Deep learning approach to measure cartilage volume: data from the osteoarthritis initiative
Osteoarthritis Imaging(2023)
摘要
Change in cartilage volume on Magnetic Resonance Imaging (MRI) has been suggested as a potential surrogate endpoint for knee osteoarthritis (KOA) in clinical trials. We have developed the semi-automated Local-Area Cartilage Segmentation (LACS-SA) software, to measure cartilage volume in central weight-bearing areas of the MF, LF, MT, and LT. Though currently fast (requiring approximately 40 minutes per scan) improvements to the efficiency will be necessary to handle the workload for very large studies. Software segmentation methods are generally validated using metrics that directly compare the regions of interest such as the Dice similarity coefficient (DSC). However for cartilage, this approach may not capture the subtle changes that account for KOA-related loss where even a single voxel change in thickness can be substantial. The goal of our current work was to use a highly powered study to evaluate a deep learning (DL) version (LACS-DL) that can be used as a valid and more automated replacement by demonstrating a high correlation and equivalent responsiveness to change, 700 participants from the OAI) with KL scores of 1, 2, or 3 at baseline (BL) had MRI scans acquired at the BL, 24 mo., 48 mo., 72 mo., and 96 mo. time points (i.e., a total of 3,095 MRI scans). Cartilage volumes in the MF, LF, MT, and LT were measured using LACS-SA on the sagittal 3D double-echo steady-state (DESS) sequence. To develop LACS-DL, the 3,095 scans were segmented using the LACS_SA and used to train a U-Net convolutional neural network (CNN). The data were divided into two sets (Set 1 and Set 2) with a 50% split by subject ID. Set 1 was used to train the CNN for testing on Set 2, and Set 2 was used to train for testing on Set 1. We measured the coefficient of variation (CoV), and correlation (Pearson's R2) between BL cartilage volumes calculated with LACS-SA and LACS-DL. To evaluate the performance of LACS-DL for use in clinical trials, we calculated the responsiveness to change between BL and the other time points using standardized response mean (SRM) values. The BL KL grade distribution was KL1 23%, KL2 48%, and KL3 29%, and the participants were 59% female, had a mean (SD) age of 61.3 (8.9) years, and mean (SD) BMI of 29.0 (4.6) kg/m2. The R2 and CoV values ranged from 0.89 to 0.95 and 5.0% to 7.3% respectively. The reader time for LACS-DL is approximately 2 minutes/scan, or about 5% of the time needed for LACS-SA. Table 1 provides the SRM values for LACS-SA and LACS-DL. The data suggest that the DL approach has similar responsiveness. The average DSC was 0.90. The results offer compelling evidence that LACS-DL is a valid substitute for LACS-SA both through concurrent validity and responsiveness to change. The 20-fold improvement in reader time and equivalent performance to LACS-SA suggest that LACS-DL could be utilized in large longitudinal studies and clinical trials of KOA.
更多查看译文
关键词
measure cartilage volume,osteoarthritis initiative,deep learning,deep learning approach
AI 理解论文
溯源树
样例
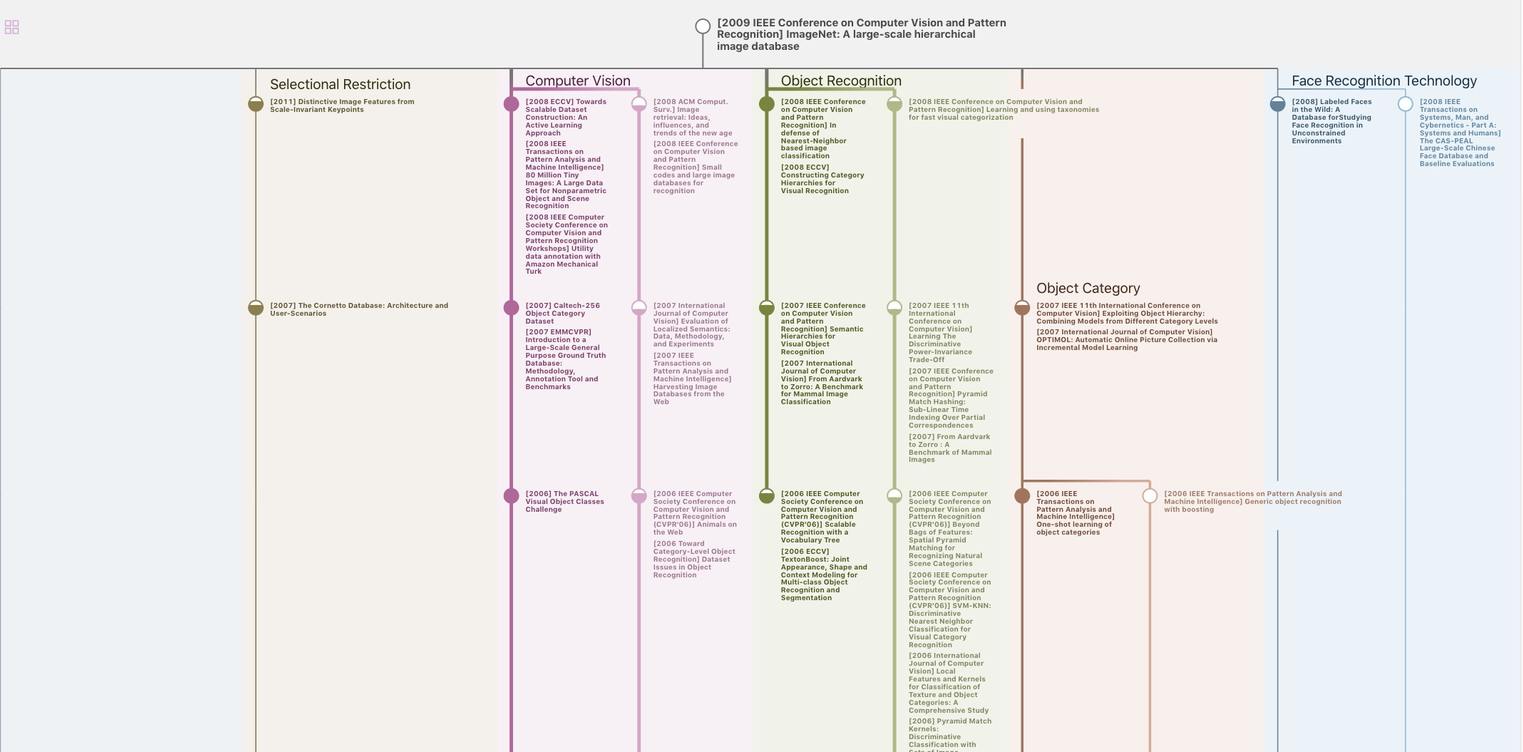
生成溯源树,研究论文发展脉络
Chat Paper
正在生成论文摘要