When Fair Classification Meets Noisy Protected Attributes
PROCEEDINGS OF THE 2023 AAAI/ACM CONFERENCE ON AI, ETHICS, AND SOCIETY, AIES 2023(2023)
摘要
The operationalization of algorithmic fairness comes with several practical challenges, not the least of which is the availability or reliability of protected attributes in datasets. In real-world contexts, practical and legal impediments may prevent the collection and use of demographic data, making it difficult to ensure algorithmic fairness. While initial fairness algorithms did not consider these limitations, recent proposals aim to achieve algorithmic fairness in classification by incorporating noisiness in protected attributes or not using protected attributes at all. To the best of our knowledge, this is the first head-to-head study of fair classification algorithms to compare attribute-reliant, noise-tolerant and attribute-unaware algorithms along the dual axes of predictivity and fairness. We evaluated these algorithms via case studies on four real-world datasets and synthetic perturbations. Our study reveals that attribute-unaware and noise-tolerant fair classifiers can potentially achieve similar level of performance as attribute-reliant algorithms, even when protected attributes are noisy. However, implementing them in practice requires careful nuance. Our study provides insights into the practical implications of using fair classification algorithms in scenarios where protected attributes are noisy or partially available.
更多查看译文
关键词
fairness,classification,protected attributes,evaluation
AI 理解论文
溯源树
样例
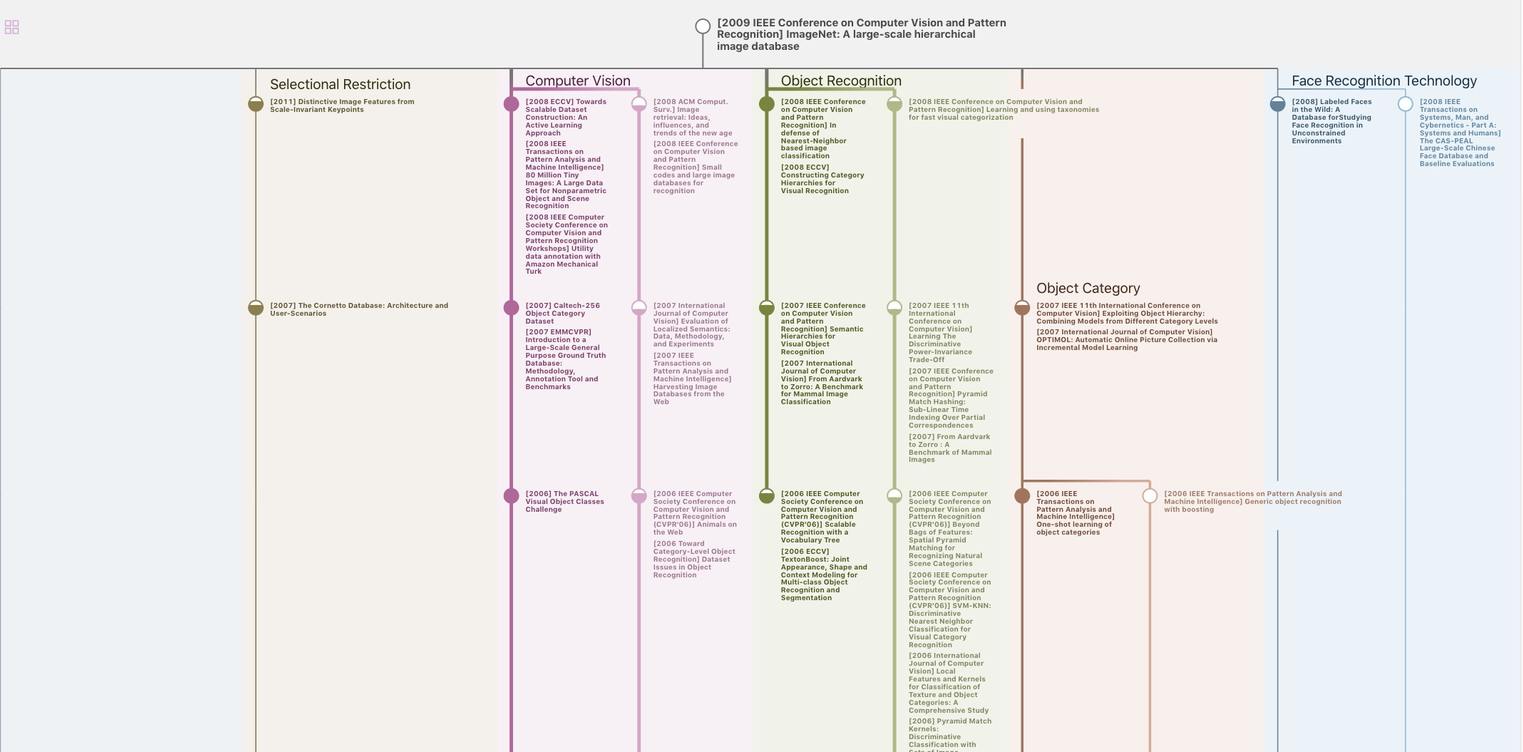
生成溯源树,研究论文发展脉络
Chat Paper
正在生成论文摘要