Unsupervised 3D Out-of-Distribution Detection with Latent Diffusion Models
MEDICAL IMAGE COMPUTING AND COMPUTER ASSISTED INTERVENTION, MICCAI 2023, PT I(2023)
摘要
Methods for out-of-distribution (OOD) detection that scale to 3D data are crucial components of any real-world clinical deep learning system. Classic denoising diffusion probabilistic models (DDPMs) have been recently proposed as a robust way to perform reconstruction-based OOD detection on 2D datasets, but do not trivially scale to 3D data. In this work, we propose to use Latent Diffusion Models (LDMs), which enable the scaling of DDPMs to high-resolution 3D medical data. We validate the proposed approach on near- and far-OOD datasets and compare it to a recently proposed, 3D-enabled approach using Latent Transformer Models (LTMs). Not only does the proposed LDM-based approach achieve statistically significant better performance, it also shows less sensitivity to the underlying latent representation, more favourable memory scaling, and produces better spatial anomaly maps. Code is available at https://github.com/marksgraham/ddpm-ood.
更多查看译文
关键词
Latent diffusion models,Out-of-distribution detection
AI 理解论文
溯源树
样例
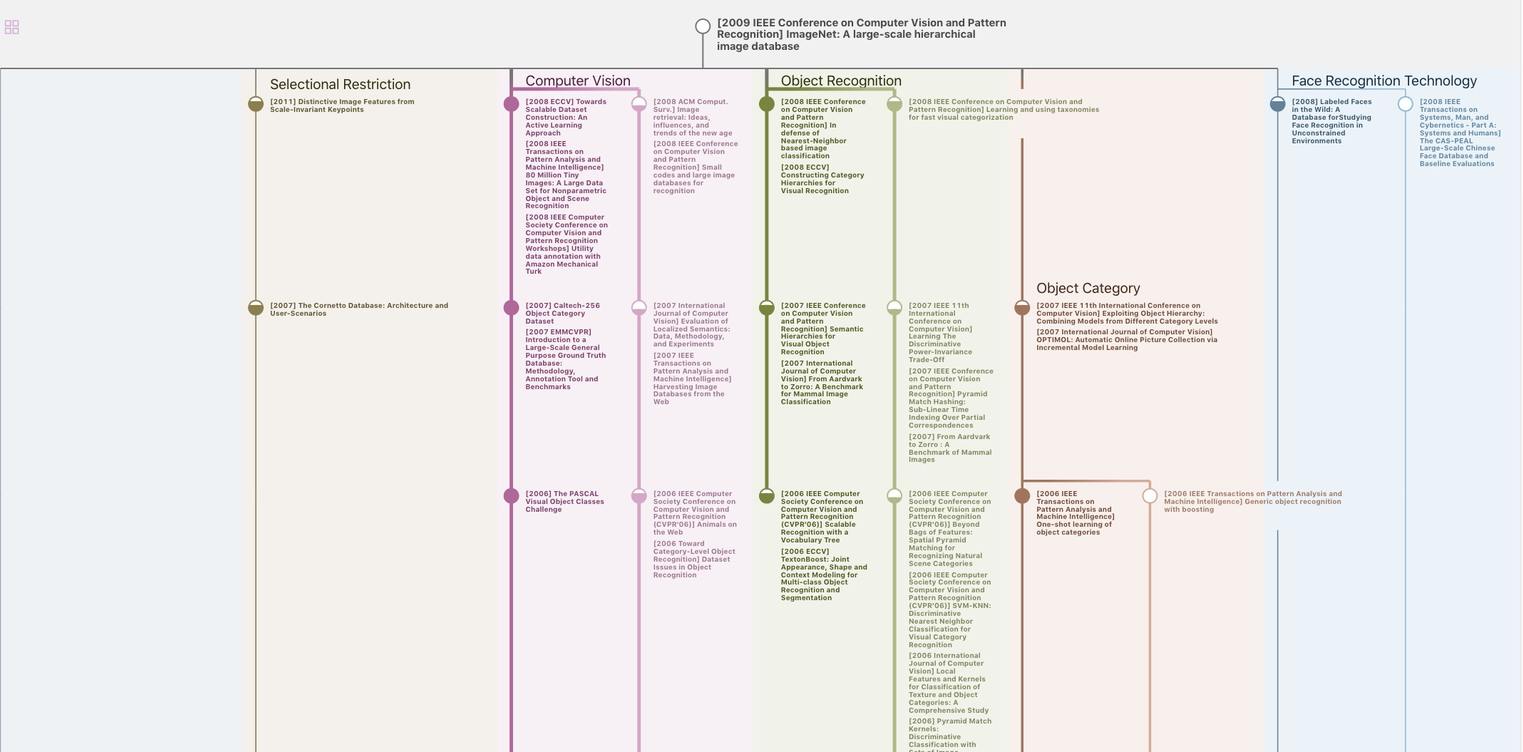
生成溯源树,研究论文发展脉络
Chat Paper
正在生成论文摘要