Protecting the Future: Neonatal Seizure Detection with Spatial-Temporal Modeling
CoRR(2023)
摘要
A timely detection of seizures for newborn infants with electroencephalogram (EEG) has been a common yet life-saving practice in the Neonatal Intensive Care Unit (NICU). However, it requires great human efforts for real-time monitoring, which calls for automated solutions to neonatal seizure detection. Moreover, the current automated methods focusing on adult epilepsy monitoring often fail due to (i) dynamic seizure onset location in human brains; (ii) different montages on neonates and (iii) huge distribution shift among different subjects. In this paper, we propose a deep learning framework, namely STATENet, to address the exclusive challenges with exquisite designs at the temporal, spatial and model levels. The experiments over the real-world large-scale neonatal EEG dataset illustrate that our framework achieves significantly better seizure detection performance.
更多查看译文
关键词
Seizure Detection,Spatial-temporal Model,Neonatal Seizure Detection,Deep Learning,Epilepsy,Neonatal Intensive Care Unit,Real-world Datasets,Neural Network,Signal Processing,Convolutional Neural Network,Spatial Relationship,Recurrent Neural Network,EEG Data,Final Prediction,EEG Signals,General Issues,Channel Signal,Graph Neural Networks,Aggregation Function,Multivariate Time Series,Temporal Convolutional Network,Graph Attention Network,Multilayer Perception,Seizure Events,Dilated Convolution,Wave Signal,Dilated Convolution Layers,Spatial Module,Machine Learning,Bipolar Montage
AI 理解论文
溯源树
样例
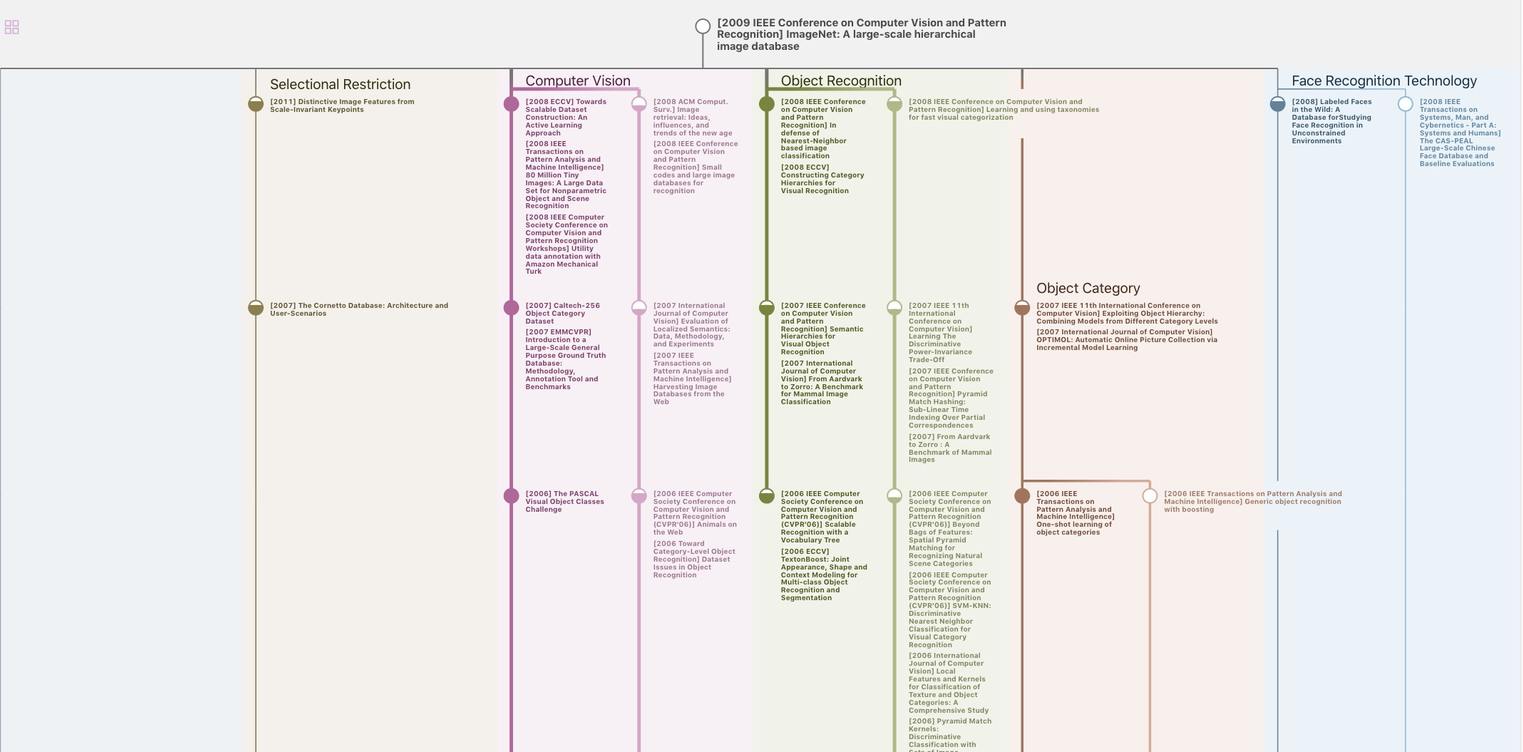
生成溯源树,研究论文发展脉络
Chat Paper
正在生成论文摘要