Resource-efficient machining through physics-informed machine learning
Procedia CIRP(2023)
摘要
Resource-efficient machining has been at the forefront of sustainable manufacturing for many years. However, the total life of the cutting tool is often not fully exploited to avoid the severe consequences of excessive wear. This conservative strategy increases production time, cost per part, and the carbon footprint of the machining process. The present contribution focuses on resource-efficient machining through physics-informed machine learning, exploiting the benefits of both physics-based and data-driven modelling to accurately predict cutting tool life. The results demonstrate the utility and superiority of this hybrid approach relative to traditional methods and the recent trends towards purely data-driven techniques.
更多查看译文
关键词
cutting tool life prediction,physics-informed machine learning,grey-box modelling,process optimisation
AI 理解论文
溯源树
样例
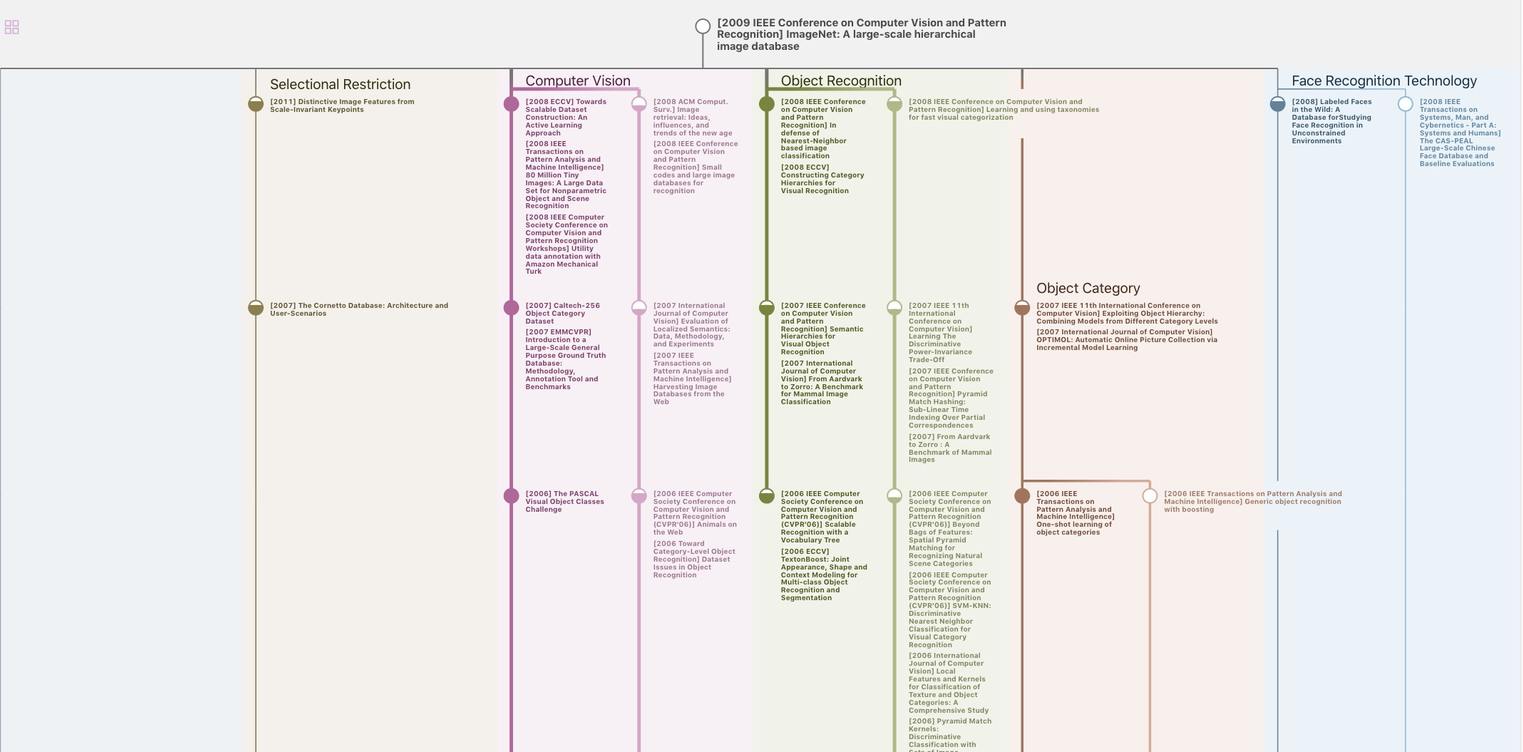
生成溯源树,研究论文发展脉络
Chat Paper
正在生成论文摘要