MinkSORT: A 3D deep feature extractor using sparse convolutions to improve 3D multi-object tracking in greenhouse tomato plants
BIOSYSTEMS ENGINEERING(2023)
摘要
The agro-food industry is turning to robots to address the challenge of labour shortage. However, agro-food environments pose difficulties for robots due to high variation and occlusions. In the presence of these chal-lenges, accurate world models, with information about object location, shape, and properties, are crucial for robots to perform tasks accurately. Building such models is challenging due to the complex and unique nature of agro-food environments, and errors in the model can lead to task execution issues. In this paper, MinkSORT, a novel method for generating tracking features using a 3D sparse convolutional network in a deepSORT-like approach, is proposed to improve the accuracy of world models in agro-food environments. MinkSORT was evaluated using real-world data collected in a tomato greenhouse, where it significantly improved the perfor-mance of a baseline model that tracks tomato positions in 3D using a Kalman filter and Mahalanobis distance. MinkSORT improved the HOTA from 42.8% to 44.77%, the association accuracy from 32.55% to 35.55%, and the MOTA from 57.63% to 58.81%. Different contrastive loss functions for training MinkSORT were also eval-uated, and it was demonstrated that it leads to improved performance in terms of three separate precision and recall detection outcomes. The proposed method improves world model accuracy, enabling robots to perform tasks such as harvesting and plant maintenance with greater efficiency and accuracy, which is essential for meeting the growing demand for food in a sustainable manner.
更多查看译文
关键词
World modelling,Deepsort,Robotics in agriculture,Deep learning,3D deep learning
AI 理解论文
溯源树
样例
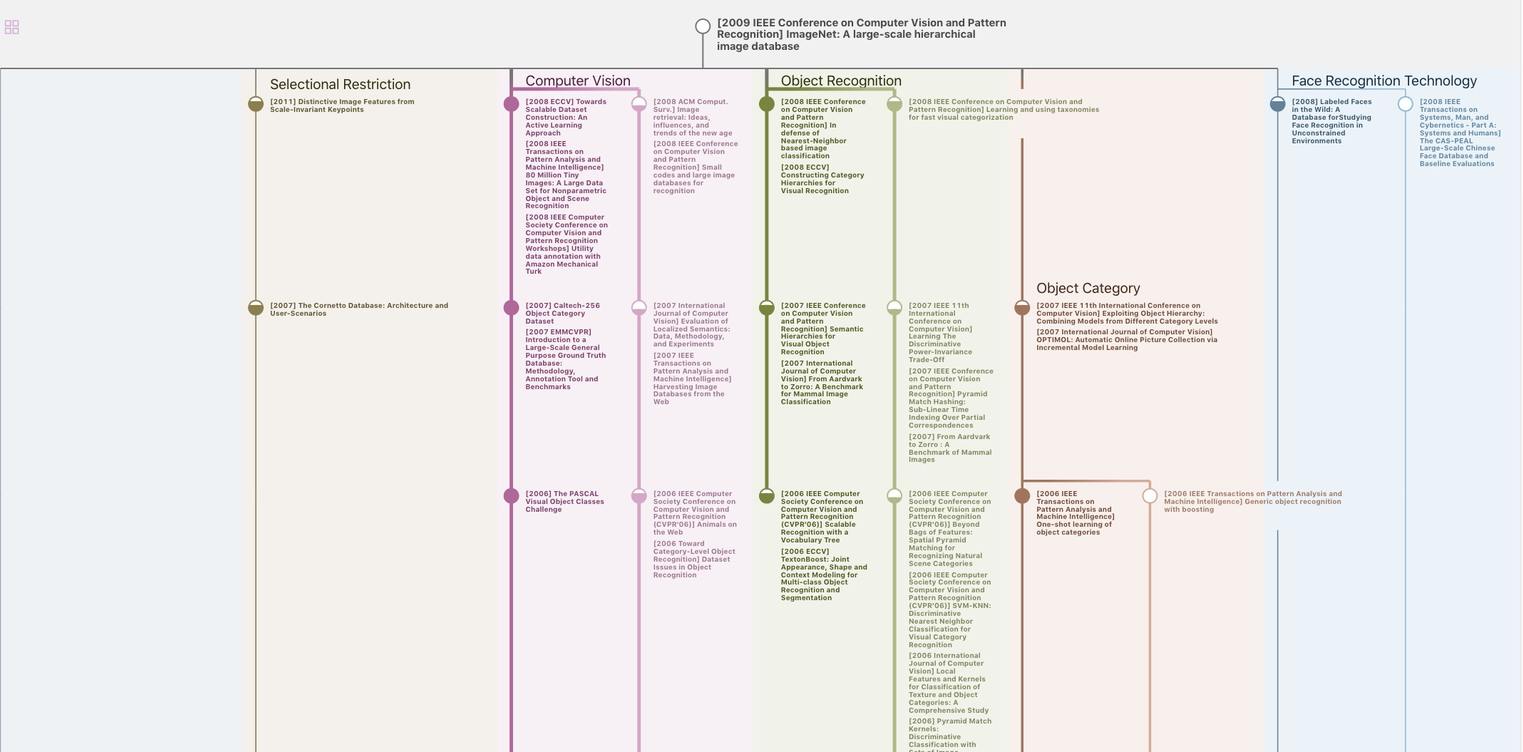
生成溯源树,研究论文发展脉络
Chat Paper
正在生成论文摘要