A Mapping Study of Machine Learning Methods for Remaining Useful Life Estimation of Lead-Acid Batteries
CoRR(2023)
摘要
Energy storage solutions play an increasingly important role in modern infrastructure and lead-acid batteries are among the most commonly used in the rechargeable category. Due to normal degradation over time, correctly determining the battery's State of Health (SoH) and Remaining Useful Life (RUL) contributes to enhancing predictive maintenance, reliability, and longevity of battery systems. Besides improving the cost savings, correct estimation of the SoH can lead to reduced pollution though reuse of retired batteries. This paper presents a mapping study of the state-of-the-art in machine learning methods for estimating the SoH and RUL of lead-acid batteries. These two indicators are critical in the battery management systems of electric vehicles, renewable energy systems, and other applications that rely heavily on this battery technology. In this study, we analyzed the types of machine learning algorithms employed for estimating SoH and RUL, and evaluated their performance in terms of accuracy and inference time. Additionally, this mapping identifies and analyzes the most commonly used combinations of sensors in specific applications, such as vehicular batteries. The mapping concludes by highlighting potential gaps and opportunities for future research, which lays the foundation for further advancements in the field.
更多查看译文
关键词
machine learning methods,lead-acid
AI 理解论文
溯源树
样例
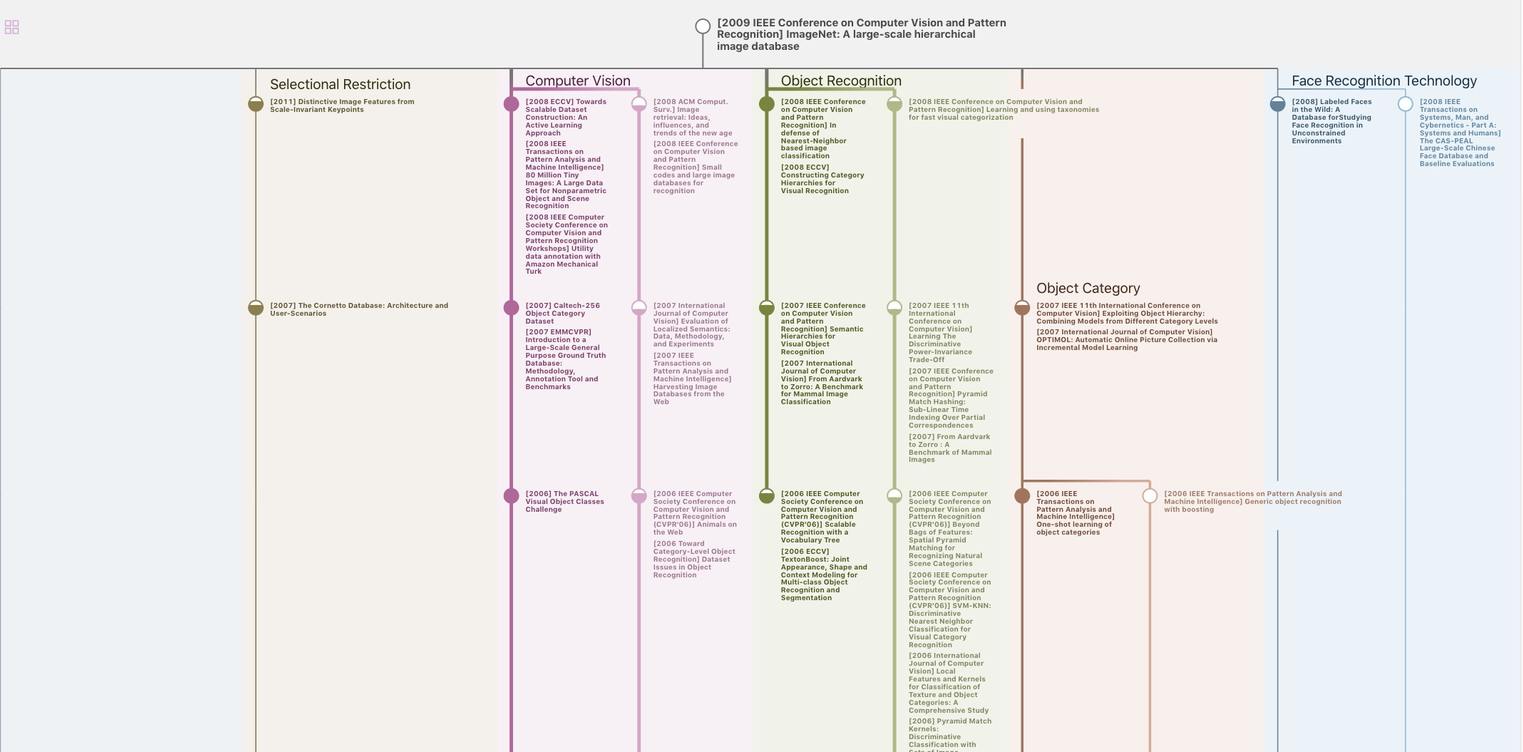
生成溯源树,研究论文发展脉络
Chat Paper
正在生成论文摘要