Deep learning for automatic segmentation of clinical photographs of oral premalignant and malignant lesions
Oral Surgery, Oral Medicine, Oral Pathology and Oral Radiology(2023)
摘要
Objective To explore the usage of Deep Learning (DL) induced models for automatic segmentation of premalignant and incipient malignant lesions on photographic images. Study Design A dataset of 308 clinical images from three institutions was used to design, train, and evaluate DL-based models. For each image, ground-truth annotation was performed by three experts and combined via union of the labelled areas, thus minimizing false negatives. The dataset was split into two subsets, with 246 training and 62 test images, with 10-fold cross-validation being applied to the first subset. The experimental results were evaluated using mean pixel-wise Intersection Over Union (IoU). Preliminary Results The best performing model was a U-Net architecture with a 224 × 224 input. The downstack section of the U-Net was a VGG16 CNN pre-trained with the ImageNet dataset, fine-tuned with the training subset. The training used random horizontal and vertical flips as data augmentation. A mean IoU of 0.675 (±0.030 std) and mean accuracy of 0.865 (±0.020 std) were obtained. Conclusion These preliminary results demonstrate the feasibility of DL-powered models for automatic segmentation of premalignant and incipient malignant lesions. The induced model can be a reliable, fast, and non-invasive screening tool for cancer detection. To explore the usage of Deep Learning (DL) induced models for automatic segmentation of premalignant and incipient malignant lesions on photographic images. A dataset of 308 clinical images from three institutions was used to design, train, and evaluate DL-based models. For each image, ground-truth annotation was performed by three experts and combined via union of the labelled areas, thus minimizing false negatives. The dataset was split into two subsets, with 246 training and 62 test images, with 10-fold cross-validation being applied to the first subset. The experimental results were evaluated using mean pixel-wise Intersection Over Union (IoU). The best performing model was a U-Net architecture with a 224 × 224 input. The downstack section of the U-Net was a VGG16 CNN pre-trained with the ImageNet dataset, fine-tuned with the training subset. The training used random horizontal and vertical flips as data augmentation. A mean IoU of 0.675 (±0.030 std) and mean accuracy of 0.865 (±0.020 std) were obtained. These preliminary results demonstrate the feasibility of DL-powered models for automatic segmentation of premalignant and incipient malignant lesions. The induced model can be a reliable, fast, and non-invasive screening tool for cancer detection.
更多查看译文
关键词
oral premalignant,clinical photographs,deep learning,automatic segmentation
AI 理解论文
溯源树
样例
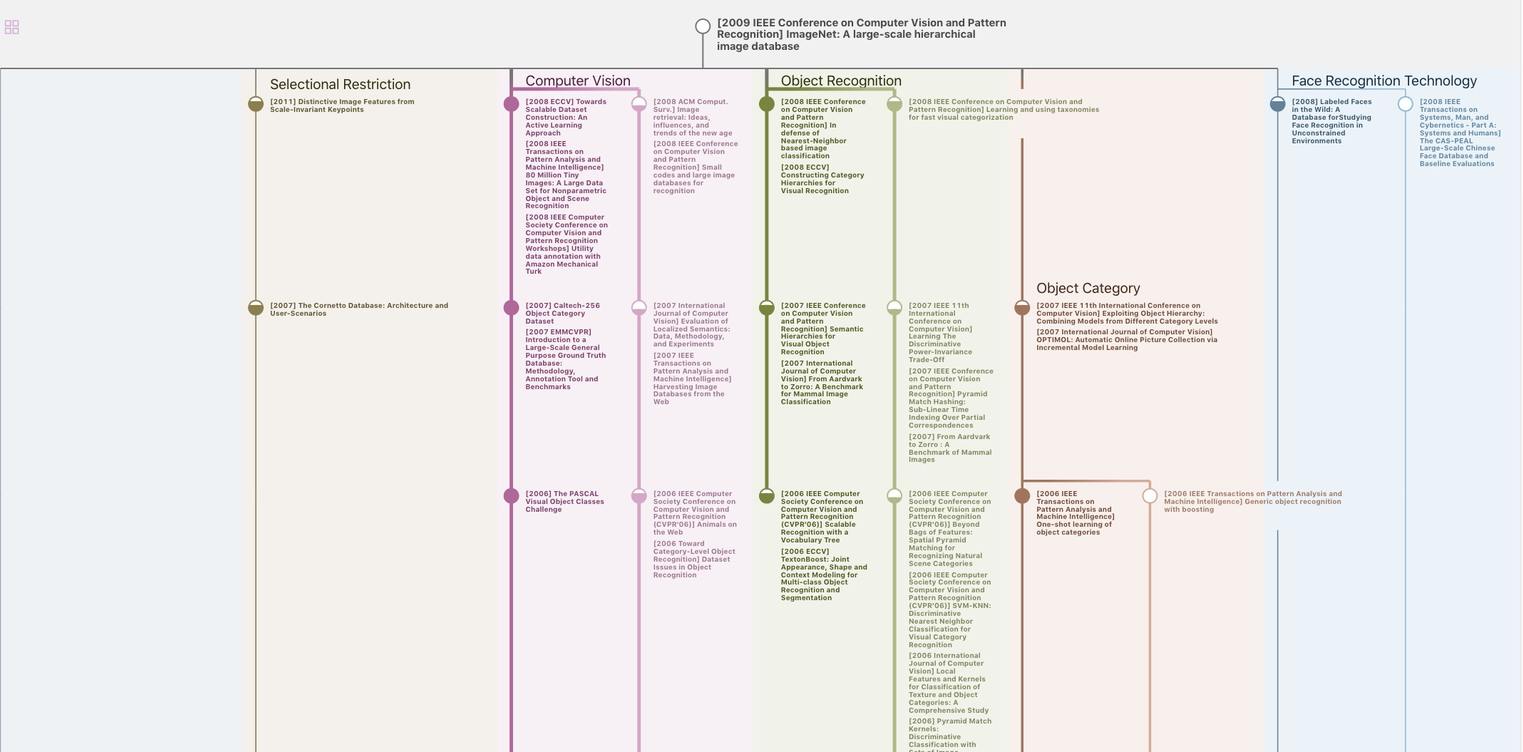
生成溯源树,研究论文发展脉络
Chat Paper
正在生成论文摘要