Laser-induced breakdown spectroscopy combined with principal component analysis-based support vector machine for rapid classification of coal from different mining areas
Optik(2023)
摘要
Coal is one of our most important energy sources. Because of differences in coal-forming year and coal seam quality, the elemental compositions of coal vary among different mining areas, directly affecting coal combustion efficiency and pollution emission. To improve the accuracy of coal classification, laser-induced breakdown spectroscopy (LIBS) combined with a support vector machine (SVM) algorithm model based on principal component analysis (PCA) is proposed to identify and classify coal from 10 different regions. The samples were first penetrated using LIBS, and spectral data were collected. The spectral data were then normalized to improve their signal-to-noise ratio. Finally, the PCA-SVM model is established, the model parameters are evaluated using the grid search method, and the spectral feature classification performance is determined via cross-validation. The results show that the classification accuracy of the PCA-SVM model can reach 98.52%. To verify the performance of this classification model, it was compared with those of five other common classification models and proved that PCA-SVM has great potential for coal quality identification and classification, thus providing a new data analysis and processing solution for coal quality identification and classification in industrial applications.
更多查看译文
关键词
Coal classification,Laser-induced breakdown spectroscopy,Principal component analysis,Support vector machine
AI 理解论文
溯源树
样例
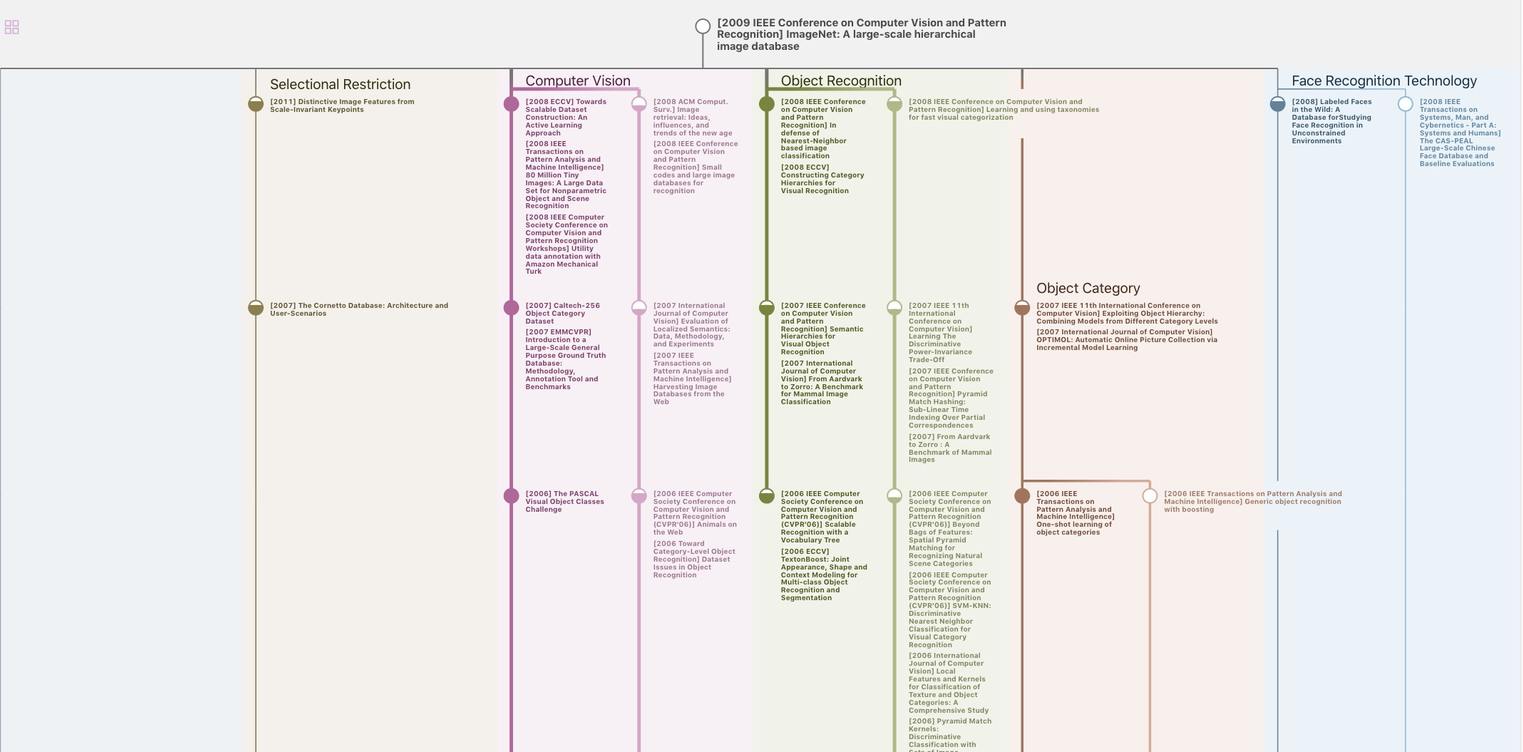
生成溯源树,研究论文发展脉络
Chat Paper
正在生成论文摘要