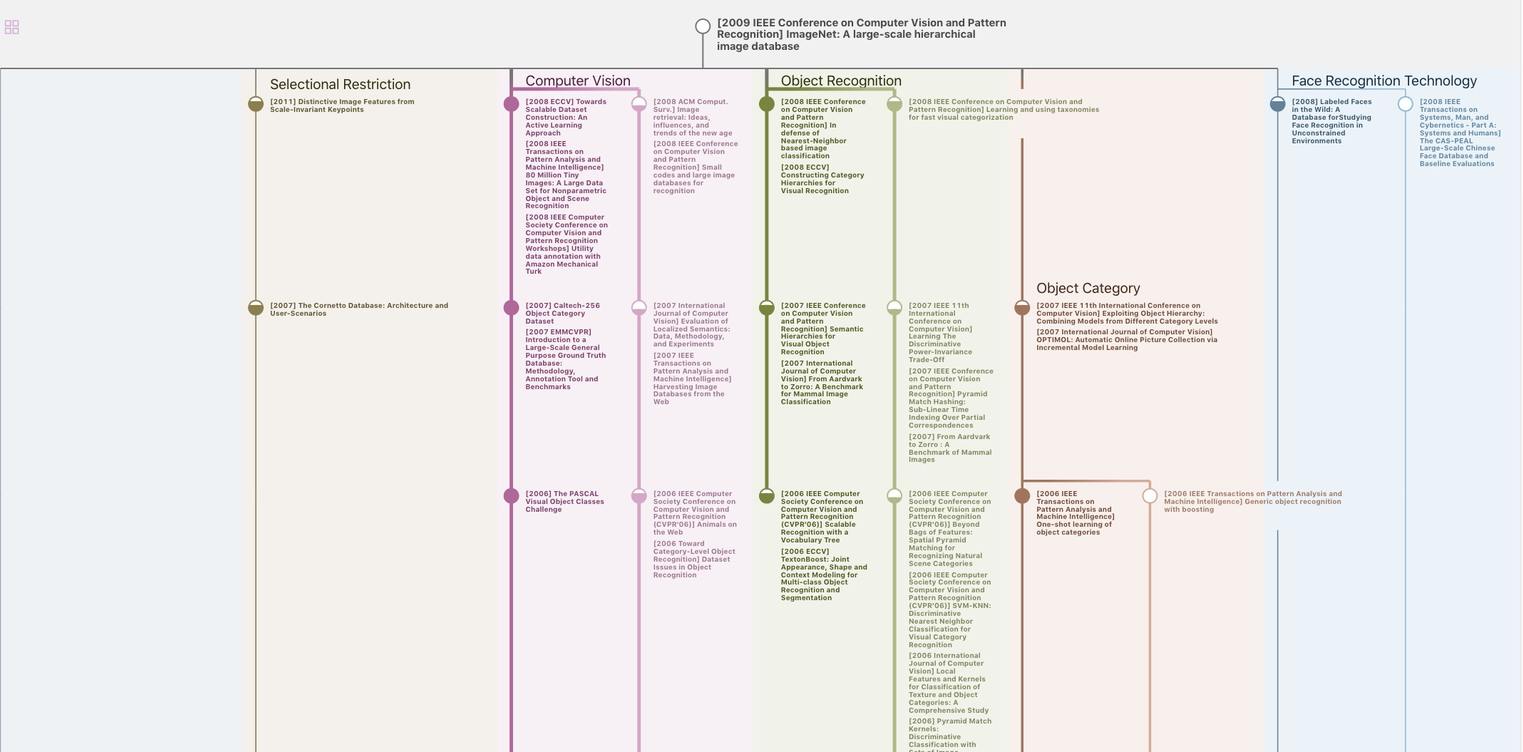
生成溯源树,研究论文发展脉络
Chat Paper
正在生成论文摘要