An Examination of Wearable Sensors and Video Data Capture for Human Exercise Classification
MACHINE LEARNING AND KNOWLEDGE DISCOVERY IN DATABASES: APPLIED DATA SCIENCE AND DEMO TRACK, ECML PKDD 2023, PT VI(2023)
摘要
Wearable sensors such as Inertial Measurement Units (IMUs) are often used to assess the performance of human exercise. Common approaches use handcrafted features based on domain expertise or automatically extracted features using time series analysis. Multiple sensors are required to achieve high classification accuracy, which is not very practical. These sensors require calibration and synchronization and may lead to discomfort over longer time periods. Recent work utilizing computer vision techniques has shown similar performance using video, without the need for manual feature engineering, and avoiding some pitfalls such as sensor calibration and placement on the body. In this paper, we compare the performance of IMUs to a video-based approach for human exercise classification on two real-world datasets consisting of Military Press and Rowing exercises. We compare the performance using a single camera that captures video in the frontal view versus using 5 IMUs placed on different parts of the body. We observe that an approach based on a single camera can outperform a single IMU by 10 percentage points on average. Additionally, a minimum of 3 IMUs are required to outperform a single camera. We observe that working with the raw data using multivariate time series classifiers outperforms traditional approaches based on handcrafted or automatically extracted features. Finally, we show that an ensemble model combining the data from a single camera with a single IMU outperforms either data modality. Our work opens up new and more realistic avenues for this application, where a video captured using a readily available smartphone camera, combined with a single sensor, can be used for effective human exercise classification.
更多查看译文
关键词
Exercise Classification,Inertial Sensors,Video,Time Series Classification,Real-World Datasets
AI 理解论文
溯源树
样例
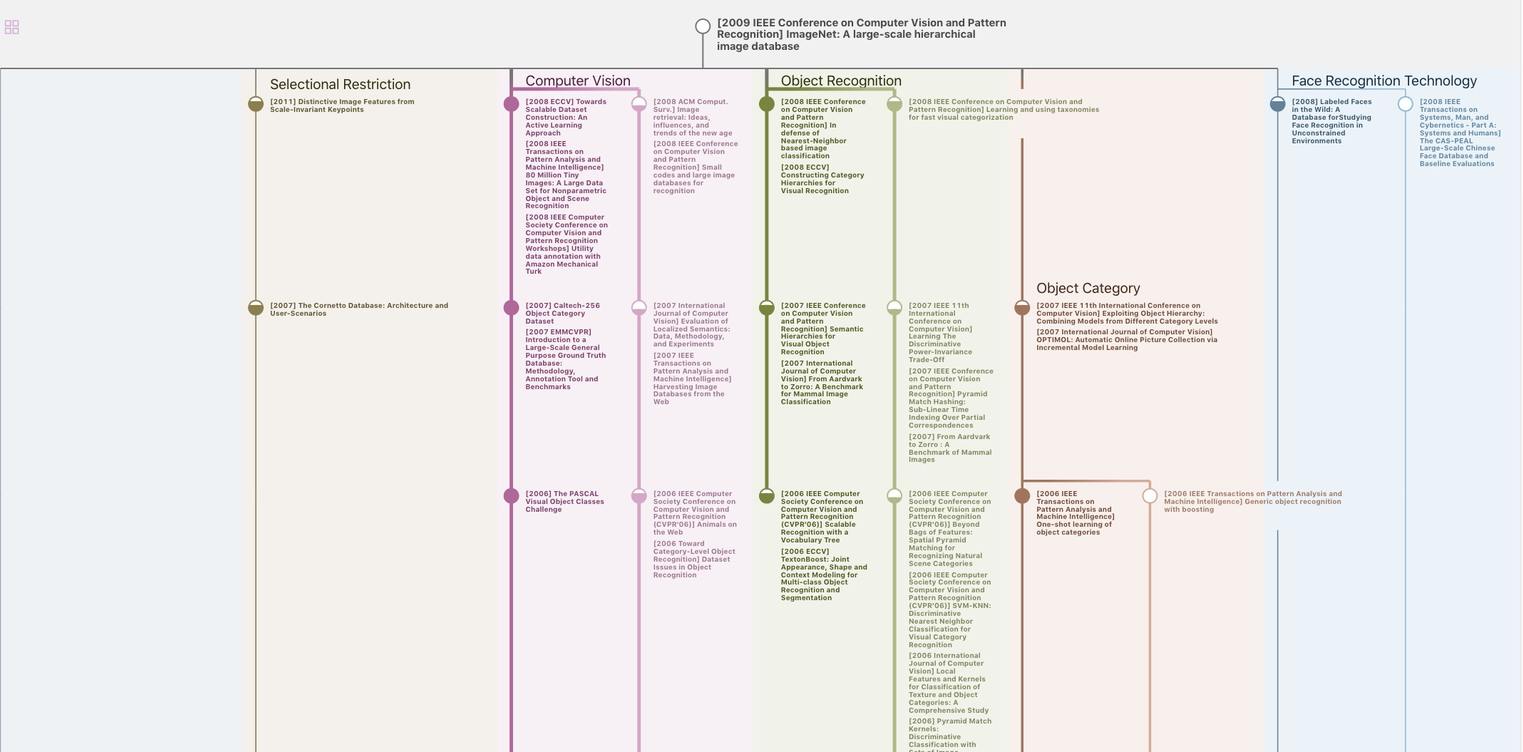
生成溯源树,研究论文发展脉络
Chat Paper
正在生成论文摘要