Multi-task Deep Neural Network for Air Pollution Prediction and Spatial Effect Analysis
2023 8th International Conference on Cloud Computing and Big Data Analytics (ICCCBDA)(2023)
摘要
The deterioration of air quality is a crucial issue because of the negative effect it brings to human health and environment. Many data-driven models have been established to predict air pollution of one single location from its local weather and pollutant factors. However, an existing challenge is to develop a single model that can simultaneously predict air pollution of multiple locations and investigate the spatial effect. In response to this problem, this paper proposes a multi-task deep neural network (MT-DNN) model. The proposed model can identify the shared key factors that affect air pollution of multiple locations, build a shared model structure with these factors, and produce predictions for multiple locations. The proposed model is applied to predict PM2.5 of six locations in Beijing area. The experiment results show that high prediction accuracies have been achieved by the model. More importantly, the model reveals the spatiotemporal correlations between factors of multiple locations, and how these factors interact with each other. These findings present a new framework for time series prediction problem with spatial effect analysis.
更多查看译文
关键词
Air pollution, time series prediction, feature selection, neural networks, multi-task model
AI 理解论文
溯源树
样例
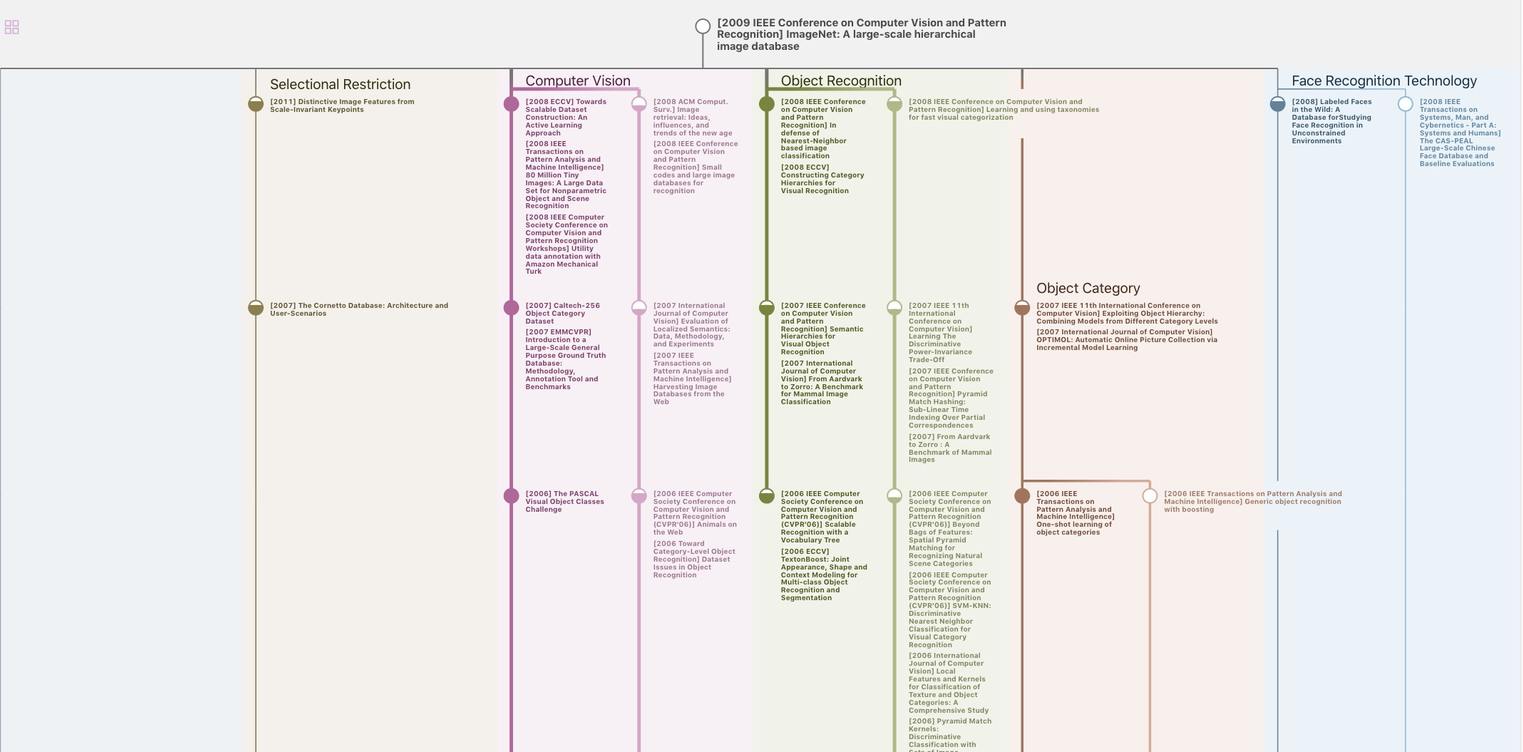
生成溯源树,研究论文发展脉络
Chat Paper
正在生成论文摘要