Signal area estimation based on deep learning
Physical Communication(2023)
摘要
In many practical application scenarios, radio communication signals are commonly represented as a spectrogram, which represents the signal strength measured at multiple discrete time instants and frequency points within a specific time interval and frequency band, respectively. In the context of spectrum occupancy measurements, the notion of Signal Area (SA) is defined as the rectangular region in the time–frequency domain where a signal is assumed to be present. Signal Area Estimation (SAE) is an important functionality in spectrum-aware wireless systems where spectrum usage monitoring is required. However, the conventional approaches to SAE have a limited estimation accuracy, in particular at low SNR. In this work, a novel technique for SAE is proposed using Deep Learning based on Artificial Neural Network (DL-ANN) for enhanced extraction of SA information from radio spectrograms. The performance of the proposed DL-ANN method is evaluated both with software simulations and hardware experiments, and the results are compared with several conventional methods from the literature, showing significant performance improvements. A key feature of the proposed method is the improvement in the SAE accuracy compared to other existing methods (in particular in the low SNR regime) and the capability to extract the location of the detected SAs automatically. Overall, the proposed technique is a promising solution for the automatic processing of radio spectrograms in spectrum-aware wireless systems.
更多查看译文
关键词
Spectrum awareness,Signal area estimation,Deep learning,Artificial neural network
AI 理解论文
溯源树
样例
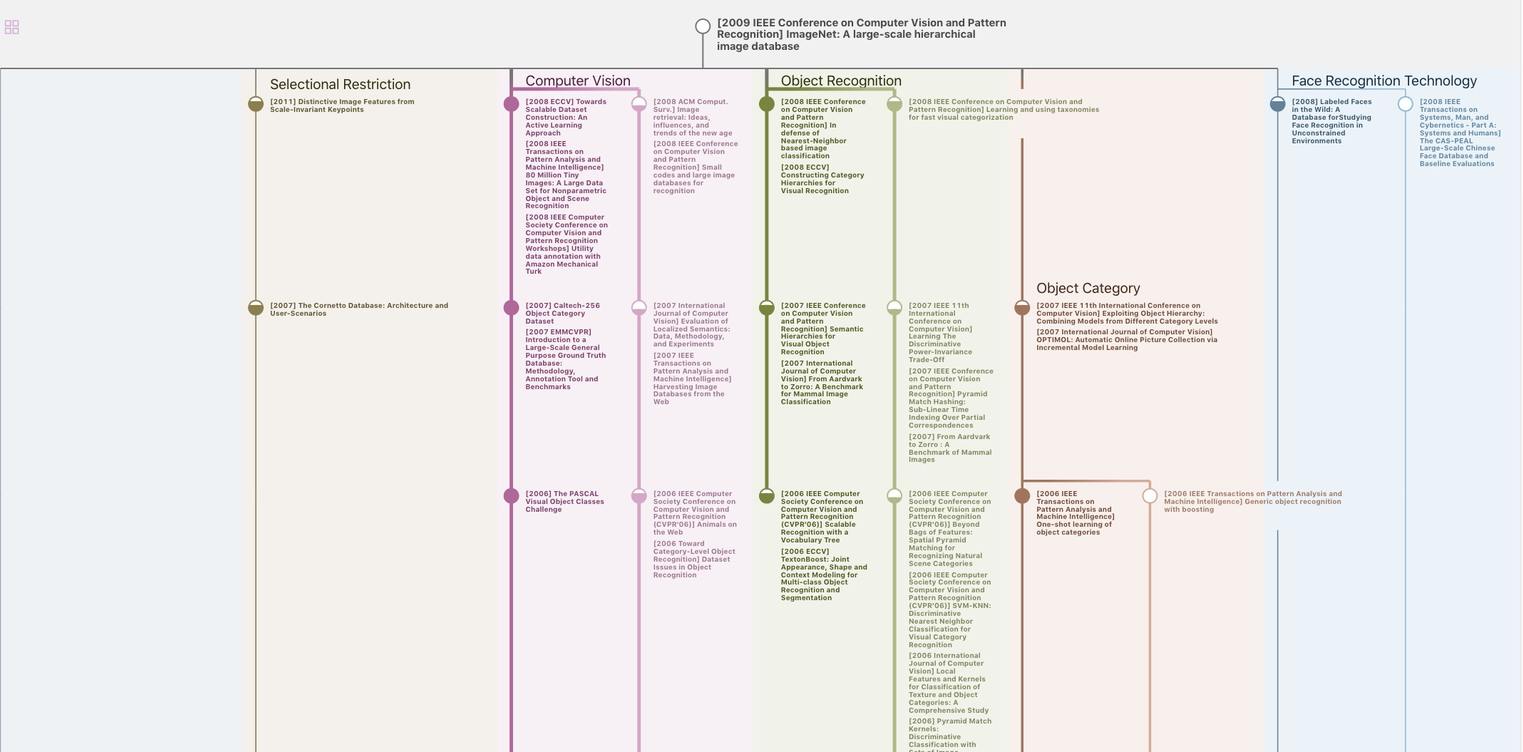
生成溯源树,研究论文发展脉络
Chat Paper
正在生成论文摘要