High-fidelity GAN inversion by frequency domain guidance
Computers & Graphics(2023)
摘要
Generative Adversarial Network (GAN) inversion aims to invert the input image into the latent space of a pre-trained GAN to obtain a latent code, and then apply it to downstream visual tasks. Several studies have shown that deep neural networks suffer from spectral bias, making it difficult for the generative network to learn the high-frequency distribution of images, and thus lead to poor high-frequency detail in the generated images. To overcome the aforementioned limitations, we propose a high-frequency information generation guidance branch to achieve more fidelity image reconstruction. Specifically, we first utilize the frequency domain gap between the initial reconstructed image and the original image, and then apply them to guide the generator to reconstruct the high-frequency part of the hard-to-recover image. In this manner, the high-frequency information content of the image can be better reconstructed. In addition, to learn the high-frequency information of the image that is not learned previously more quickly, we invoke focal frequency loss to accelerate the learning process of the network. Extensive experimental results on several benchmark datasets demonstrate that our method outperforms the state-of-the-art, while the network can converge faster.
更多查看译文
关键词
GAN inversion,Frequency domain,Image reconstruction
AI 理解论文
溯源树
样例
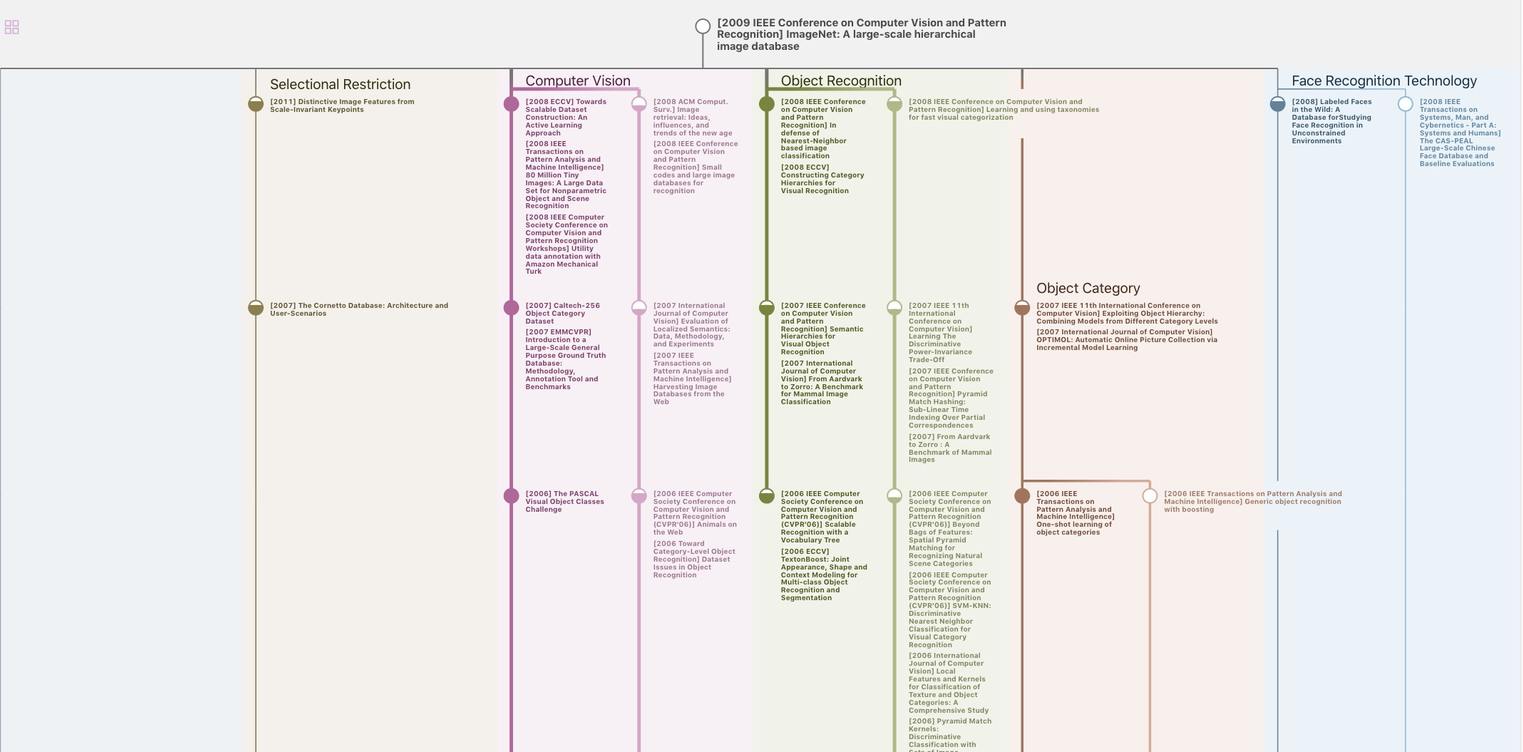
生成溯源树,研究论文发展脉络
Chat Paper
正在生成论文摘要