Weakly-Supervised Positional Contrastive Learning: Application to Cirrhosis Classification
MEDICAL IMAGE COMPUTING AND COMPUTER ASSISTED INTERVENTION, MICCAI 2023, PT I(2023)
摘要
n Large medical imaging datasets can be cheaply and quickly annotated with low-confidence, weak labels (e.g., radiological scores). Access to high-confidence labels, such as histology-based diagnoses, is rare and costly. Pretraining strategies, like contrastive learning (CL) methods, can leverage unlabeled or weakly-annotated datasets. These methods typically require large batch sizes, which poses a difficulty in the case of large 3D images at full resolution, due to limited GPU memory. Nevertheless, volumetric positional information about the spatial context of each 2D slice can be very important for some medical applications. In this work, we propose an efficient weakly-supervised positional (WSP) contrastive learning strategy where we integrate both the spatial context of each 2D slice and a weak label via a generic kernel-based loss function. We illustrate our method on cirrhosis prediction using a large volume of weakly-labeled images, namely radiological low-confidence annotations, and small strongly-labeled (i.e., high-confidence) datasets. The proposed model improves the classification AUC by 5% with respect to a baseline model on our internal dataset, and by 26% on the public LIHC dataset from the Cancer Genome Atlas. The code is available at: https://github.com/Guerbet-AI/wsp-contrastive.
更多查看译文
关键词
Weakly-supervised learning,Contrastive learning,CT,Cirrhosis prediction,Liver
AI 理解论文
溯源树
样例
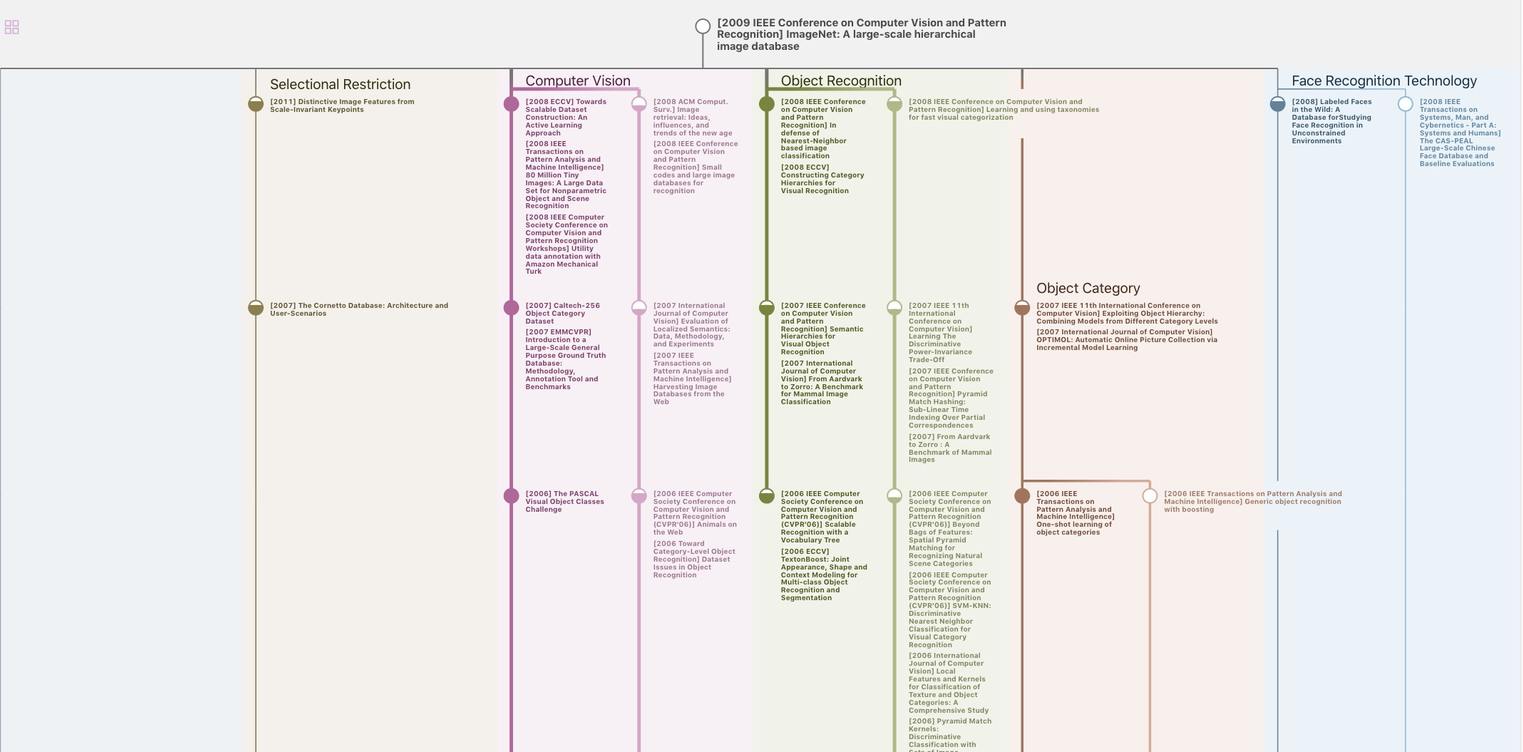
生成溯源树,研究论文发展脉络
Chat Paper
正在生成论文摘要