Research on early diagnosis of Alzheimer's disease based on dual fusion cluster graph convolutional network
Biomedical Signal Processing and Control(2023)
摘要
Objective: Mild Cognitive Impairment (MCI) is an early stage of Alzheimer's Disease (AD), often mistaken for natural aging. Early detection and treatment of MCI are crucial for effective treatment, but the condition can be difficult to diagnose. In recent years, multi-modal data and deep learning methods have shown promise in this field. The objective of this study is to develop a computer-aided MCI diagnosis system that effectively processes multi-modal data using deep learning methods.Method: We proposed a Dual Fusion Cluster Graph Convolution Network (DFCGCN) model, which combines two channels of feature extraction, one adjacency matrix, and the Cluster GCN in series. Brain imaging is downsampled using graph pooling and flattened into sparse vectors, from which advanced features are extracted. Similarity between connectivity matrices is calculated using the Gaussian kernel function and combined with non-imaging details to construct a population graph that better represents inter-subject variability. Finally, features are assigned to subjects in the population graph, and node embeddings are learned using Cluster GCN to output diagnostic results.Result: We tested the proposed algorithm on the public Alzheimer's Disease Neuroimaging Initiative (ADNI) dataset, achieving an accuracy, sensitivity, and specificity of 90.7%, 91.1%, and 94.0%, respectively.Conclusion: The DFCGCN model presented in this study enhances the diagnosis of MCI and outperforms other state-of-the-art algorithms. This approach has potential to be a valuable tool for early detection and treatment of MCI.
更多查看译文
关键词
Graph convolution neural network,Multi-modal,Feature extraction,Adjacency matrix,ADNI dataset
AI 理解论文
溯源树
样例
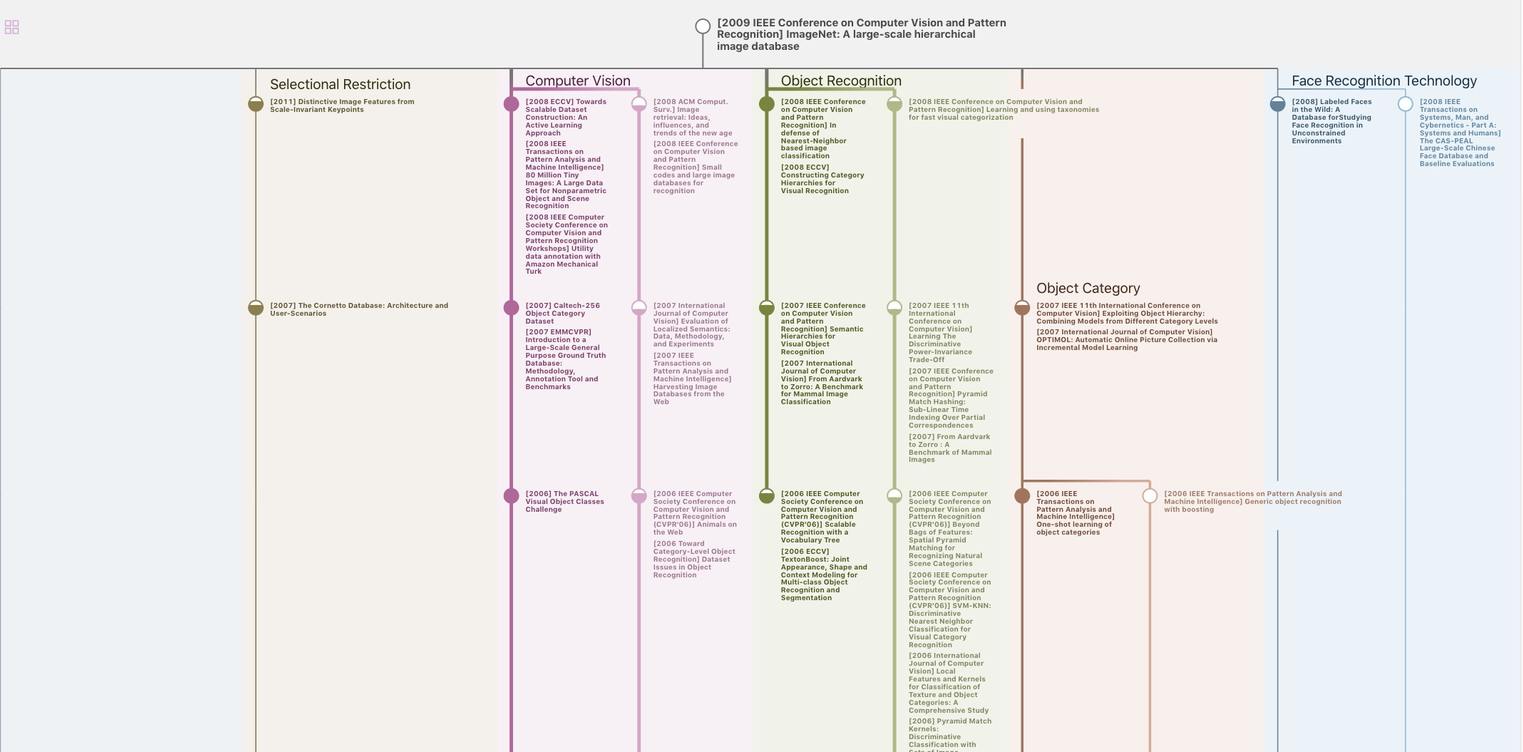
生成溯源树,研究论文发展脉络
Chat Paper
正在生成论文摘要