Improving building inventory with a machine learning approach: application in southern Italy
Procedia Structural Integrity(2023)
摘要
The compiling of large-scale building inventory is a fundamental step in the evaluation of seismic risk in a region of interest. For classification of buildings in vulnerability classes, different levels of detail could be employed depending on the model adopted for the vulnerability assessment. Generally, the key structural characteristics of exposed building needed for the application of more refined vulnerability models are not easily available. Aimed to improve the information quality relevant to exposure, the interview-based form Cartis was recently implemented by ReLUIS under supervision of Italian Civil Protection Department within “Territorial Themes” DPC-ReLUIS project. Being based on an interview protocol, this form allows to rapidly detect relevant buildings data at urban level. Despite Cartis is an extremely useful tool for the compiling of regional scale inventories, nowadays the database covers only the 5% of Italian territory. This study aims to exploit the actual data available in Cartis database to build an exposure model that could be applied for preliminary inventory evaluation also in towns where the Cartis form is not compiled yet. To this end, a machine learning approach is applied. Cartis data available for several municipalities in Campania region are used together with basic information on buildings and population provided by Italian census data (ISTAT) to train a supervised learning algorithm for identification of homogenous urban sectors in terms of buildings features (i.e., Town Compartments) in municipal area. Once the algorithm is implemented, it may be employed in other municipalities to delimit relative Town Compartments and to identify most common building typologies within them.
更多查看译文
关键词
Cartis,Exposure modelling,Machine learning
AI 理解论文
溯源树
样例
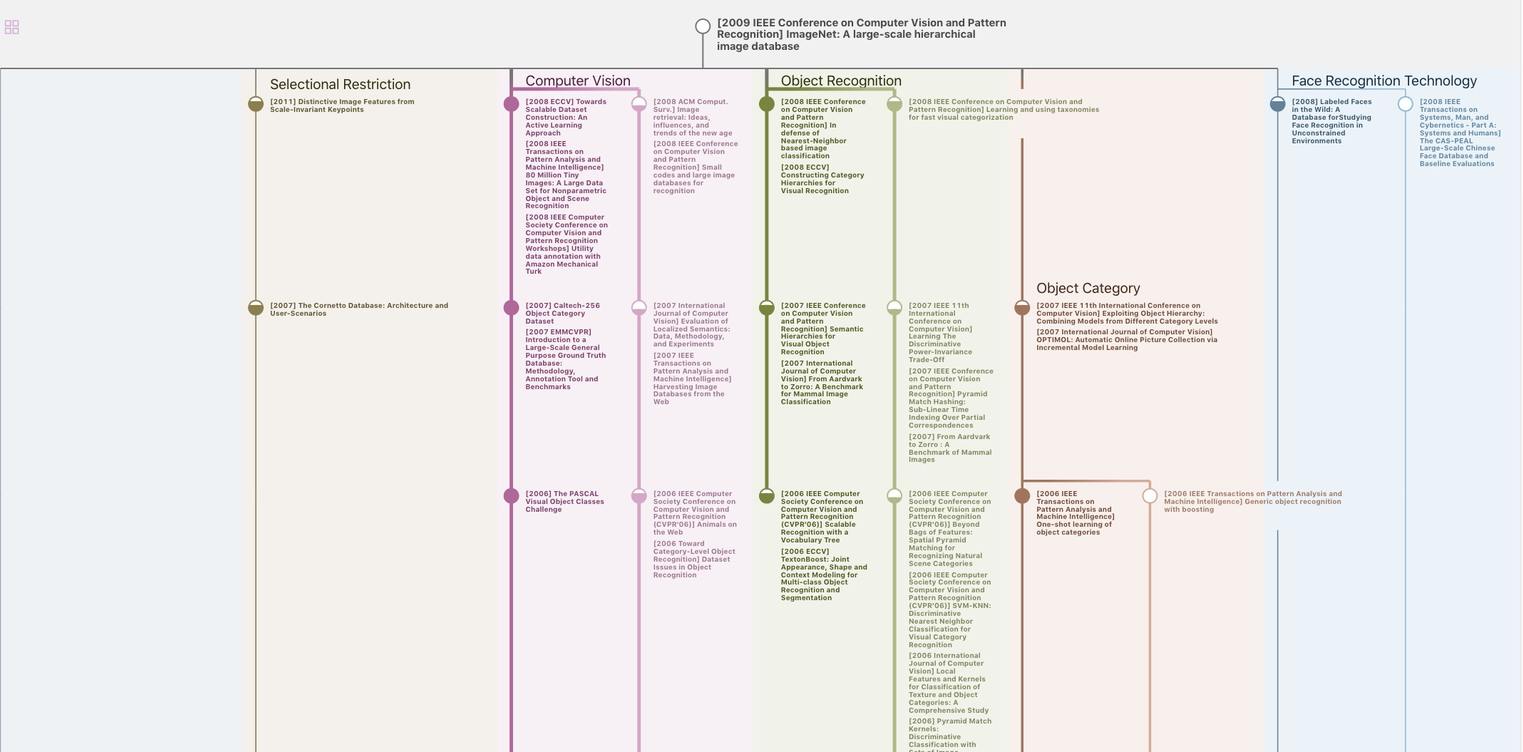
生成溯源树,研究论文发展脉络
Chat Paper
正在生成论文摘要