Few-shot working condition recognition of a sucker-rod pumping system based on a 4-dimensional time-frequency signature and meta-learning convolutional shrinkage neural network
Petroleum Science(2023)
摘要
The accurate and intelligent identification of the working conditions of a sucker-rod pumping system is necessary. As onshore oil extraction gradually enters its mid-to late-stage, the cost required to train a deep learning working condition recognition model for pumping wells by obtaining enough new working condition samples is expensive. For the few-shot problem and large calculation issues of new working conditions of oil wells, a working condition recognition method for pumping unit wells based on a 4-dimensional time-frequency signature (4D-TFS) and meta-learning convolutional shrinkage neural network (ML-CSNN) is proposed. First, the measured pumping unit well workup data are converted into 4D-TFS data, and the initial feature extraction task is performed while compressing the data. Subsequently, a convolutional shrinkage neural network (CSNN) with a specific structure that can ablate low-frequency features is designed to extract working conditions features. Finally, a meta-learning fine-tuning framework for learning the network parameters that are susceptible to task changes is merged into the CSNN to solve the few-shot issue. The results of the experiments demonstrate that the trained ML-CSNN has good recognition accuracy and generalization ability for few-shot working condition recognition. More specifically, in the case of lower computational complexity, only few-shot samples are needed to fine-tune the network parameters, and the model can be quickly adapted to new classes of well conditions.
更多查看译文
关键词
convolutional shrinkage,neural network,recognition,few-shot,sucker-rod,time-frequency,meta-learning
AI 理解论文
溯源树
样例
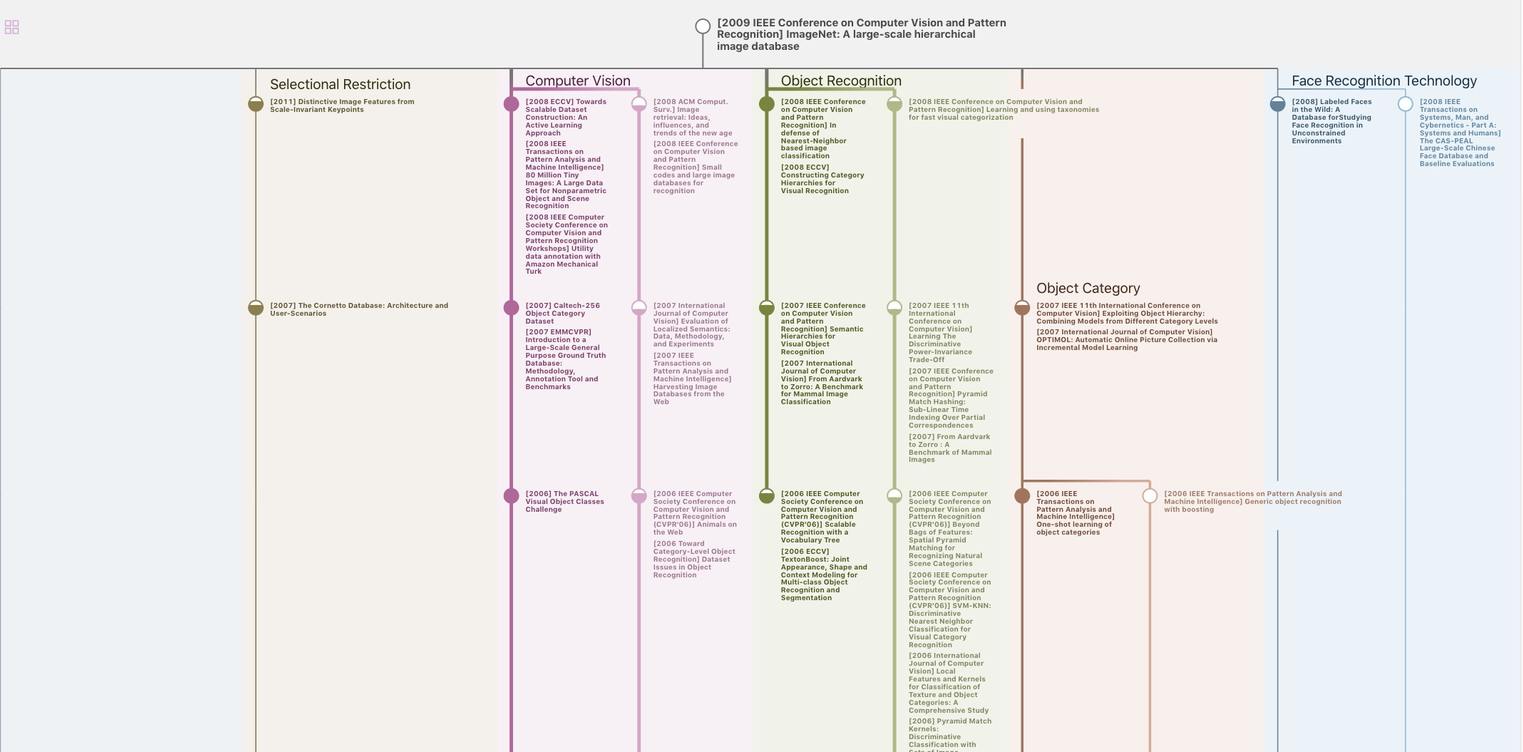
生成溯源树,研究论文发展脉络
Chat Paper
正在生成论文摘要