Benchmarking Algorithms for Federated Domain Generalization
ICLR 2024(2023)
摘要
While prior domain generalization (DG) benchmarks consider train-test dataset heterogeneity, we evaluate Federated DG which introduces federated learning (FL) specific challenges. Additionally, we explore domain-based heterogeneity in clients' local datasets - a realistic Federated DG scenario. Prior Federated DG evaluations are limited in terms of the number or heterogeneity of clients and dataset diversity. To address this gap, we propose an Federated DG benchmark methodology that enables control of the number and heterogeneity of clients and provides metrics for dataset difficulty. We then apply our methodology to evaluate 13 Federated DG methods, which include centralized DG methods adapted to the FL context, FL methods that handle client heterogeneity, and methods designed specifically for Federated DG. Our results suggest that despite some progress, there remain significant performance gaps in Federated DG particularly when evaluating with a large number of clients, high client heterogeneity, or more realistic datasets. Please check our extendable benchmark code here: https://github.com/inouye-lab/FedDG_Benchmark.
更多查看译文
关键词
federated learning,distributed learning,domain generalization,out-of-distribution generalization,benchmarking,data paritioning.
AI 理解论文
溯源树
样例
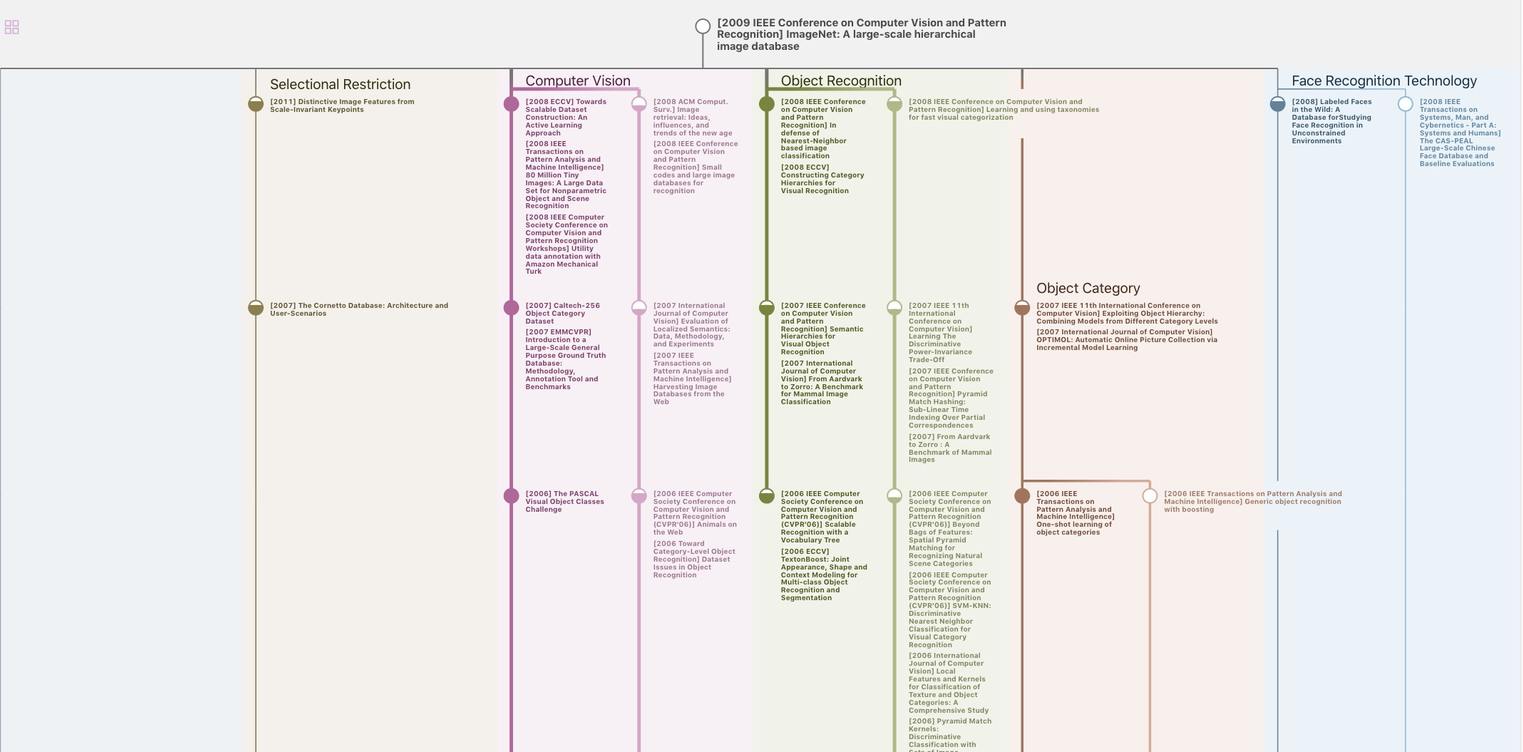
生成溯源树,研究论文发展脉络
Chat Paper
正在生成论文摘要