Electricity Theft Detection Via Deep Learning
2023 International Conference on Communication Technologies (ComTech)(2023)
摘要
Electricity theft is a major problem facing many countries around the world. Its adverse effects include loss in revenue for power distribution companies and the government economy, the distribution quality of electricity, increased generation load, and high electricity cost which affects honest consumers as well. With the advancement in smart meters in electricity infrastructure, massive data is generated that can be analyzed for electricity consumers’ consumption patterns. With the help of these consumption patterns of consumers, several machine learning and deep learning models and techniques are built to detect fraudulent consumers. With the help of detection of the theft location and fraud consumers, energy distribution companies can impose fines on these consumers and reduce revenue losses. In this research, a combination of CNN and STN based model is proposed to detect electricity theft. STN is used in many image classification problems along with CNN to enhance the performance of the models. STN along with CNN is used for the first time in electricity theft detection. STN is used to translate, rotate and scale the original input. The dataset used in this research is real customers’ electricity usage data publicly provided by the State Grid Corporation of China (SGCC). SGCC dataset has missing values and also has class imbalance problems. The number of theft users is significantly lower than the number of honest consumers, which is addressed by using the Synthetic Minority Oversampling Technique (SMOTE). State-of-the-art machine learning and deep learning models are compared to the proposed model, and the findings reveal that the suggested model can recognize theft and regular users more accurately.
更多查看译文
关键词
Electricity theft detection,CNN,STN
AI 理解论文
溯源树
样例
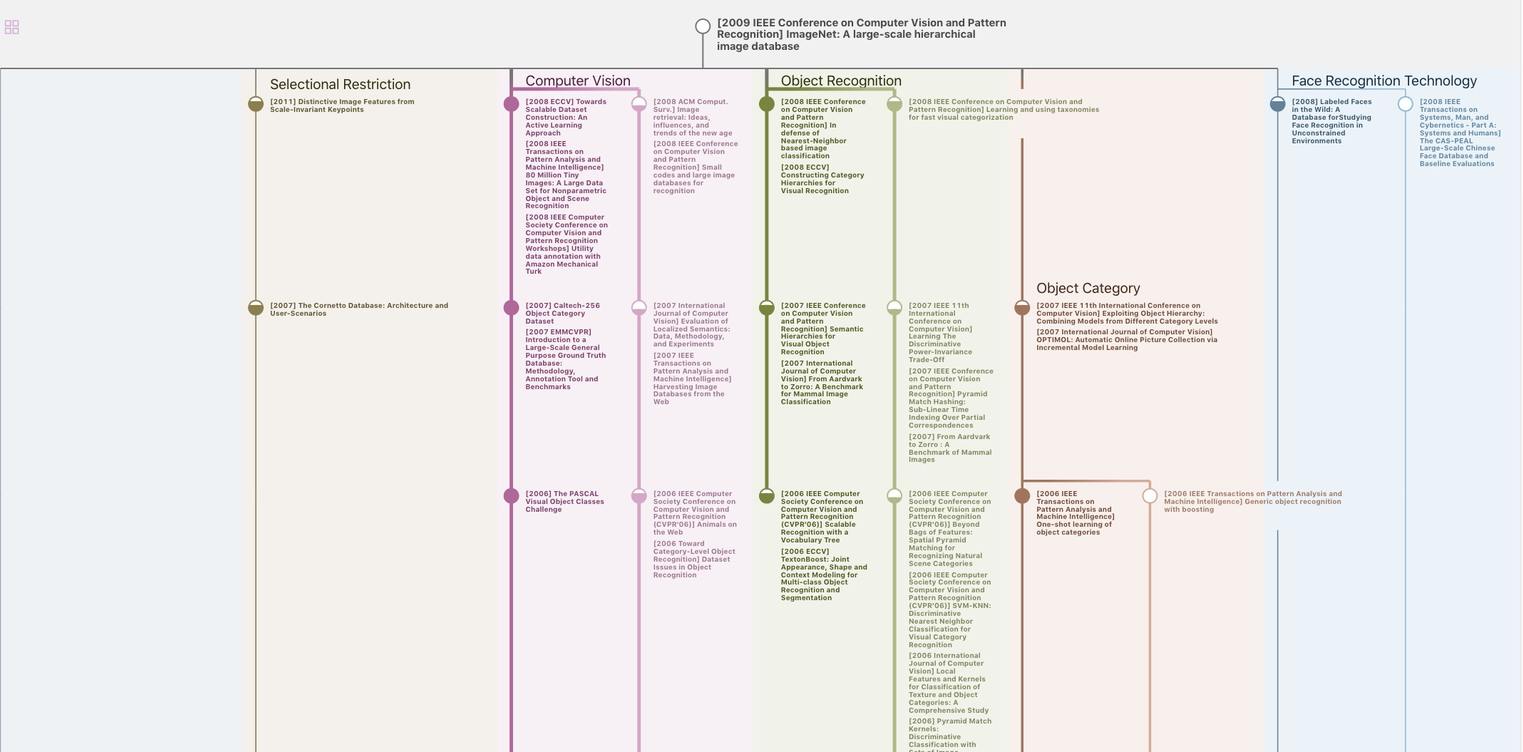
生成溯源树,研究论文发展脉络
Chat Paper
正在生成论文摘要