FILM: How can Few-Shot Image Classification Benefit from Pre-Trained Language Models?
CoRR(2023)
摘要
Few-shot learning aims to train models that can be generalized to novel classes with only a few samples. Recently, a line of works are proposed to enhance few-shot learning with accessible semantic information from class names. However, these works focus on improving existing modules such as visual prototypes and feature extractors of the standard few-shot learning framework. This limits the full potential use of semantic information. In this paper, we propose a novel few-shot learning framework that uses pre-trained language models based on contrastive learning. To address the challenge of alignment between visual features and textual embeddings obtained from text-based pre-trained language model, we carefully design the textual branch of our framework and introduce a metric module to generalize the cosine similarity. For better transferability, we let the metric module adapt to different few-shot tasks and adopt MAML to train the model via bi-level optimization. Moreover, we conduct extensive experiments on multiple benchmarks to demonstrate the effectiveness of our method.
更多查看译文
关键词
language models,classification,few-shot,pre-trained
AI 理解论文
溯源树
样例
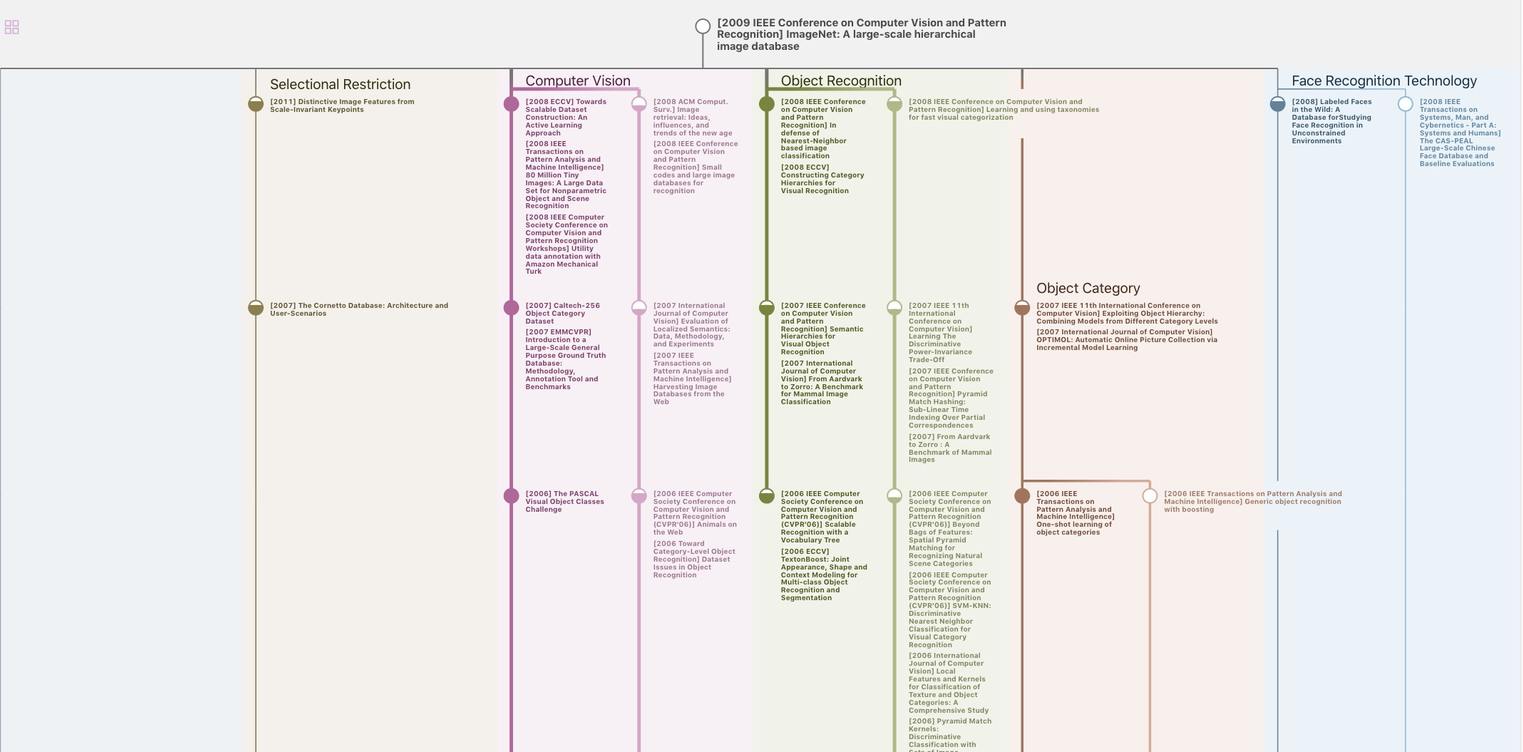
生成溯源树,研究论文发展脉络
Chat Paper
正在生成论文摘要